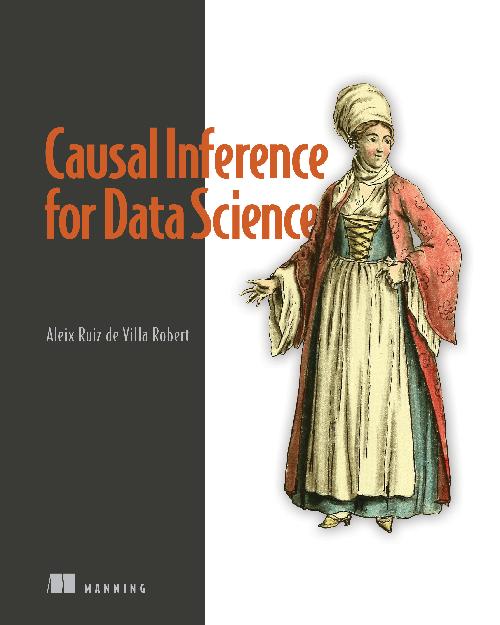
Welcome
Thanks for purchasing the MEAP edition of "Causal Inference for Data Science". This book is for data scientists, but also for machine learning practitioners/engineers/researchers that may feel the need to include causality in their models. It is also for statisticians and econometricians that want to develop their knowledge on causal inference through machine learning and modeling causality using graphs. Readers may need a basic knowledge of probability (basic distributions, conditional probabilities, …), statistics (confidence intervals, linear models), machine learning (cross validation and some nonlinear models) and some experience programming.
I remember discovering causal inference in 2016 through the works of Judea Pearl, and the feelings I had at that moment: a combination of high curiosity and not understanding anything at all,at the same time. As I kept reading, I realized that it solves very fundamental questions around decision making and predictive modeling. After some time, I started to think differently about many problems I had been working on. I ended up enjoying a lot everything related to causal inference and deciding to try to make a living out of it. Moreover,I felt very comfortable with its intrinsic objective: finding the “why”.