5 Pretraining on Unlabeled Data
This chapter covers
- Computing the training and validation set losses to assess the quality of LLM-generated text during training
- Implementing a training function and pretraining the LLM
- Saving and loading model weights to continue training an LLM
- Loading pretrained weights from OpenAI
In the previous chapters, we implemented the data sampling, attention mechanism and coded the LLM architecture. The core focus of this chapter is to implement a training function and pretrain the LLM, as illustrated in Figure 5.1.
Figure 5.1 A mental model of the three main stages of coding an LLM, pretraining the LLM on a general text dataset and finetuning it on a labeled dataset. This chapter focuses on pretraining the LLM, which includes implementing the training code, evaluating the performance, and saving and loading model weights.
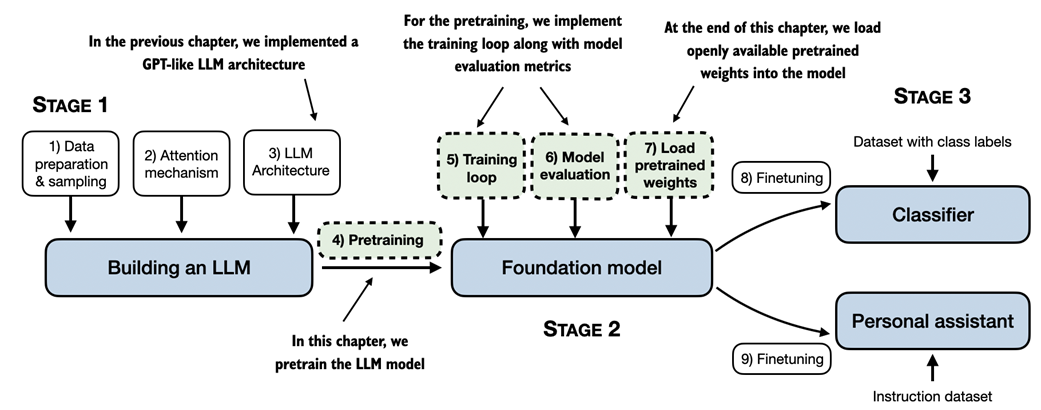
As illustrated in Figure 5.1, we will also learn about basic model evaluation techniques to measure the quality of the generated text, which is a requirement for optimizing the LLM during the training process. Moreover, we will discuss how to load pretrained weights, giving our LLM a solid starting point for finetuning in the upcoming chapters.