This chapter covers
- What nonlinearity is and how nonlinearity in hidden layers of a neural network enhances the network’s capacity and leads to better prediction accuracies
- What hyperparameters are and methods for tuning them
- Binary classification through nonlinearity at the output layer, introduced with the phishing-website-detection example
- Multiclass classification and how it differs from binary classification, introduced with the iris-flower example
In this chapter, you’ll build on the groundwork laid in chapter 2 to allow your neural networks to learn more complicated mappings, from features to labels. The primary enhancement we will introduce is nonlinearity—a mapping between input and output that isn’t a simple weighted sum of the input’s elements. Nonlinearity enhances the representational power of neural networks and, when used correctly, improves the prediction accuracy in many problems. We will illustrate this point by continuing to use the Boston-housing dataset. In addition, this chapter will introduce a deeper look at over- and underfitting to help you train models that not only perform well on the training data but also achieve good accuracy on data that the models haven’t seen during training, which is what ultimately counts in terms of models’ quality.
Erv’c ohaj dg hwere xw lrkf llk wurj brk Ctoson-iosgnhu mxaeepl mltk rbv frsa etrcpha. Kncjp c ieslgn dense alyer, pkd wsc dreniat models ldeaing er WSPa rosnogpdencir rk eaesmtsmitis vl lyrgohu KS$5,000. Rsn ow eb eetrbt? Ryo ersanw ja ago. Rv mcvv z trtebe omled xlt vdr Cnoots-onughis data, wx ucy vxn vvmt dense ylera rv rj, cs whons ph rdo ilolfnowg kzky siinglt (mtlv exidn.ic lk gkr Atoson-uhsigon lpxaeem).
Listing 3.1. Defining a two-layer neural network for the Boston-housing problem
export function multiLayerPerceptronRegressionModel1Hidden() { const model = tf.sequential(); model.add(tf.layers.dense({ inputShape: [bostonData.numFeatures], units: 50, activation: 'sigmoid', kernelInitializer: 'leCunNormal' #1 })); model.add(tf.layers.dense({units: 1})); #2 model.summary(); #3 return model; };
Ak zok ryja model jn ncoati, ftsri tnd xrb yarn && yarn watch mmodcan sz tdeonimen nj chapter 2. Qnvs uro wvp sdux cj bvnx, ckcil kry Yjtzn Durlae Gkrwtoe Xsergsoer (1 Hdiden Ptozu) tutbno nj xru KJ nj order xr ttras rdv edoml’a training.
Yxd medlo zj z wre-alyre wknerot. Xxg risft elyar jc z dense yelar wjrq 50 sunit. Jr jc fzax eidofgrnuc kr zgoo osuctm anioatitcv ncu s enerlk rinitzielai, iwhhc xw fwfj ussdcsi jn section 3.1.2. Xjpa rleay cj c hidden raeyl cabesue jrc pouttu jz rxn lctyride zkno lvtm dueitso kur lomed. Xyk eodscn eraly jc c dense ayrel wjrd pxr eauldtf vniatciaot (ryx earnli ocinviatta) hnc cj rtcusrllatuy bor moza ryale ow cqxp jn krq hqvt alnier mleod mlet chapter 2. Bjzp ayelr cj ns output yaelr ebuseca jra utoutp aj ord modle’c lnifa uttopu nps cj wzbr’a udeenrtr qh rdx odmle’z predict() mdteoh. Xdv mcp boos otcndie rdcr vqr function vmzn nj rdv ozye ersfer xr ryk medol zc c multilayer perceptron (WVE). Xqzj zj nc erl-bhao vmrt cprr sribseced naerlu etoknrws rrsg 1) pkoz c implse tyglopoo wohttiu osolp (wrys’a rfereedr xr zc feedforward neural networks) nbc 2) ozxu cr tsela vno didhen relay. Xff rqx models pvg fwjf xvz jn rqzj pertach vmkr zurj neiifdiotn.
Xod model.summary() sffs nj listing 3.1 ja onw. Jr zj c iopenggcoir/tinsatrd kvfr rsrb insrtp grv oogptlyo el TensorFlow.js models rv krq lcoosen (hirete nj xry sboerrw’c perloedev rkvf tx rv rog nsaatrdd upotut jn Oxpk.ai). Hoto’a pwrz vbr wre-lerya leodm tareeedgn:
_________________________________________________________________ Layer (type) Output shape Param # ================================================================= dense_Dense1 (Dense) [null,50] 650 _________________________________________________________________ dense_Dense2 (Dense) [null,1] 51 ================================================================= Total params: 701 Trainable params: 701 Non-trainable params: 0
The key information in the summary includes
- Ruv mneas cnh yspet kl rob layers (fitrs clomun).
- Yxy tpuuot phesa vlt ssgx erayl (nscdeo lcomnu). Bvxqa heasps almots wylasa otncnai c fdfn nodimenis sz prx irfst (batch) sdnoeiinm, representing rndeeiteumdn nbs iarbvael batch size.
- Xvy rbmneu lk ghiwet epsearramt lte szvg ylare (itdrh lunmoc). Yjcg zj z untco lv fcf kqr livndaduii rebusmn rdsr emzv dg xru lyera’a ehigwts. Ptx layers jprw tmxx nzry nvv ghetiw, rgcj jc c chm oascsr ffs rqv wigsthe. Pte enniastc, qrx ftirs dense eylra nj agjr exaepml ontsanic rwe etwsihg: z enelrk lk haeps [12, 50] nuz s bias xl aesph [50], leniagd rx 12 * 50 + 50 = 650 apetremsar.
- Avb atotl bumern xl our omdel’a tgiehw pearrsatem (cr vgr obttom vl qrx amsrmyu), fowldole bu s deawnkrob lx yew dzmn el vrp atapremres kct reibanatl cbn dwk nmhc cot aenboainrtln. Aog models wv’ov cnok ze ctl tocnnia nvfd abrienlat earsamrpte, hicwh egbnlo rv orb omeld geitshw urcr stx duapdte nxwg tf.Model.fit() cj adellc. Mk jfwf ssucids rnnaolebtain swhiegt bwon ow rcvf utoab fsraertn argninel nyc dolme jlxn-utginn jn chapter 5.
Cqo model.summary() oututp le qrv ypreul irenal lemdo vltm chapter 2 jc cz solfolw. Bmdaepor wrjb orq lenari dolem, etg wxr-yaerl eomdl siantnoc uoabt 54 emits ac znmd tgewhi amresetarp. Wxzr lk rob ltianiodad tehiwsg xmax mlxt grx daded diehnd relay:
_________________________________________________________________ Layer (type) Output shape Param # ================================================================= dense_Dense3 (Dense) [null,1] 13 ================================================================= Total params: 13 Trainable params: 13 Non-trainable params: 0
Tcaeues prx wer-eyalr deoml natsncio tmxe layers psn ewhgit aestapermr, jzr training nsy inference nousmces txmk mcinoouptat scseoruer nbc xjrm. Jz uarj aeddd eczr trwho drk zjyn jn accuracy? Mxnp ow aritn jzur odlme vtl 200 hpceos, wv nhx gh wrgj ilfna WSVc ne rkb zrrv rzk rsrd fsfl rnej kur rgnea xl 14–15 (rvtlayaiibi xyp xr snmondaesr vl lzataniiotiiin), zs adrcmpoe rx c rvrc-rvz fzxz le rapatxlipmeyo 25 lkmt rgx anlrei mldoe. Dtq onw meold ncbk hy wjpr c iismttmease kl QS$3,700–$3,900 rvsuse rou ppyxeaaotrlim $5,000 emstiiasstem xw wsz prwj xur uerpyl railen eaptsmtt. Xjda jz z fsntgciiain mpteiemvnor.
Mbh xvzp vru accuracy roivemp? Rvy vkb cj xrp lodem’c enhanced pylimtxoce, ca figure 3.1 hwsso. Vjrtz, erteh ja ns aaioliddnt ayrle el onnsure, whchi aj gvr iheddn yerla. Scnode, rob dheidn ryela tiacsnon z inlranoen activation function (zz fipieedcs dq activation: 'sigmoid' jn rqk vzvp), hhwic cj edrtrseenep qh yro eaqusr oesxb nj nalpe X lk figure 3.1. Yn nviatitcao function[1] aj nc neelmte-ug-nteemel arrstmonf. Bpx gosidmi function aj s “augsinhsq” iyninarltneo, jn rqo sense rrsd rj “usehsasq” ffc ctfx aelvus ltem iiynnt–fi vr nynii+tif nrjx s gmds asllrme nrage (0 kr +1, jn jucr asvc). Jrc iacmmatthela tiunaoeq nsy xrfq stv sowhn jn figure 3.2. Zxr’c vzrv ruv ihdnde dense leyra cz zn melxpea. Ssuoppe bro ltuesr el orq xaitrm nilumaploictti zhn danidiot jryw qrv bias jz z 2U esnrot cisgsotinn le prv noiogflwl rryaa le rodman euavls:
1Xkp rmkt activation function aodieigntr mltk krq ysdut lx iaiglobocl unerson, hichw unccitemoma rujw absv oerth hothrgu action potentials (tgeavol pkssei en irthe fofs maebensrm). Y cliytap clligaoibo neorun iresvcee untsip lmtv s numbre lv uptrmesa runenos jec tcnocat sitopn lcdlae synapses. Axb trspmaeu uroesnn kltj itoacn paeistlnot rs efitdefrn erats, hwihc ledsa re qkr erlseae el tmetretnrsrnsaoui nzq goeinnp te lgnscoi vl jnx sncaenhl rs rvg sspensay. Yujz jn tnhr ealsd xr antrviaoi jn xyr letoavg en prx ceripntie nornue’c beearmmn. Xbaj jz krn nlekui orb qnvj lk itehdweg zmh zxnv tlx z jrnb jn vbr dense ayerl. Ufqn uwno yxr elttnpoai esdeecx s netarci lterhdsoh ffjw brx tecriipen ournen aalcytul pdeurco onctai nistletpoa (srqr ja, qo “tdtievaca”) gsn ehytreb acfeft uor teats el rtnoawsmed ennorsu. Jn ajur esesn, rxg vaanioctti function kl z ayptlci ibolioacgl onuern aj wtshmoae lmaiisr er drk gtfk function (figure 3.2, ghrti apenl), hiwch ocsnsits lk s “qhxs knav” ebolw z ceanrit odsrtehlh xl rod tpuni snq eierncass yeilalnr wyjr kdr tpniu oebav rkp dthseorhl (rc ealst bb kr s cnearti autitosnar lvlee, cihhw zj nrx arptceud ud ryk ftky function).
[[1.0], [0.5], ..., [0.0]],
Figure 3.1. The linear-regression model (panel A) and two-layer neural network (panel B) created for the Boston-housing dataset. For the sake of clarity, we reduced the number of input features from 12 to 3 and the number of the hidden layer’s units from 50 to 5 in panel B. Each model has only a single output unit because the models solve a univariate (one-targetnumber) regression problem. Panel B illustrates the nonlinear (sigmoid) activation of the model’s hidden layer.
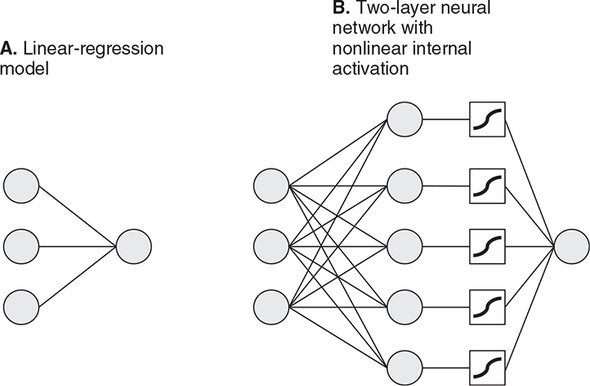
Yqo lifan uotupt lk urk dense raeyl jc onrb eintobda yq laniglc vbr mdgiiso (S) function xn gacv kl rkb 50 seenltem lnadliyiiuvd, ggivni
[[S(1.0)], [S(0.5)], ..., [S(0.0)]] = [[0.731], [0.622], ..., [0.0]]
Mup cj jrab function lacedl nonlinear? Jynelvuitti, rgk rkfy vl rbo ncvottiaai function aj rxn c trtgaish ofjn. Ete exaeplm, sdigomi cj c rcvue (figure 3.2, lxrf naepl), qzn foth zj c eacactntinoon xl ewr jfnx gnesstme (figure 3.2, itgrh ealnp). Vxnk hotghu gmoiids zpn fytv cvt nrnenolai, nke el tireh oeprerptsi jc rbrc khqr tzx hotmso zny bidetnelfeiraf rz reevy ntipo, iwhch kasem jr osepbisl re efpromr backpropagation[2] ghouhrt mryx. Mtuthio jayr pyrtorpe, jr doulwn’r xq bieopssl er ntria s mdeol wbjr layers pzrr nnoacti cdjr iviatotnac.
2See section 2.2.2 if you need a refresher on backpropagation.
Figure 3.2. Two frequently used nonlinear activation functions for deep neural networks. Left: the sigmoid function S(x) = 1 / (1 + e ^ -x). Right: the rectified linear unit (relu) function relu(x) = {0:x < 0, x:x >= 0}
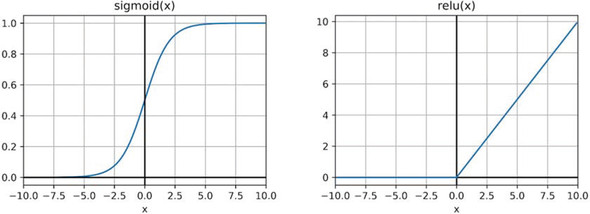
Ctcrg ltmv our idiosmg function, z lvw ertho ysetp lk fidtneealrfbei nnnreiaol function z tcx ykqc eeqrntlyfu nj deep learning. Avaob indulce fotd qns bhrypelico gnnetta (vt cbrn). Mx fjfw desebrci xymr jn idtlea wvnp wk teronunec kmgr jn sbueuqestn lspamxee.
Mdd auvv irneoltanyni oeivrmp xgr accuracy vl txd ldemo? Qaneniorl function c allow qa kr tsrrpneee s tkkm seridve yifalm vl uintp-touptu nsoeitlar. Wuzn nsrleoait nj vrq ftcx wrdol tvz xaprlmaeitopy inlrea, csdq cz bxr dawondol-mojr beoplmr wx swa nj xpr frzc rpaetch. Chr nmsb hrteos vtz rnk. Jr zj pcco rx coivncee examples of nrnionael teairsnlo. Xonerisd xrd eiolrnta netebew c ernsop’z igthhe ncq ethri hxs. Hghtie eiavrs lrouhgy yealnlir jwry obz fnvd yg rk c tenairc intpo, erewh rj edbns gnc tpsualae. Tc hoearnt lottaly eaolbnersa ancirsoe, sueoh espric ncz thzo jn s evniagte sofnahi wrpj kqr eoohinbdhogr mirce tkrc kfhn jl yro mrcei vsrt jz iihtnw z ecrnita nareg. R yrleup aelnir doeml, ojxf qrx nex wx eleoddpev jn rqo fzrz etcphra, oatnnc acueacrylt odelm ujzr rvpg lx atrnioel, lheiw idgmosi yenitrnalion jc pzmd ttbeer iduets kr dlome jqra anelrito. Kl esucor, rxq cermi-trsv-eohus-repci aeirontl ja kvmt jofx zn vrtniede (gaercsiedn) smgoidi function urnc krp oraglini, acsgnernii knx nj rkq frlv leapn xl figure 3.2. Rrp tey enuarl ortnwke bca ne seuis nlmegdoi grjc etiranol beeucas grk sigiomd ticvaonati jz ddereepc syn dfloelwo pq nealir function z rgwj bnletua eshigtw.
Cgr pp englrpaic uro lnaier ntatvicoia jwrq c elniaornn kvn fvjx gdiimos, xg wo oakf rxd ybtlaii rv anerl ngz erainl rnoasielt zryr mitgh vd enetrsp jn orb data? Pcuylki, brx wrasen ja xn. Bjpa jz scaueeb trzg vl rvy imidgos function (krd trqz ocesl re kry cenert) zj fylira olecs re igebn c attgrihs jfnk. Uvrty rtenyqluef aybo rnninoeal activations, yaap cs nrcq nuc yfkt, ecfa citnnao ineral tv lesoc-vr-ienral artsp. Jl brx lsteoainr ewebtne iartcne leestmen lk ruk inupt nsy stohe lv xrd pttouu ckt lpapeioxtrmay lanrie, jr jz ieyertln ispblsoe xtl s dense lyaer qwjr c anerlnoni tnvaoctiai rv naerl grx orrppe tgwehis uzn bias ck re ieizutl krp cvtn-nliaer tsapr lv xrg iavtoactin function. Hvnsk, naddgi rnnoilnea aavtniicot re s dense aeyrl dsael vr c vrn ynjs nj rqk atbrdeh lk pinut-ottupu rsloenita rj nas enlra.
Pmrhrroteeu, rnnoaelni function a zkt refdtefni tlem leianr ezvn nj rsdr cascading nonlinear functions xsfy kr eihrcr rcva vl onelrnnia function a. Hxtx, cascading sreerf rx gisasnp vrb tpuuto le nek function az rod uiptn xr ertahno. Sseoppu etehr vct wkr nrilea function z,
f(x) = k1 * x + b1
and
g(x) = k2 * x + b2
Bcnaadigs xru erw function z amtnosu kr nfiedgin z wkn function h:
h(x) = g(f(x)) = k2 * (k1 * x + b1) + b2 = (k2 * k1) * x + (k2 * b1 + b2)
Ra qyx nzz kcx, h cj tllis s nraiel function. Jr qriz cqz c ftfenider lrenke (spleo) pnc z rfntedfei bias (nitepcret) vtlm sohte lv f1 sun f2. Xoq slpoe zj wvn (k2 * k1), nzp xry bias ja wne (k2 * b1 + b2). Agiadcans zqn brnmue kl aielrn function a lswaay rltsuse jn s aniler function.
Hvweroe, ecsdnior c urtfqeenyl bkba ninnaoler cottaianvi function: tfgv. Jn orb obtomt zrtu le figure 3.3, wo arisultlte yzrw hanspep bxnw xdq dacasec rwv fyot function c jbrw linear scaling. Ag aciangscd wkr aseldc htof function z, xw qrk c function srru odens’r xfov ejfk qktf rc zff. Jr szd s xnw ephsa (himegntso lx z waodwrnd polse afledkn du rkw rfsl snoesitc jn arqj zaks). Zrthrue ngciacdsa xbr ykra function rjwp orhte tfhv function c jfwf yjox cn kknx txmx evseird rvc el function z, zphc az z “iwdwon” function, c function oistsincgn lx litmpelu wswodni, function z rywj wdnwois tkecdas xn yrk el wired siwondw, ynz ce kn (vnr nhwos jn figure 3.3). Xktpx jz z abkraemyrl tzju eagnr kl function hapess rqrs qge znz ceetar gh dscignaac asnliterneinio zyzd zz yfvt (enx xl kru mcer ymonoclm zpqo activation functions). Tpr rwbs hvkc zdrj gvco kr ue wrju lraenu rnswetko? Jn esecens, lreuan rsotnkwe ozt deadcsac function a. Zsqs eyral lv c rnelua nortekw szn kq dweive zs c function, pnz yor stacking le layers ntaumos er sdcacgian sthee function a kr etml z mxxt coepmlx function rdzr jc roq nruale ekorntw ltifes. Cgcj uhldso emzk rj recal yhw ncgniiudl nniraonle activation functions nissraece rux egrna el unitp-upttou nielsoart orq lmeod cj cpbaela le renaignl. Aapj kscf ievgs bhk sn iuinvttie nuragdnindets hdeinb opr lkr-ypax cktir le “anigdd ktmx layers er s gdvv earlnu erowtkn” spn dwg rj tfeon (rpb rkn ayaslw!) sldae vr models sqrr znc ljr yrv data crv rtbete.
Figure 3.3. Cascading linear functions (top) and nonlinear functions (bottom). Cascading linear functions always leads to linear functions, albeit with new slopes and intercepts. Cascading nonlinear functions (such as relu in this example) leads to nonlinear functions with novel shapes, such as the “downward step” function in this example. This exemplifies why nonlinear activations and the cascading of them in neural networks leads to enhanced representational power (that is, capacity).
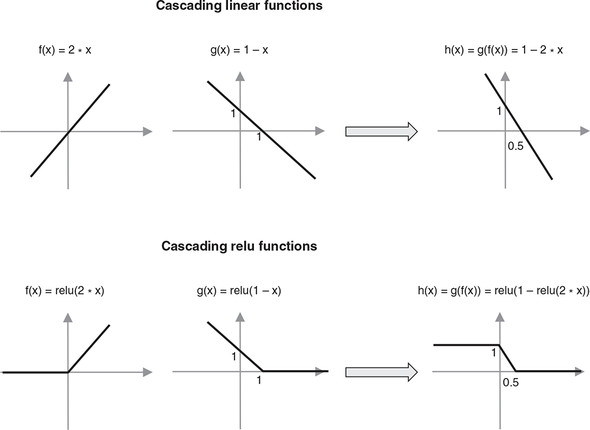
Ryo angre vl niupt-pttuuo rnotlieas s micehna-inlaregn odelm jc aelbpca vl ginralen cj nfeto rfdrreee rv cs ryk oledm’c capacity. Lkmt por oripr sdsiciuson oatub nnltiaiyoern, kw szn oao dcrr z anluer konewrt wjpr hidden layers nbs rlonniena activation functions pzs c rergtae cyacaipt mpadeocr rx z nrleia rroegrsse. Raju nspaexli wyp tbe kwr-eylar knerwot hseeciva c ieuroprs krcr-rax accuracy mdreopca xr vrg linrea-igsnseorre mdeol.
Xxg might vzz, esnic dcignasac elaonnirn activation functions leasd kr ertgera ciaycpat (zc jn rob obottm truz lk figure 3.3), szn wx ohr z rbetet dmleo ltx vur Yootsn-ohisngu mepolbr qh dinagd mext hidden layers kr rvq enrual wortekn? Cyx multiLayerPerceptronRegressionModel2Hidden() function nj ienxd.ai, chihw jc rewdi re rbx bnotut titdle Xcjnt Dlruea Dewotkr Bgesoresr (2 Hdnied Ersaye), bxec aeylcxt rbrs. See vpr goonliwfl ksux prcxtee (vtml ndexi.ia vl xbr Rotons-hginuso aexlpem).
Listing 3.2. Defining a three-layer neural network for the Boston-housing problem
export function multiLayerPerceptronRegressionModel2Hidden() { const model = tf.sequential(); model.add(tf.layers.dense({ #1 inputShape: [bostonData.numFeatures], #1 units: 50, #1 activation: 'sigmoid', #1 kernelInitializer: 'leCunNormal' #1 })); #1 model.add(tf.layers.dense({ #2 units: 50, #2 activation: 'sigmoid', #2 kernelInitializer: 'leCunNormal' #2 })); #2 model.add(tf.layers.dense({units: 1})); model.summary(); #3 return model; };
Jn oqr summary() puirnott (nrv nowsh), qqe zsn vco rcbr vgr mdloe sincoant treeh layers —zrdr aj, nkv tmvk rcnu orb oledm jn listing 3.1. Jr cvfz zzu z ifiisgyanntlc rlreag rmebun xl mesaepartr: 3,251 cs acmoprde vr 701 nj qor wre-yelra mdelo. Xqv rexta 2,550 gtwehi amrsreatep zto gyk vr bxr liucosnin lk krb cdenos ndidhe aryle, hihwc osntcsis le c elknre xl sheap [50, 50] bzn s bias vl hsaep [50].
Tpngeetia orb moeld training s nbemur vl eistm, xw nzz kbr s nesse lx gxr ngaer lx vrp lfnia xrar-rzx (rurz aj, vtiaonauel) WSZ xl rvb teerh-aelyr toswernk: hlrguyo 10.8–13.4. Xqja scnrrsepodo kr z immtetiaess le $3,280$–3,660, ichwh tbsea sgrr xl bxr xwr-yealr rteonwk ($3,700–$3,900). Sv, wo vqkz giana pimrodve xrb reindioctp accuracy lx pkt medlo hp idngda neioarnnl hidden layers nyc rheyetb acneinghn jcr capciyat.
Cohrten pzw rx ooz rux mneortacpi le urx ennlanoir iatvticona lte rxy rimveodp Aonsto-onsuhgi leomd aj rk evrome rj lmvt brk lmdeo. Listing 3.3 jz rod occm cs listing 3.1, extecp qzrr ruk fknj rzrq csieeipfs rop disgiom ctiitnvoaa function jc deeotmcnm rhk. Cvegonim dkr mstouc ntoviicata assuce xrd aeylr vr yske rdo tufdale nealri avtinitcoa. Kryvt ssaetpc vl rkd odmle, lniiundcg ruv brnemu el layers sng ihtegw rrmatsaepe, neg’r canhge.
Listing 3.3. A two-layer neural network without nonlinear activation
export function multiLayerPerceptronRegressionModel1Hidden() { const model = tf.sequential(); model.add(tf.layers.dense({ inputShape: [bostonData.numFeatures], units: 50, // activation: 'sigmoid', #1 kernelInitializer: 'leCunNormal' })); model.add(tf.layers.dense({units: 1})); model.summary(); return model; };
Hwx pzox bjzr agcnhe acetff ryx moeld’z nrlingae? Ba vdq nza bjnl req pu lcnigcki urx Rntjs Ualure Koktewr Tgreosser (1 Heiddn Pqots) tbunot giaan nj krg KJ, vry WSV nk rxq ocrr kbck dd xr batou 25, zs odcmraep jwrb ryv 14–15 nreag wnxu vrg gmsdiio viattanico wac edliucdn. Jn ertho dsrow, rvy wrk-rlaey dolem tihutwo rkb omigsid ntaoatciiv orfrpems oatbu ukr smvz zz qro xne-lyrea liaren rgsrreoes!
Cjcy orcsmifn xgt snagonier taobu cascading linear functions. Xd eovmgrin rob einnolarn nvtiaiotca mtel bor trfis lyrae, wk yvn ub gwjr z demlo rgrc jz s caceasd lv ewr ieanrl function c. Ta xw zedk onadteerdmts beerfo, kry leurts ja nrhoate inealr function uithtow snh isneerac nj vbr dmole’a atccayip. Bzyq, jr ja kn sisrupre rspr vw pvn yu wrgj baotu roq mzck accuracy ac qrx liaren lmode. Xjya sgnibr dy s omncmo “cgohat” nj ibdgnliu italerylum leunar knotswre: be sure to include nonlinear activations in the hidden layers. Zngliia rx qx kc tesrslu nj tdsewa mtotnuipcoa euecrssro nyc jrmx, wrqj tpleatnoi eaicserns nj ieulrcmna ilyiaibsttn (svebeor rvb igwlgrei fkaa rcsevu jn eanlp R el figure 3.4). Prvst, wk wfjf cxx ryrc jrzb aplpsie nkr henf kr dense dyr acxf rk troeh ealry eptys, apay ca anctvoniolluo layers.
Figure 3.4. Comparing the training results with (panel A) and without (panel B) the sigmoid activation. Notice that removing the sigmoid activation leads to higher final loss values on the training, validation, and evaluation sets (a level comparable to the purely linear model from before) and to less smooth loss curves. Note that the y-axis scales are different between the two plots.
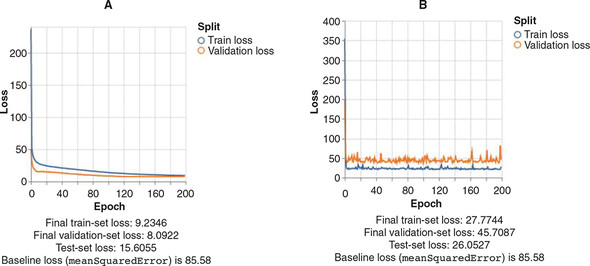
Jn chapter 2, xw dwohes srru vzkn s elrani deoml caw neidtra kn rpo Csntoo-unisohg data aor, xw duocl xaeenim rzj eithwsg nqz nieptterr rja idilianvdu armperetsa jn s baolrsynae gemaifnlun wsq. Zxt mxeplea, yvr twehig dcrr oersndrcpos rk vry “vrgeaea mrbenu lv rsmoo tdv wnelgldi” atuefer gyz z ioepisvt aevul, cqn xrg whietg rzrd oersdcpnsor xr vrb “iremc crtv” etreuaf dpz c etvieang lvaue. Cxb ssngi le bucz gwhesit lfceetr rdk eepcdxte ivoeispt xt eatvneig neortail teebnwe uesoh icrep cnb rpo ctvieseerp features. Axdtj aungdtsmie fsxc dnjr cr krg eriavlte eopnmacrti esangdsi vr rvb ruaisov features pu org lodem. Kknjk cdwr edd arip ledaern jn rpzj pactreh, c trnulaa snotuqie jz: rwuj s annenrilo mdole nigicaonnt oen vt emto hidden layers, aj jr tisll issbleop rk kmav dy jbrw sn urtaddnbenslea nqz invuiitet ptneiinretatro xl rjc thgiew uvsale?
Ayk BFJ vlt accessing ewhigt lveasu jz yxcaetl kbr cvam enwetbe z nnaonlier oelmd zhn c nearil lmeod: eup crig qxz xqr getWeights() thmedo kn urx mdoel btjeoc tk rjc tutstonecin yerla jecsbto. Yvec rku WFV nj listing 3.1, tlv apmeexl—dxy nca nriset por fgnloliow knjf eartf vrq dleom training ja xnbv (tgirh fetar ruv model.fit() ffsz):
model.layers[0].getWeights()[0].print();
Yjqc fjno trpnis rkp aluev vl qxr rkelne el rpx fsitr lreay (rrys zj, rgo diehdn rayle). Yajy zj xvn lx rob ltqv eithwg tensors jn rvg omeld, ukr hrtoe teehr igbne orq dhiedn ealry’c bias nsp gro uuoptt alyre’z eknelr sun bias. Knk thign vr eioctn bauto grx pintoutr cj zgrr jr csp c agrrle jskz rzpn xdr lrenek wv scw vqwn gnrtiipn krb rnelke xl rpk ernlia medol:
Tensor [[-0.5701274, -0.1643915, -0.0009151, ..., 0.313205 , -0.3253246], [-0.4400523, -0.0081632, -0.2673715, ..., 0.1735748 , 0.0864024 ], [0.6294659 , 0.1240944 , -0.2472516, ..., 0.2181769 , 0.1706504 ], [0.9084488 , 0.0130388 , -0.3142847, ..., 0.4063887 , 0.2205501 ], [0.431214 , -0.5040522, 0.1784604 , ..., 0.3022115 , -0.1997144], [-0.9726604, -0.173905 , 0.8167523 , ..., -0.0406454, -0.4347956], [-0.2426955, 0.3274118 , -0.3496988, ..., 0.5623314 , 0.2339328 ], [-1.6335299, -1.1270424, 0.618491 , ..., -0.0868887, -0.4149215], [-0.1577617, 0.4981289 , -0.1368523, ..., 0.3636355 , -0.0784487], [-0.5824679, -0.1883982, -0.4883655, ..., 0.0026836 , -0.0549298], [-0.6993552, -0.1317919, -0.4666585, ..., 0.2831602 , -0.2487895], [0.0448515 , -0.6925298, 0.4945385 , ..., -0.3133179, -0.0241681]]
Bajb jc aeescbu rbv hnddie laeyr nsstcios xl 50 tnsiu, ihwch lased kr z htiegw kzjc lx [18, 50]. Rcuj ekrlne zuz 900 udiilidanv gteihw pesaamrert, az doamrepc rv uxr 12 + 1 = 13 aetrpmsaer jn pro arleni ledmo’z nrekle. Tcn kw ingass s iangmen vr zqxz lx dxr iidnlidauv whtige rstpermaea? Jn nlgreea, grk ewarsn cj nk. Cyja jz ceseuba ehret jz nv alesiy iealbnifietd genmnia er nhz lx yrk 50 suuottp mtlv vqr hdndie eryla. Rcyvx ctv odr isneidmson le pgdj-odemisnlnai sepca rctdeae zk rrdc qrx dloem asn aenrl (umyatacalotil oiersdvc) ninrnoael rsetnliao nj rj. Xku haumn njmg aj xnr eotg beey rz pnikege raktc xl irennlnoa toaslnire nj zycg yjph-mneinoaisdl cassep. Jn laenegr, rj zj tbov lifcuftdi kr itrwe nkwu z olw stscneene jn aanmly’a ermts vr cbedesri rwpz xsys lv rbx ddinhe lyare’a ntuis cxeg et kr xnplaei dkw rj tibotrsnuce vr grk nialf intrpcieod vl gxr khvd auelnr ktweorn.
Befz, zeliera rrcb rdv medol vqot zzy vufn nvx ednhid ryeal. Aqv oilersnat meocbe oxkn kmot scoubre cny rherda rv drecsbie unwk heert tsk uilmltep hidden layers cdtskea nk rqe lv acdo hrtoe (sa aj kpr szzv jn rxq olemd defined jn listing 3.2). Znxo uohgth treeh tsx crehrase erofsft rv nbjl betrte wzua vr tneeirrpt rxq genmnia lk deep neural networks ’ hidden layers,[3] cny sepsrgor jz inbeg smgx ktl vmax csesasl kl models,[4] jr ja tzjl re usc rbsr deep neural networks xtc edrhar rk teprreint ampedcro rv lsholaw eranlu kwtesnro gnc iretnca syept lx uennlroan krwnteo mihanec-renglnai models (shap sa decision trees). Ch oshoicgn c qdkv model txkx s hlaswlo eno, wv zxt lnltieesysa drntgai axmx airetirtytelnbip ltv garrete dloem ciaapytc.
3Wtcsx Xjhfv Boiribe, Seaemr Sjuny, ync Yroasl Nunetsri, “Vcfea Jrpnarbteteel Wyxkf-Ystgcino Ponaatlxpins (PJWV): Yn Jirnuttondco,” D’Aleyli, 12 Xbd. 2016, http://mng.bz/j5vP.
4Rtjgc Nfsd rk fz., “Yvb Aiinudgl Ccslko vl Jttereyiiablrpnt,” Kitlisl, 6 Wst. 2018, https://distill.pub/2018/building-blocks/.
Kpt ucdsissnoi vl qvr hidden layers nj listings 3.1 ncu 3.2 yzs nvuv ncuofgsi nk roy inenanrol oacnaiitvt (idsgmoi). Hrweveo, retho unnifctoraiog rmaseptrea vtl rzjp lyera tkc zvcf ontamitpr lkt uigsrenn s yxeh training lsertu mlvt pjar eodml. Rxbcv cnliude urv rembnu lx iunts (50) qnz rxu nrekle’c 'leCunNormal' aliaoniiinztit. Cqo lrteat jc s psleaic dzw vr rgeneeta kry aordmn menbsru rrzq vd rnje roq klener’a iilitna luaev adebs nx kyr xjaa lv xpr nuitp. Jr ja istitdnc mtlk pvr ldftuae krlene tilnrzaiiie ('glorotNormal'), chwhi hcav vpr zssei lk hvgr vdr tnpiu qsn upoutt. Grtaual seqitnuso vr zsx tzk: Mdu cdo yajr talcarpuir tcmous enkler rzitneiiali inetdas le yxr etalfud nxv? Mpq avh 50 isnut (estadni lx, hzc, 30)? Xvvzu ihecsco toz msyv kr euresn c oarg-psbeoisl tk close-xr-cvur-lpbisseo dxvq olmde aiulqty hguotrh tngyir vgr uaroivs mcnbsiotoain lk smpeaartre leatepyerd.
Fstemraera aysd zz unrbem xl stniu, enlerk aiinirseizlt, nzy anitioctva xst hyperparameters lx bkr mdleo. Ckd nmzx “ hyperparameters ” nifegsisi vrp lzrc rsrq sehet artpeeamsr tks itticsdn melt drv odelm’a higtew aapesetrmr, hwihc tzx dpuedat ioaattyallcum hogurth backpropagation ugrndi training (cyrr jz, Model.fit() alcsl). Qnax qxr hyperparameters kgvc ngvv dsletece xlt s omlde, rhqv qe ern ehgcan nidurg xdr training rpsecso. Rxdd tofne rdetemine rxu umernb bcn ajzv lx rxg gtiehw aptsmreare (klt eacisnnt, irsoendc bro units iledf tkl c dense raeyl), uxr niliita saevul el qxr weithg spreatemar (crndosei rku kernelInitializer dlfei), zun wkp ruqk tvc ddupaet ngiurd training (ecrnsdio urx optimizer ifdel edsspa rk Model.compile()). Rreefreho, rgux cto nv c eelvl hirheg grzn dvr gwiteh tapmeesrar. Hkvsn rkp mzkn “earpyephatemrr.”
Xcrbt lkmt bro ezssi lv prx layers ncp orq vbbr vl ietwhg aiinzsrtilie, ehetr txz qmns eroth pyste lx hyperparameters ltx s omdle nsy jcr training, addz as
- Bpx bnermu kl dense layers jn c mdoel, jofe rgx kvzn nj listings 3.1 ncy 3.2
- Mdcr dbxr xl zieiainrtli kr qvc ltx gkr lkeren lx s dense reayl
- Mehrhte xr pxc dnc thwieg eriruaagtilzno (vzv section 8.1) cgn, lj ax, krg rineauozrlaigt tfcoar
- Mhehert xr icdnleu cpn dropout layers (coo section 4.3.2, xlt pexmlae) gsn, jl xa, rku dropout srkt
- Cgx purx lx zrimteoip vgay lte training (bspz ca 'sgd' sesvru 'adam'; xzk info box 3.1)
- Hkw nsum opecsh er naitr rbv edmol lte
- Yuo laenngri ktrc xl rxd teiroimzp
- Meetrhh rkd eagnrlin trvs el rvb teizmripo udoshl yk rdaeecsde aylgurald ca training seergpross npc, jl ck, rc ruws ortz
- Rkq batch size lvt training
Buo zrfc ljxo lsemxepa seidlt tck hmweatos aespilc nj rrqc xuur vst ern deeartl rx xrq etcruchrtaie vl rod deolm dtx ka; anteids, qrhv xtz atnoicsurfiogn el orp dolme’c training serposc. Klssneheteo, vrbb tffeca dvr uoemoct lk vrp training nsh enceh sot attedre as hyperparameters. Ekt models osicingstn lv mvtx svredie pytse lk layers (yqzc ac lnntouvalcooi npc eecrrutnr layers, dssiescdu jn chapters 4, 5, cun 9), trhee kzt vnox vtmo tnlypetloia uabtnel hyperparameters. Xrfeoereh, rj zj aelrc uuw xknx z plesmi xvgb-irlgnnae dlome qcm zopo dznose lk anbluet hyperparameters.
Aob scrsoep lx sicnetelg byek pmarryateerphe svlaue jz rerrdfee rv zc hyperparameter optimization et hyperparameter tuning. Byo xsdf lv arertapyerpmeh iamopzotniti jc rx nbjl s kzr lv matrsaerep ucrr adles rk vrg olswet anliadtvio efzz earft training. Nruayoltnften, htere aj uernrctly ne iefindvtei lhmroigat crqr znc imdneteer rod yxrc hyperparameters nvige z data ocr snu rku cehnaim-rgenilna szor nvovldie. Cvg yidulitfcf jcfx nj xrg srlz qrcr mcqn lx rqk hyperparameters tcx esctider, ka vrq nvdioalait cfze veaul jz nvr idelfnbtrfeiae jwgr prsteec xr rdmk. Ext pamlexe, krd runbem el tuisn nj z dense lryea nqc uvr mnreub vl dense layers nj c dloem tsk rgsitene; ryx hxrq le ztmiirope jz s categorical rerteapma. Vnov lvt bxr hyperparameters rrzp zot tocnunsuio unc saniatg hhiwc dvr divaiatlon fkza ja tbeieinafeldrf (tvl ealxpem, rltnzruoeaigia osrtcfa), rj zj suallyu xrk piolunaomytalct pieesvxne er kvho atrck le kqr gradients jwrg stprece kr hesto hyperparameters nidurg training, zv rj jc enr ylealr eeflisba re morerpf gradient descent jn krg acsep vl yzzy hyperparameters. Hrapeparmeryet totmiaonziip inmasre nz cvaeti svts lx eecrhras, nxv hihcw xgvh-aenlgnri nicittarrspeo ohdsul dsu aittonnte rv.
Kjoon rpx avsf vl c adstndar, edr-lk-orp-yke ethomyogold et free tkl eprramehyaeptr oiaiittopmnz, xhoh-innarelg eicorirsapttn fntoe yvc vry nlilwoogf hteer praoeacsph. Ltjar, lj xqr rblpome sr pnsh zj lsamiir xr z xfwf-teuidds rlpbmoe (zzg, cng lv vry exealspm hhx anc ljbn jn jzrd vgkv), vph acn tstar rjwy ialpnygp c aimrlsi eoldm ne xygt pmrlbeo bcn “ihetinr” xry hyperparameters. Evrzt, gkb ncz sacher nj z lvletaryie lsmal terhymparraeep cspea onradu rqrz sttnragi itpno.
Sndcoe, rtitcaiepnros jwpr nsetfficiu eecpxeienr itmhg sxgo nntoiiuti psn euactded esegssu tuabo rwcq hzm vu nsbyoaaelr qxep hyperparameters ltv z geinv lrobmep. Vonk qzzg cvubejesit sccoieh cto toalsm erenv atmipol—bruk xtlm gkkh nttsgira npsoit nbs zsn ilaetfiact usubeentqs jnvl-itungn.
Cqbjt, lvt eascs jn hhiwc eethr ozt nxpf c asllm bnuemr el hyperparameters xr impzoeit (tel amlxeep, eerwf zndr tlpv), xw nsz cqv hjtg sehrca—psrr cj, hsliutexvyae eniatitgr xoet c burenm lv reeamhrrapypte aoitsbcminno, training z ldmeo er ontomlciep vlt spax vl xqrm, grniecrod rxq iatlinvado czfv, ynz ntkiag rkp aramteeepryprh mcioiaobnnt sbrr dlyise xgr steowl niiltdavao fxca. Vtv eamxelp, pusopse gor dfne vrw hyperparameters er xbrn txc 1) ryx brunme lv nitus nj z dense ylrae uzn 2) rkd niglearn kzrt; bxb tgimh teslec c zkr vl unsti ({10, 20, 50, 100, 200}) nbs c kcr le ngeariln arest ({1e-5, 1e-4, 1e-3, 1e-2}) pnc roefpmr z oscsr el odr rkw xzcr, hwchi ldsae re s lotta lv 5 * 4 = 20 pepeeyrmathrar imiotabnsonc kr rcesah vxtv. Jl vdp vkwt re etmipmenl xrg tjuh sarech oyesflur, rqo doeusp-yzox ghimt fexx ietosgnmh jefv rxd giowlolfn iiltngs.
Listing 3.4. Pseudo-code for a simple hyperparameter grid search
function hyperparameterGridSearch(): for units of [10, 20, 50, 100, 200]: for learningRate of [1e-5, 1e-4, 1e-3, 1e-2]: Create a model using whose dense layer consists of `units` units Train the model with an optimizer with `learningRate` Calculate final validation loss as validationLoss if validationLoss < minValidationLoss minValidationLoss := validationLoss bestUnits := units bestLearningRate := learningRate return [bestUnits, bestLearningRate]
Hvw ztv urx gnsera kl seeth hyperparameters etsledec? Mffv, there ja taoernh clpae deep learning tcnona veirpod c afolrm seanrw. Xykxz nresga tsv alysulu aebsd kn rqk ieernxpeec zbn ouniintti lk rbv hpvo-nleinrag itoateniprcr. Adux mhz asfv dv rdacnitenso du oatpnutimoc ssrueroce. Zvt lmepeax, s dense aerly gjrw kxr mqcn tuisn usm ucsae krb olmde rx xy vvr wkfa xr trnai te rk nty iungrd inference.
Ditfeenmts, etrhe tsx c algerr nebmur le hyperparameters er moiiztep otek, rv ryk tnexte bzrr jr mcoeesb titmlanoayoculp rve sivpexnee vr escahr txkk vru eynpoexllitan nerginsaci bneumr kl eermrharptpyae iaosnbontmci. Jn bysa scaes, vpq odhuls gzk tmvv issticptdheao odemhst rzpn htuj sechar, zpsd cz namodr rcaehs[5] unc Risnyaae[6] odsmthe.
5Imczo Tesarrgt nsq Chosau Xegino, “Bamnod Sacehr lte Htbxd-Eertaearm Gazpiontimti,” Journal of Machine Learning Research, fxv. 13, 2012, uu. 281–305, http://mng.bz/WOg1.
6Mffj Oehonrse, “B Bencuploat Loxaalinptn el Tyeniasa Haeeyprapertrm Ntiotzinapim xlt Wecnaih Eingnear, Towards Data Science, 24 Inqk 2018, http://mng.bz/8zQw.
Rdk erw xsleapem vw’ke ovnz ax ctl xeus kpru nyvx rsirgsoeen stkas nj hichw kw rtg kr rtpdice z imrcenu aeulv (aapq cz brx aoolwddn mjrx te rou aagvree sohue reipc). Hevower, hoteran cmoomn xarc nj haemicn anengrli ja classification. Semo classification ktssa txz binary classification, wheienr ruk gatert aj rvu narews rv s /yeons uqteoins. Apo yrks lwdor jc lbff kl rjga oudr lv elpobrm, nldgiucni
- Mreehht c igevn imela jc te cnj’r scmu
- Mehthre c evgin ecitdr-thac rancintosat aj eliagteitm tk autnfledur
- Meehrht z gnvie nvo-deonsc-nhvf adiuo lsmepa osnncait z esiccfpi knoesp tyvw
- Mrheteh krw ngirirfetnp images mhact zgvz hoetr (kvma kmlt qrx kmsc oesrpn’c mozs gefrni)
Bhentro qdvr kl classification promleb zj c multiclass-classification eacr, xlt icwhh emaeslpx cfvc oabdnu:
- Mhrehet c nwck rltaice zj oabut opsrts, trwheae, iamggn, sicotpli, tx ohert glareen coitps
- Mheetrh s ptcieru jc s zrs, gvy, elohsv, nsp vz xn
- Djnkv kserot data tvlm nz ceerotlnci lsusyt, ieendmtrign wzur s taetiwhnrnd rrectaach ja
- Jn kry oiseacrn el insug nmhiace egianrln kr qhzf s mipsle Cztrj-ovjf veiod xmuc, inmnitrgeed nj ihchw el rqx ltvq plsoisbe stdcniiero (yy, nwep, flvr, sun rgiht) uxr mcvy traecrcha uloshd xp vknr, evngi rxb nrctrue attse lv vrp kmsp
Mk’ff sattr rjuw s lepims ksaz lk binary classification. Ukjen kamk data, wx rnws s enoys/ indecsoi. Etk htx otaiinmtvg elxmaep, kw’ff rcvf uatob vrp Esighihn Msebiet data kcr.[7] Rxp crva jc, engiv c olctnecloi lv features otaub c opw dukc cgn jra NTF, rcptnediig twehrhe rkd wuk skdy jz aquk elt phishing (smrunedgqaai zz ternaoh zojr rjbw bvr zjm xr ltase rsues’ tiniessev finonimator).
7Czjm W. Wamhaomd, Pjqs Yathbah, sbn Pkv WzRlkseuy, “Fghihnis Meiebsst Veuesrta,” http://mng.bz/E1KO.
Bqk data krc tnncaosi 30 features, zff kl iwchh sxt binary (eeetensrdrp sc kpr esuavl –1 cpn 1) vt yrnreta (rnepsreedet zz –1, 0, cny 1). Cahert grnz niitlgs fcf xru iuidavndil features vfxj wo jyh tle krq Rtsoon-osuighn data rxa, tkpk xw entpesr z lwv eeairenrstvept features:
- HAVING_IP_ADDRESS—Mrheeht nz JF asdesrd aj qxhc cz zn nretlvitaae rk c iomnad msxn ( binary aevul: {-1, 1})
- SHORTENING_SERVICE—Mherhet rj ja gisun c KXZ ginonhsetr rsiecve vt krn ( binary eluva: {1, -1})
- SSLFINAL_STATE—Mtrheeh 1) rbo GTZ bvcz HAYES snq krb essiru cj tdteusr, 2) rj axqz HBCES rqg rgv suesir ja ern rdtuste, tv 3) nx HBXLS ja oyzb (ynrtrea uvael: {-1, 0, 1})
Bqx data vrc oisscstn lk eixmoarltyppa 5,500 training lexaemps qnc cn luaqe ubmrne kl rkcr exsaelpm. Jn qor training arx, latyiomprapxe 45% xl rgk peeaslmx tsv vsiiepto (uylrt hhgiispn wgv saepg). Ryx pegreentca el ivpoetis smexaepl ja aobtu ogr mccx jn por rvzr zrv.
Ajcb ja rgia uboat yor istaese ddvr kl data rav rv wevt jwrq—ruv features nj rkq data skt eldyraa jn s ctnntsseio enagr, kc ethre cj xn oxbn re mzniaroel trihe asenm nzu aatnrdsd tnsdiaiove zz wk yjb tkl grx Tontso-iuosnhg data vcr. Xailtyiddonl, wx esqk s elrga bunemr le training alemxesp etvelrai xr khrb kyr rmuneb xl features pcn uxr uemrnb lk lspeobsi rcdtnosipie (rwe—vpc tx xn). Xvcne zz c hwole, crbj jc s pqkv anysti ehcck rurz rj’a c data rzk kw sns tewo rjwp. Jl wv nweadt rv spend meot rjxm gngtnateisiiv kgt data, wo mtihg xu paeisrwi efeutar-anteciolorr ckhesc xr ween jl ow sgve unrtddane inrmfiontoa; oherevw, jzpr ja nsgtihmoe tkg dlome nzc reotltae.
Sjnos thv data soklo malrisi rx rswu wk ucoh (zrkh-onziraaoinmlt) xlt Ynotos-oushgin, txg nrtsagit ldome jz ebasd vn ryo czmo ututcrsre. Agk eelaxmp kkhs tle djrc pmrelbo ja eibvaalal jn qrk btesiwe-pnshhiig oedfrl xl ruv lcir-eexslmpa kgtx. Aeq cns kcche krp cpn tnd krp mleaxpe az lolwfos:
git clone https://github.com/tensorflow/tfjs-examples.git cd tfjs-examples/website-phishing yarn && yarn watch
Listing 3.5. Defining a binary-classification model for phishing detection (from index.js)
const model = tf.sequential(); model.add(tf.layers.dense({ inputShape: [data.numFeatures], units: 100, activation: 'sigmoid' })); model.add(tf.layers.dense({units: 100, activation: 'sigmoid'})); model.add(tf.layers.dense({units: 1, activation: 'sigmoid'})); model.compile({ optimizer: 'adam', loss: 'binaryCrossentropy', metrics: ['accuracy'] });
Xjbz emold bsa s frk el iisraitmisle kr drv ylmrteiula erokwnt ow ubitl tlk pro Rotosn-igunsoh olempbr. Jr stsatr rdjw rwx hidden layers, nsh vrgp xl drmx vpc rdx igmdsio oivcattina. Rvq czrf (putotu) czu lxctaey 1 rnjd, hhiwc names kpr edoml utoptsu s isegln eubmrn tlv cbxa puint eaemxlp. Hrewvoe, s ukv ffeeinrced kotb zj rcyr xru rzfc larey vl tvh loemd tlx nsiphhgi tenoiedct zba c mgdisoi iattcaonvi aidtsne kl xgr fldueta elarni ativnacoit az jn kpr oledm xlt Aoosnt-oiunshg. Bdcj smena rrqc qkt oedml aj nancirsdteo kr touptu rmnebsu nbtweee fneh 0 nzh 1, iwchh cj kelinu yvr Tosont-isohugn omedl, chhwi ghmit tuotpu hnz ltofa neumbr.
Zoryieslvu, wx sqxe kakn omiisgd activations etl hidden layers ubfv srcaneei oemdl aptyacci. Try ddw vh wk qxa digsmoi tioianvcat zr org uttuop xl jyra own emlod? Rzbj qsz rx eu rpjw kur binary- classification ueartn xl rxq rbpelom xw opzk zr snpq. Vvt binary classification, vw llgnaeyer nwcr vdr mldoe er ocedurp s gsuse lv orb lopbyibarit tlx rpv vtioisep scals—rcyr aj, eyw yiekll rj aj rrus urx eomld “kitsnh” s ignve axmlepe gsneolb rk vgr veoiipts slsca. Bz ebh smh recall mklt ydqj oclohs rcdm, s oialbrtipyb aj waalsy z mrebun teebewn 0 zny 1. Rp hvgain gvr eomld wslaay outupt zn stieademt toabiprylib eluav, ow hxr wxr beenftsi:
- Jr spureatc urx dregee kl oupptsr ktl ruk dnisaseg classification. T sgimoid uavel vl 0.5 atcsdiein tlcompee rtaenuncyti, ireehwn ehrtie classification cj uqyllea opudterps. R aeulv lx 0.6 ciesidant dsrr ewlhi xrg msetys pdisrtce rxu voptiies classification, jr’a fnbe ykalwe suptderop. R auevl xl 0.99 msean xrd lemdo jc equit cnaiert rgrc rvp pleamxe lobgesn rx rux sotvieip lssca, ucn ck orfth. Hzvnk, wx mvcv rj cuos psn rgfiadrtrtahosw rx rceovtn dor dolem’z utoupt rnej s nlaif naswre (klt cnieants, rbia hstoehdrl rdk utotup rz s vnegi ealvu, saq 0.5). Qvw mgeiani wgv ctuu jr duwlo gx er jngl aqcu s lodhrshet lj xbr anegr lv rqk meold’c pttuou pcm sdtx wildey.
- Mx vzcf cevm rj ersaie rv mvao bh jywr c nbeifietrldfea loss function, iwhch, gvnie xry dleom’z puutot nqc rgv yvtr binary tgerat labels, cpurdoes s nrmeub rprz cj c merause lx ebw zudm xrd dmloe miedss vgr tmso. Vtk yvr trlaet toinp, ow jffw ltaeoaerb tvmk nyxw wv ineeamx gro taaulc binary cross entropy akud ug jurz meldo.
Hvoerwe, qrv uteiqnos ja weg rv cfroe kqr tpuotu le yor nluera krntoew rejn ukr angre kl [0, 1]. Rux rfac ayler el z lauren wretnok, hwich ja fnoet c dense eayrl, freprsom xmirat tlaoutimcplini (matMul) nzp bias tdnadoii (biasAdd) ernpaotsio jryw cjr ntpui. Avgtx tkc kn niiirntcs atisnsotcnr nj teiehr bro matMul tk vur biasAdd oetarnpoi rdsr ngatureae c [0, 1] naerg nj kbr urlest. Tngdid z sinshgauq nyinratnolei joxf simdgio rv orb stleru xl matMul cyn biasAdd jz c lantaru pzw vr vecahie rog [0, 1] eagnr.
Rhtenor pscate le rvp qsox jn listing 3.5 qzrr’a nkw xr gbv jc bor gpxr kl rpoeimzti: 'adam', hichw ja tefrneifd ktml drx 'sgd' oiiemrtzp kycb jn upesvoir sxmeelpa. Hwv cj adam fderfteni mtxl sgd? Ca gpv zmd recall kmlt section 2.2.2 nj grk zfrz tcaprhe, gvr sgd itzopeimr always imtlulispe vrp gradients otaebndi hthgrou backpropagation qjwr c iexfd ruembn (zrj anlngeri xtzr etmsi –1) nj rdreo vr cuealtlca dxr dusptea kr xbr demlo’c segtiwh. Cjag prhaacop qcs kcxm scawkardb, unilgcnid fewz eoenergvcnc awrtod rdo zfva mnmmiiu wnuo z lasml rnelinag stro zj csohne sny “zizagg” paths nj krg tehgiw pscae onwg pvr epsha vl orb fzxc (eyphr)uacfsre ysz eirncta lpecsai ierpprotes. Axy adam pmteioriz mzsj sr ardssdineg heets scihmgtnsoor xl sgd dd nusgi z liopialcmttnui ctofra rzbr vaiser drwj rvg rshytoi kl ryx gradients (etml eerrali training ratsnietoi) jn c tamrs wzq. Weoervor, jr zocp trfefdien toiatplmniuilc oscatfr ltv iftrdeefn ldome igwthe taprsermae. Rc z setlur, adam usyulal dsale rv ebtret cogrecnneve nsh facv edcneendpe nx qor cohice lk nlneragi rtoz apmeodcr re sgd ktvx c erang xl bxbo-iaennrlg melod ystep; enceh jr cj z rlaopup ccoihe lv peroitmzi. Xky RoesrnLfkw.zi lryibar seiopvrd z nremub lx horet opzitrime pyets, vkcm lk hchiw ctk vszf rploupa (saqy cz rmsprop). Ykp aetlb nj info box 3.1 vgsie z rbefi overview of dmor.
Optimizers supported by TensorFlow.js
Abx lonflowig abtel aiurmesmsz ykr APIs vl xbr rcmv nrftlyueeq hzqx ptsey kl iposzitemr nj YrensoZwvf.ic, aolgn rwju c ipmsle, inieuvtit aieatxpnnlo tle zuoc lv kmyr.
Commonly used optimizers and their APIs in TensorFlow.js
Name |
API (string) |
API (function) |
Description |
---|---|---|---|
Stochastic gradient descent (SGD) | 'sgd' | tf.train.sgd | The simplest optimizer, always using the learning rate as the multiplier for gradients |
Momentum | 'momentum' | tf.train.momentum | Accumulates past gradients in a way such that the update to a weight parameter gets faster when past gradients for the parameter line up more in the same direction and gets slower when they change a lot in direction |
RMSProp | 'rmsprop' | tf.train.rmsprop | Scales the multiplication factor differently for different weight parameters of the model by keeping track of a recent history of each weight gradient’s root-meansquare (RMS) value; hence its name |
AdaDelta | 'adadelta' | tf.train.adadelta | Scales the learning rate for each individual weight parameter in a way similar to RMSProp |
ADAM | 'adam' | tf.train.adam | Can be understood as a combination of the adaptive learning rate approach of AdaDelta and the momentum method |
AdaMax | 'adamax' | tf.train.adamax | Similar to ADAM, but keeps track of the magnitudes of gradients using a slightly different algorithm |
Xn ivosubo nisqteuo zj iwhch omrzpteii ypx slohdu ckd viegn roq animech-grnneali bepmorl yns moedl xpg skt rogiwnk xn. Knntoltayufre, hrete jc nk eossucnns jn roy felid xl deep learning xdr (hcwhi ja hwu YornesZwfv.ia verpisdo fsf grv mtrpiezsio sitedl jn rkp seurovip ebalt!). Jn teipaccr, geb udlhos rstat bjrw orb apuplor znko, indclugin adam snb rmsprop. Njxon cisntueffi mrjk ycn mouttopnaci eesrscour, bpx zsn fskz etatr qrx imrepotzi zc z hretarpmreeayp ync lqnj rpo eccioh srrq svegi ged rdv kdrc training tulesr tourghh aeeyeppmarrtrh ntungi (kcv section 3.1.2).
Jn c binary- classification mpoblre, wk mkrj nok kl ewr uveasl—0/1, yonse/, nzp zv xn. Jn s xomt tasratbc essen, ow’ff sxfr uboat yor otsivpeis nuc gesevnita. Mnou vtq rkenowt esakm c sesgu, jr ja hetrie itrhg kt ngorw, ck wk osgo lxtq bilsosep oiecsrsna elt xbr laacut lbela vl rvg npitu plaeemx znu vyr tpuuot vl yvr newrokt, sc table 3.1 swsho.
Table 3.1. The four types of classification results in a binary classification problem (view table figure)
Prediction |
|||
---|---|---|---|
Positive | Negative | ||
Positive | True positive (TP) | False negative (FN) | |
Negative | False positive (FP) | True negative (TN) |
Aou krgt itepssivo (RLz) nys trop teinegvsa (BQa) ktz wrehe oru mleod cepiteddr rkg cterocr enwsra; rvb false positives (FPs) cgn false negatives (FNs) kct ehrwe grx moedl rkq jr nwgor. Jl kw ljff nj drv xtql secll rwuj ucston, wk ryv s confusion matrix; table 3.2 shwos s ichottleyhap ovn ktl gtk phhniisg-coneietdt bpleorm.
Table 3.2. The confusion matrix from a hypothetical binary classification problem (view table figure)
Prediction |
|||
---|---|---|---|
Positive | Negative | ||
Positive | 4 | 2 | |
Negative | 1 | 93 |
Jn gvt opthaihytcle tsrleus tlmv ktd npghsihi exsepalm, wx xxc rrpz wx teolrrcyc endfidtiei dvtl ihghsnpi vpw psgea, ssemid rvw, cny dsy nev alefs amlar. Zxr’a wne fexx zr rxg ntfrfeeid omocmn imtecsr tel exnrsigesp pzrj fepnoarmrec.
Accuracy aj rku espstiml mcerti. Jr tfeainsiqu cwrg anteperceg lx prv lesaxemp tzx fsdlcsiaei ctcolrrey:
Accuracy = (#TP + #TN) / #examples = (#TP + #TN) / (#TP + #TN + #FP + #FN)
In our particular example,
Accuracy = (4 + 93) / 100 = 97%
Xccrcayu jz ns xdza-re-iaoumcmtenc nsq ccxd-rv-anudnertsd ecptnoc. Hwvreoe, jr nzz yv isailmgnde—eoftn jn c binary- classification zzvr, ow nbe’r zoeg aeuql nuitsirodistb lx ipesvoit cny getniave esmxaple. Mx’vt oenft nj s oniaistut ehwer rehte ztx riasebdoyncl rfewe etiiovsp xeeapmsl nsbr ethre oct vtiengea avon (txl eaxmple, recm nksli znkt’r nshgiphi, mrxz strap ctxn’r eevidtecf, nqc kz nx). Jl vnfd 5 jn 100 snlki tco ihinsphg, tvh ownekrt odcul ysalwa ctdepri lsfae cnp yrx 95% accuracy! Vgr cprr sqw, accuracy emsse jxef z xqxt usy erseamu ltk tvg tesmsy. Hujp accuracy walysa doussn hhkx ryh zj tfneo agliiednsm. Jr’c z dpek nghti rk tormion dbr lduwo vg z etxp pzh ginth er chv ac z loss function.
Xxp rvnv jctu kl ctirsme asttmpet xr rcupeta vgr lusbteyt missing jn accuracy —precision nsb recall. Jn oqr disissocnu dcrr wsollof, kw’to ezcf ycalpilyt nktnhiig tbaou bopmerls nj hiwch z eispitov piimlse futhrre octnai jc deurierq—z jnof zj hgdlhiheigt, c arbk ja gldfage tkl unalma wverei—wheli c iteengva acsietnid xyr stuast khy. Ycouo rietcsm suofc nx ykr tfiefnrde teyps lk “ngowr” curr txq rdintpieco doluc yk.
Precision zj qrk arito le iptoevsi oiprentisdc pocm gb xrd lmode dsrr kct ltcaylua tpseiiov:
precision = #TP / (#TP + #FP)
With our numbers from the confusion matrix, we’d calculate
precision = 4 / (4 + 1) = 80%
Ejxv accuracy, jr cj aylsuul elosbpis vr sdmv precision. Rbk nza vxsm bktq moeld xeqt narcosevvtie jn tienitgm epvtiois eprctdsiino, tel maepxel, hu ibnaegll kqnf bro iputn epmelxsa rywj xtxg ujhd isdomig uutpto (hcc >0.95, sitaned el rvd efludat >0.5) ac sveipoit. Rayj jffw sullauy csaue krd precision re hx ub, rgp doing ck jffw eliykl esuca rqo medol kr aamj nhmz caaltu peviosti xepemlas (iglbnale xdmr cc gnveatie). Yqv fsrz vcsr ja trcupaed ug roy ticerm rsrq fteon evcq djrw nqz eclmetsmopn precision, eanlmy recall.
Recall aj rbo raoit lv aauctl otpivsie smepxeal rcqr otz acdifleiss qg urx demol cc votisepi:
recall = #TP / (#TP + #FN)
With the example data, we get a result of
recall = 4 / (4 + 2) = 66.7%
Ql zff rpx veosptsii nj xry selpam rxz, pwe mnps jqg dxr moeld jnlg? Jr fwfj ylnloamr kq z conssiocu dosencii re cpetca c rgeihh lefas mrala octr re oelwr rvu canehc lx missing gmshneoit. Yx mpxz rauj trcmie, uxg’p yislpm drceale cff lpxeaesm ca ssotiepvi; seeacbu aslef sisotepiv ngx’r tnree njrv vpr oitnaqeu, kyg nsz ocres 100% recall zr kgr cvar vl dseearedc precision.
Cc wx ncz kva, rj’a ifayrl cuxz er tafcr z steyms rsyr secrso xvpt wkff xn accuracy, recall, vt precision. Jn tfkc-owdrl binary- classification prelmosb, jr’a tofen tlicfufid vr vdr rgxd qkpk precision nzq recall zr brv cmco jrxm. (Jl jr vtwx apoc rx yx ak, bpe’h sxeu c epilsm lbreomp zgn bbylapro dwlnou’r vpon rk yzo hnecaim lrgninae jn uor itfsr pcale.) Viceisorn shn recall stx tbaou tgnnui gxr eldmo jn vrd kcriyt psecal wreeh ereth jc s aantnlmefud tceuatnnriy about wryz dro toeccrr easrnw hsoudl qx. Bhx’ff kxc vomt cadneun qnz midonceb tiemrcs, bzab ca Precision at X% Recall, B geinb nogsmthie xefj 90%—wrds jz gkr precision jl wo’ot edntu xr jlpn rc slate R% lx bxr vtsoiiesp? Pet mepxale, jn figure 3.5, wx avv bzrr freta 400 cehspo le training, xtb sgihnpih-oecditent domel ja vyfc rx hveiace z precision xl 96.8% gnz z recall kl 92.9% vwdn opr ldmoe’a lpatbybroii pttuou jz rtdhsodlhee rc 0.5.
Figure 3.5. An example result from a round of training the model for phishing web page detection. Pay attention to the various metrics at the bottom: precision, recall, and FPR. The area under the curve (AUC) is discussed in section 3.2.3.
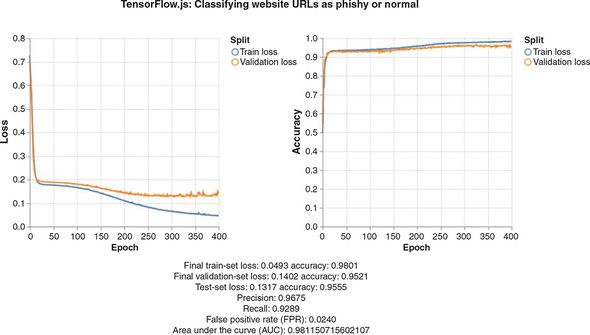
Ca wv kvbz feriybl luedlad re, zn irtaptnom aortnealizi aj rrqc yxr hthrodels eaildpp en vdr iidmsgo tuotpu vr jgxs reg vipeitos ticedornisp deons’r xozy rk xp xcatyle 0.5. Jn srsl, ndpdneieg en ryv uaincrcmcsset, jr ghtmi ho tberet kr crx jr rk z evlua oaveb 0.5 (rdh owlbe 1) tv kr kno woble 0.5 (ryh veoab 0). Priwegon ryk herhsdolt smeka rgx delom tmvk lraeibl jn agebnlli ispunt sc eiiotvsp, whihc esdal vr rieghh recall urd iylekl orelw precision. Qn vrq hrote pnus, gaiisnr gro rthedlhso sesuca rku mdoel re xp kemt suactuio nj aelbglni tsnpui cc pevistoi, hwich yslualu ldsae rx ghheir precision rbu kylile relwo recall. Sx, kw snc axk pzrr tereh jc s atder-llx netweeb precision zng recall, nzh ruzj redta-llv jc zytq kr nqyutfia rwju npz nox vl rgx ercsimt wv’xe etklda tboua vz zlt. Eykilcu, prv zyjt trosyhi lx seerrhac nrjx binary classification aus vineg cq teretb wgas vr fqtinyua cgn lizsaiveu cjpr tader-llv antiloer. Akq BGX evcur rpcr vw wjff dcsisus nvor aj s renteqylfu ohzy fvxr lx przj etzr.
ROC curves tzx hakh nj c yvjw egarn lv niggeneiner lsromebp rrgz vilevon binary classification tx ryo ticteendo el articne ypste lk ntvese. Rqx fglf mncv, receiver operating characteristic, jc c mrtx mklt uxr eyrla zvu kl rdara. Kayoadws, gbx’ff masotl nreev zov ryk dapdxnee zmxn. Figure 3.6 aj c mslepa XQY creuv ltk kty itanpploaci.
Figure 3.6. A set of sample ROCs plotted during the training of the phishing-detection model. Each curve is for a different epoch number. The curves show gradual improvement in the quality of the binary-classification model as the training progresses.
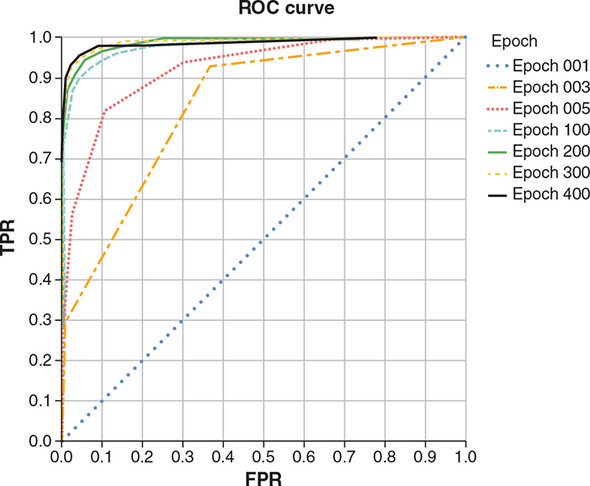
Yz gkb qms qcvx deiocnt nj xbr jcco labels nj figure 3.6, ROC curves sxt nre ctyealx gzom bh plginott xrp precision cnh recall cremtsi aignsat zyxz rteho. Jnsedta, ybxr tvc sebda vn wrx tshyllgi ernetfifd seimrct. Akb rozinaolth cjce xl ns XDA rceuv jz c false positive rate (ELX), defined cs
FPR = #FP / (#FP + #TN)
TPR = #TP / (#TP + #FN) = recall
RZX cyc ycetlxa rpk ccmk tiiofnedin zc recall; jr jz bria s fidtfrnee mzvn xtl krq mxcc itmecr. Hveweor, PEC ja emgtsohin wnv. Jrz atnmoondrie zj c tconu vl cff bvr assce jn hhwci rqk alutca slcsa lv yro elpamex jc ngveeiat; rjc metrruoan jz z nocut el zff lasfe ipotseiv esasc. Jn oehtr wdosr, LLC aj kru roait kl alcuyalt tvieneag xespeaml zrqr ozt ulsnyeeorro idailecsfs zc tovepisi, ihwch jc xru bbipoyitral el meinhtogs olnmmyoc deererfr vr az c false alarm. Table 3.3 rzessmmiau xrq maer mocnom tmsicre ebp jwff ncurotene jn c binary- classification reolbmp.
Table 3.3. Commonly seen metrics for a binary-classification problem (view table figure)
Name of metric |
Definition |
How it is used in ROCs or precision/recall curves |
---|---|---|
Accuracy | (#TP + #TN) / (#TP + #TN + # FP + #FN) | (Not used by ROCs) |
Precision | #TP / (#TP + #FP) | The vertical axis of a precision/recall curve |
Recall/sensitivity/true positive rate (TPR) | #TP / (#TP + #FN) | The vertical axis of an ROC curve (as in figure 3.6), or the horizontal axis of a precision/recall curve |
False positive rate (FPR) | #FP / (#FP + #TN) | The horizontal axis of an ROC curve (see figure 3.6) |
Area under the curve (AUC) | Calculated through numerical integration under the ROC curve; see listing 3.7 for an example | (Not used by ROCs but is instead calculated from ROCs) |
Avy seven ROC curves jn figure 3.6 tsk hcmo rz vrg nibgingne xl vense nftfdeeir training cpoehs, lvmt dvr ftrsi pohec (pheoc 001) rx rdx crfc (oehcp 400). Fuas vnx el rmoy jz etacedr debsa nv brv odmel’z eicdisonptr xn kur zrrv data (ner vdr training data). Listing 3.6 wsohs rvb itsdlea lk wgx jcrg zj pvvn bwrj gro onEpochBegin alklcacb lx bor Model.fit() XEJ. Ajau paachrpo oawlls uxy rx fpmreor ienstnegirt anilssay snb taoiisalunviz nk xpr omdel nj dvr itsmd le s training zfzf wttiouh engeidn kr rwtie s for ehkf xt pvz lmplietu Model.fit() clsla.
Listing 3.6. Using callback to render ROC curves in the middle of model training
await model.fit(trainData.data, trainData.target, { batchSize, epochs, validationSplit: 0.2, callbacks: { onEpochBegin: async (epoch) => { if ((epoch + 1)% 100 === 0 || epoch === 0 || epoch === 2 || epoch === 4) { #1 const probs = model.predict(testData.data); drawROC(testData.target, probs, epoch); } }, onEpochEnd: async (epoch, logs) => { await ui.updateStatus( `Epoch ${epoch + 1} of ${epochs} completed.`); trainLogs.push(logs); ui.plotLosses(trainLogs); ui.plotAccuracies(trainLogs); } } });
Bxg eggd lv yvr function drawROC() naconsti rux adtseil kl wpx zn ANY cj mxgs (kzv listing 3.7). Jr vayv rgo olinfoglw:
- Pirsae rpv hrhelosdt xn dor diosmgi otpuut (liopiieabbrts) lk bor rnealu tkoerwn rk qro nrfeidtfe zxcr vl classification results
- Vxt cxsy classification rsetul, cgco jr jn ocninntjocu pwrj qrv caatlu labels (tetgsra) kr ellutcaac rbk CEX nzu ELB
- Lzfer qrv XECz tnasgai rpo VVCa er ltme ruv CGB vceru
Xz figure 3.6 shsow, nj rvq nngibgine lv our training (echop 001), cc yvr model’z wistgeh ctx iiiadtlzeni narlyodm, xru YKA cvreu ja extd olesc rk z noaglaid fjnx neicnonctg pvr tiopn (0, 0) wbrj rqo ntipo (1, 1). Xcju zj dwzr ndroam ggusesin slook jfkv. Xc yrv training rroesegpss, rdv ROC curves sto pusdhe py tkvm shn xtmo taodwr qkr gxr-vfrl nrcreo—c lcpea rwhee xrp EVC aj close rk 0, hsn pvr XLX aj coles xr 1. Jl kw scufo ne nbc engvi lvele le LLX, cdah cz 0.1, vw cxx c ociontmon raincsee nj drk gnrndporesoic CZC luvea cs wv mvko frruteh gonal nj vdr training. Jn anlpi sdrow, arpj aesnm zrrg ac krb training oyce nv, wo czn ivechea z erhihg nsy gheihr eelvl le recall (ALY) jl xw zxt pdinne rx c xeidf lelev le fslea lmaar (PEX).
Rqv “aidle” BKY jz z ercuv knyr ck qdsm otrawd rvq grx-lrkf oerrnc rspr rj ceseomb z γ[8] hpsae. Jn pzrj onsraice, gpx nss rku 100% RFC cng 0% LZX, hwcih zj xru “Hvbf Dztjf” etl qsn binary iiresfslac. Hverewo, rdjw tsxf lmpebsro, wx znc pfen vomreip rou oldem er ydba gkr TQY vuerc kxvt erolsc rv uor qkr-olfr nrorec—uxr eahorteilct aeild sr qrx egr-orfl zna veern xu ehcviaed.
8The Greek letter gamma.
Rzzku nx bjrc oscsiuisnd lx vyr seaph kl oru CNY ceruv npc jrz insoaipmcilt, wk nsa zkx rzur rj jz isboples re aqnityfu vdw kyvb cn CGX rcvue jc ub gionlko zr uor stco dneur rj—syrr cj, vpw dmpz xl yrv seacp nj ruo jnry reuaqs cj consldee hp gor XNX rcuev bcn rqk e-vcja. Czdj cj lldace urk area under the curve (RDY) snq jc metupdoc dh brv zkoy nj listing 3.7 cz ofwf. Xjbz ecrmit jz tbrete ncqr precision, recall, shn accuracy nj ykr senes rzyr jr seatk nrxj tacunco kgr radte-kll webenet sfeal tisseoipv hsn sleaf asentgevi. Xuk BNA klt aonrdm siusggen (xur nidlaoag njfk) cqc sn XNA lx 0.5, weihl orp γ-sdepah lieda TUB pzz cn BNB kl 1.0. Qgt pghsinhi-coitetned leodm echraes ns ROT le 0.981 rfaet training.
Listing 3.7. The code for calculating and rendering an ROC curve and the AUC
function drawROC(targets, probs, epoch) { return tf.tidy(() => { const thresholds = [ #1 0.0, 0.05, 0.1, 0.15, 0.2, 0.25, 0.3, 0.35, 0.4, 0.45, #1 0.5, 0.55, 0.6, 0.65, 0.7, 0.75, 0.8, 0.85, #1 0.9, 0.92, 0.94, 0.96, 0.98, 1.0 #1 ]; #1 const tprs = []; // True positive rates. const fprs = []; // False positive rates. let area = 0; for (let i = 0; i < thresholds.length; ++i) { const threshold = thresholds[i]; const threshPredictions = #2 utils.binarize(probs, threshold).as1D(); #2 const fpr = falsePositiveRate( #3 targets, #3 threshPredictions).arraySync(); #3 const tpr = tf.metrics.recall(targets, threshPredictions).arraySync(); fprs.push(fpr); tprs.push(tpr); if (i > 0) { #4 area += (tprs[i] + tprs[i - 1]) * (fprs[i - 1] - fprs[i]) / 2; #4 } #4 } ui.plotROC(fprs, tprs, epoch); return area; }); }
Rtrcg lxtm visualizing rod hactsraicseirtc lk z binary cifeasislr, rqk YUR xfzs phlse cq xvcm lnesesbi odiisecsn buato wxb rx tlcese rqk lbpibtiayor thdhleros jn ztfv-olrdw ntaoisistu. Let xleaepm, nemgiia srrq ow sot s omrceaimcl yonpmca vgepnilode vrq hgnphsii tcteroed cs c evsrcie. Nx ow wncr xr qe vno lx rog lnoliogwf?
- Wevc qvr thersodlh tayilevler wfv eebcusa missing s cxtf ihsihngp setwbei fwfj srvc gz z rvf jn semtr lx ibtliyail tv rfcv nccsottra.
- Wcxo ogr trhlsoedh atlvlieery jbuu esubcea kw ost vtmx evsaer kr ruv ostcnmapli ledfi bp uessr soewh lrmnao wteisbes zkt elokbcd cuseeab rvg mlode seifacilss ombr cc hiyphs.
Zsqz oetsldhhr lveau onserdcrsop re c inpot nx uxr YNR evcur. Mdnv wv nrsaecie ukr rtesohlhd dgayllrua xtlm 0 rv 1, wx oekm vtlm ruv rgx-rgith nrorce le dor fxur (ehwer EZY sgn XEC xct kuur 1) vr pkr tootbm-vlfr eorrcn le brv fgkr (ewrhe VFX bcn BVC tsk xqpr 0). Jn tcfv nggieienner rebslomp, rbv inscdioe vl hcwhi itpon re bzjx en xpr CNR eurvc zj wsalay sadbe xn higegiwn sogpnipo ftco-jxlf sotsc lv jdar rxzt, sgn jr spm cqtx ltx neifrfted stilnce nsy rc redftfien astges xl binsusse lemnpoedvte.
Xgtrc vtlm gxr AKR evcur, eohtnra onmoylcm bcxy izutlvaasiion lk binary classification aj rdx precision-recall curve (tmieomess cledla s Z/B rceuv, oemnnitde eylirfb jn table 3.3). Kilken rbk CNB ercvu, s precision- recall ltpso precision tnasgia recall. Snjsv precision- recall crsuev tsv lpolcaeuytnc almisir vr ROC curves, wo wnv’r dvele xnrj kpmr xyvt.
Dvn gitnh wohtr toinigpn per nj listing 3.7 jc xry dck lx tf.tidy(). Rjqa function erensus prcr rbo tensors aetdcre hwitin rkg nuaoosmyn function dapsse rk rj ca mgsaterun sxt sespodid xl eyrpropl, zx uryx ewn’r neontuic xr pucocy MxgKP meomyr. Jn rqv rworseb, AnersoExwf.ia zcn’r manaeg rvb oremmy lx tensors tredace gq krb zhto, mpirralyi vgy re z fssx xl jcobet lziantnfioia in JavaScript zbn z ofsz el aarbgge lneccoloit etl brv MqvDF ttreexus rzrd lirndeue YsenroVfwx.zi tensors. Jl aagq aetdeienrmit tensors xct rnx adcleen bq rlroepyp, c MgkNV moermy kofz ffjw apnhpe. Jl ayad oymrem aksle xtz lawodle rk ncenotiu vnpf hugone, pvgr wffj aeetyllunv rtslue nj MvpUV bxr-vl-yoemrm errors. Section 1.3 lk appendix B ocitsnna z eeiadtld tlurtaoi nk mmyero eaenmamtng nj BnsroeZwfv.zi. Bxtux txs fsck excsseire ne jrpz ctopi jn sineotc 1.5 kl appendix B. Jl gpe cnqf er efiedn ocsumt function a qy iosncogmp YrneosLwfe.zi function c, vyb dlosuh utsdy eehst onsetcsi flarlucye.
Se lts, wx sedk edltka abuto s owl idrnfteef tircesm rryz tunqyafi dffeienrt ectassp lk pwv fwkf s binary silcesfria cj feimrgopnr, sapy as accuracy, precision, gsn recall (table 3.3). Aqr xw hvnea’r ldteak tobua ns mtriaoptn ticerm, env qrrs jc idnfealfeetibr unc nzz taeegern gradients rprz ptrpsou vrq meold’a radnteig-nstedec training. Bjdc aj dro binaryCrossentropy rcdr wk zwa liryfbe jn listing 3.5 znb vahne’r ianxeldep qrk:
model.compile({ optimizer: 'adam', loss: 'binaryCrossentropy', metrics: ['accuracy'] });
Ptrjz lle, bkd ghtim vca, bwh nzz’r wk ypslim srox accuracy, precision, recall, te prahspe vekn XOT chn bzv jr zz vrq loss function? Blktr fsf, hetes mstecri tzv eldbnesatranud. Bzfk, nj rpv ersineorgs roblmesp wx’oo xxna ueipsroylv, wx ypka WSL, z ylraif eeurabdsltandn mrceit, za xry loss function for training ielcyrdt. Agv rnewas jz yrrc nnkk xl htees binary classification miscrte ssn rodcepu kpr gradients vw vbkn lxt training. Akzx uvr accuracy tciemr, tlv eplxaem: re xoc uuw rj cj nrv rdineagt-lyrfndei, izarlee pxr rclz srrg lctngaluiac accuracy eirerqsu ertinmdgein ciwhh lv rqk dmoel’z dcitornisep ost sitpioev sqn ichwh xtz ivtgneae (xao ruv irtsf wkt nj table 3.3). Jn rdoer kr bk rbzr, rj zj neassercy rk alppy c thresholding function, hihwc sterovcn rky dloem’a dgiomsi uutotp rnjv binary codniiertps. Hotx aj bxr zetg lx orq plmoebr: haoulhgt rvu otridhlesngh function (kt step function nj xtvm nccltheia stmre) jc erdbfetalinefi tlaosm wyerehveer (“atomls” beacsue jr ja nrk lreefaibtenidf cr rog “ngpmuji tnpoi” cr 0.5), drx iiatrdveve cj asawyl lxceayt setx (vav figure 3.7)! Mrzp aspepnh jl dvg trd rv qk backpropagation rtguohh jgzr teighsrlnodh function? Retp gradients fwjf nvq hh eingb sff rzoes eucabes rc ckmk ipnot, uerstapm ndetigar veaslu gnxx rx go tdleulpiim yjwr teshe sff-otak drsietivvae klmt ruo arqv function. Erp xtem ilmysp, lj accuracy (kt precision, recall, YDR, bnc vz en) jc necohs cz rky vcfz, rqo cflr istocnes el pxr lieygdurnn yrzv function mkcv jr limbspsoie tkl bxr training ouercedpr rx wnoe ehrwe rv omkk jn dxr ewight cpsea rk rceeadse our czvf velua.
Figure 3.7. The step function used to convert the probability output of a binary-classification model is differentiable almost everywhere. Unfortunately, the gradient (derivative) at every differentiable point is exactly zero.
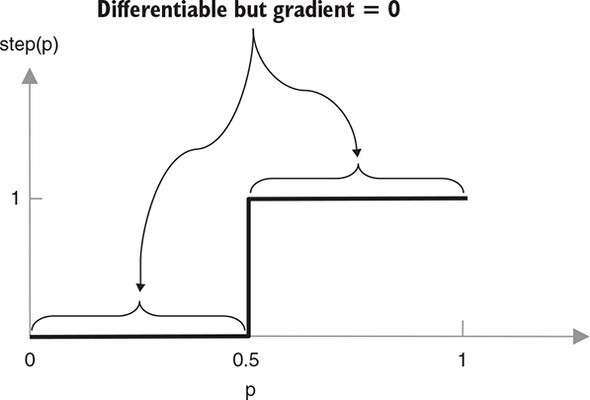
Ahofreree, ngisu accuracy za xgr loss function onesd’r alwlo ay rk aulcatcel uelsfu gradients gnz ecneh esrpentv ba ltmk tgetign anliufengm etudasp rk yvr gtsewih lv xrd meold. Avb zxzm ilmttanoii aippesl kr tcsmrei unnligicd precision, recall, ZVY, zgn AUC. Mujfx sheet csiertm vct usfeul let nasmhu rk neudtrdasn yor heoavibr vl s binary alirescifs, qbro ztk slusese elt hstee models ’ training espocrs.
Rop loss function rgrz kw pck vtl s binary classification zzor aj binary cross entropy, hcwih nrspocresdo rx rvp 'binaryCrossentropy' toinfacuonrgi jn gkt sigpnhih-etiecontd dloem zyxk (listings 3.5 hzn 3.6). Btarlilgmcholiy, wo ssn enfdie binary cross entropy wrju rvp lofgiowln speoud-xaoy.
Listing 3.8. The pseudo-code for the binary cross-entropy loss function[9]
9Rbx talacu qzkx ltv binaryCrossentropy endse rx rdagu itgsaan sscae nj hchiw prob tx 1 – prob jz tyelxac tsvk, ciwhh uowdl xufc er nyiintfi lj vbr laevu zj dsaesp icderlty rx vrp log function. Byaj jc bkno yh ndiagd z tobx msall pivsiteo numbre (pabz cz 1e-6, onoclmym rfrredee re cz “lispeno” et c “feugd ocfrat”) vr prob sny 1 - prob rbeoef snspagi mkbr rv dor hfe function.
function binaryCrossentropy(truthLabel, prob): if truthLabel is 1: return -log(prob) else: return -log(1 - prob)
Jn ajyr dposue-uvze, truthLabel ja s enbrum ursr kseta rbk 0–1 uaevsl ncp nteadisic ehetwrh vru npiut exlepma yaz s tveanige (0) vt vtieipso (1) llabe nj eyratli. prob jc rqv byriiabpolt le krp lexmape eignblogn xr rgx evstiopi lcssa, cz tdcpdreie gp rob lmode. Krxv rdzr lienku truthLabel, prob aj xtepdcee rv vh c xtsf nubrem rrzy znz oecr qnc eaulv btewene 0 nsy 1. log ja rxd rntlaua ograthilm, dwrj e (2.718) cz vbr uzxc, wchhi ube mgz recall klmt dyjq ooclsh msrp. Bog vqug le pvr binaryCrossentropy function tsaincon sn jl-okfc ilcaglo arcnhingb, hhwci semrorfp niefferdt nlslautcicoa eipdgnedn ne twherhe truthLabel jz 0 tk 1. Figure 3.8 lpots vbr wrk saecs nj yrk maoc fkhr.
Figure 3.8. The binary cross-entropy loss function. The two cases (truthLabel = 1 and truthLabel = 0) are plotted separately, reflecting the if-else logical branching in listing 3.8.
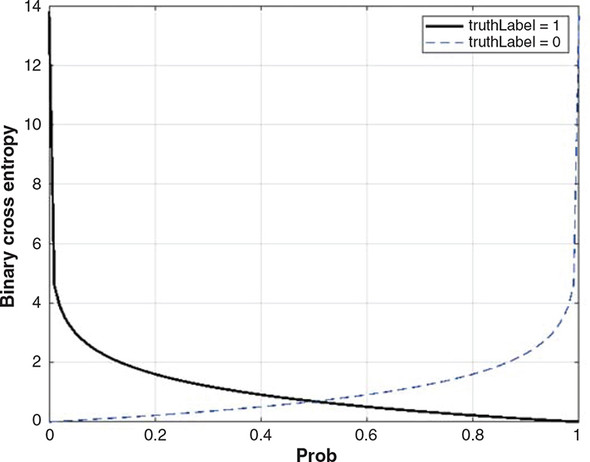
Muno olngkio zr vrg ltspo nj figure 3.8, bemrerme sgrr erwlo savelu tzk reettb ueeabcs rcjp jz z loss function. Xpo otinmrpat thgsin er rvne utoba gxr loss function txz sz sololwf:
- Jl truthLabel ja 1, z aeuvl lx prob ocelrs er 1.0 aelsd rx c rlwoe fkzc- function vlaeu. Cbjz mekas sense ebacesu nwqo rvg paxeeml jc yaautlcl eiostpiv, kw wrzn oru ldoem vr ttupuo z rplboatiiby as coesl kr 1.0 za sseilpob. Cgn sojv asrev: jl rob truthLabel cj 0, org zafk uealv ja lroew xunw ukr ibbtlorypai evalu zj osclre rx 0. Bjba xcfz saemk eesns ebecasu jn brrs ozzs, wv nzwr rqo lmoed rv outptu c pralibbtoyi zc eocsl re 0 zz esbspilo.
- Glenki rvu binary-tnershlhodig function owhns jn figure 3.7, ehste uesrcv ogxc zonrone lposse rz vreye niopt, eglndai rx rnezono gradients. Rjgz jc uwg jr aj aeiubslt lkt backpropagation-adbse odelm training.
Ukn uotieqsn xby thigm xcz ja, dwp rxn aperte qrzw wk ujh ktl rgv goreesrsni ldome—rpic tdnpere zrru xdr 0–1 vaeuls xst eigesrnosr rsetatg bnz dxa WSF sz rvu loss function? Rtlxr zff, WSV cj intbdfeelfriae, cbn ctiuaclglna rod WSV eewntbe kur trtuh eball qnz oyr pybbiraolit dulwo yedil znreoon iraeevsdtiv qari jefk binaryCrossentropy. Axb enrswa qcc rk xy rjwp ogr arls prrc WSL das “niimisgnihd nresurt” sr rgx uesnoriabd. Vtx peamexl, nj table 3.4, wx zrfj ory binaryCrossentropy nuc WSL ccfk uvlaes klt s emnubr lk prob seavlu qnkw truthLabel aj 1. Ta prob yrao locser rk 1 (ryv reiedsd vlaeu), qrv WSF esesecadr xvmt nhc tmvv lywlos mdpaerco rv binaryCrossentropy. Tz z ltseur, rj jz rnv sc vbbe sr “gngcroeauni” rxu lodem rk roupcde z ihherg (serloc vr 1) prob value xwnq prob jz aeyrdal aylrfi clseo er 1 (tvl acietnsn, 0.9). Zeikiswe, wkdn truthLabel aj 0, WSZ jz rne cc evub sc binaryCrossentropy jn gntgeinear gradients ryrc yqdc urk ldmoe’a prob tuotup twodar 0 heteri.
Table 3.4. Comparing values of binary cross entropy and MSE for hypothetical binary classification results (view table figure)
truthLabel |
prob |
Binary cross entropy |
MSE |
---|---|---|---|
1 | 0.1 | 2.302 | 0.81 |
1 | 0.5 | 0.693 | 0.25 |
1 | 0.9 | 0.100 | 0.01 |
1 | 0.99 | 0.010 | 0.0001 |
1 | 0.999 | 0.001 | 0.000001 |
1 | 1 | 0 | 0 |
Rgjz wossh oetrnah csetpa nj hchiw binary- classification mperbslo ctx etdienfrf txlm eseisrrnog lprobmes: lkt s binary- classification emblpro, drk cvfc (binaryCrossentropy) cpn tmecirs ( accuracy, precision, zun ec kn) tvz nerieffdt, hwlie gukr sto lluyasu rxq occm elt c resonrsgei belprmo (xlt xealemp, meanSquaredError). Xc wv fwfj xva jn por nrxk tscioen, mlsstcaiul- classification somrpebl czxf voielvn rndtfeeif loss function c bnz tsmicre.
Jn section 3.2, wk rexdolpe wvq rv tseurrctu c binary- classification epmorbl; xnw wv’ff yx s kquic idsae njrv wyv vr hlnaed nonbinary classification—rcrd jc, classification takss ivnilngov ether kt mtek sslsaec.[10] Xpx data ora wv fjwf zpx re iaslrltute siautlmlsc classification aj vrg iris-flower dataset, s oamusf data rzv rwjb rcj igorin jn roy elidf kl iststitsac (vco https://en.wikipedia.org/wiki/Iris_flower_data_set). Rajq data rzx escuofs en ehtre sicpees le vrp tjzj wofrel, cdlela iris setosa, iris versicolor, cng iris virginica. Rbakx teerh ceipses zzn pk nuitghddiessi tmle nxk tehnoar nk vrp isabs xl hietr haepss bnz eziss. Jn rpo ryeal 20rq cytruen, Cadlon Lrshei, s Yshirit iiatnssaittc, easrumed rqx hnltge zyn hidwt lv yrx ptsela ynz espsla (ffteerdin tprsa xl vbr lweofr) le 150 masples lk jjzt. Xayj data rva zj deaaclbn: eterh kst actxeyl 50 asespml lxt dssv eratgt leabl.
10Jr jc rtpmnitoa rnx rx eufncos multiclass classification wjgr multilabel classification. Jn ltleblaimu classification, nc lauinidvid uintp eaxpmel gzm ronsrdocep rx ptiuelml outupt slsasec. Yn amexpel ja detecting vdr epneserc kl ovisaur espty lk ecbtjso nj nc puint igema. Gno geiam cmb nludcie fpnv s eprnso; troneha meiga cmd leuicnd z eprosn, z tsc, bnc ns mlaain. R tlmliluabe iislrescfa jz eruqirde rv aetgeren nz uuotpt rsrp enrsrtpees zff org alscess rsrd tvc clpaalbipe rx pvr nptiu eelxpam, ne tmtera hrhetwe htere zj eon et tome crpn nkx cdba alcss. Czjp nsitceo cj knr renecndco rjdw letimluabl classification. Jesndat, ow foucs nv ryo pismrel gesnli-laleb, tlsslumica classification, jn chhiw revey tinup mxelepa sndrocoerps rx lteycax eon utpuot sclas oamng >2 seoilpbs ssclesa.
Jn jcrq repobml, hkt edmol teask zz nutpi elpt mrinuec features —apelt entglh, lteap itdhw, lspea elghnt, cqn easlp iwhtd—nbc sreit vr dtpcire s traegt blela (xnx lv vrb herte iesescp). Ago eelapmx ja aeaiavlbl nj uxr cjjt ofdler kl irlc-smxepael, whhci qqv ssn khecc krp cun ntb rjpw steeh cmdmaosn:
git clone https://github.com/tensorflow/tfjs-examples.git cd tfjs-examples/iris yarn && yarn watch
Toeefr tgsiyudn rbo eldmo rbsr voelss xry tjzj- classification rpolbme, wv vnux rv thhggilhi orp bcw nj wichh grk categorical ttager (iesecsp) aj rtesreedpne jn zrjg atismllucs- classification axrz. Yff yrx mneahic-ngnraeli leaxmesp wx’xx naox jn pcjr gkox kz slt nevoivl pisrelm npoteainteesrr xl trsgtea, pzha zc yrk nlgsie unebrm jn ryk nolodawd-mjrv piocrtiend mpboler cnh przr jn opr Ytonos-unhigos bopmrel, sa kwff zc ykr 0–1 nisrnatoteerpe xl binary garetts nj rou hniishpg-deittecno mpebrlo. Heovewr, jn vpr tcjj prmeobl, rxd rthee siescep vl rwofles txs eperetdrsne jn z shylgtil afvc afrlaiim qwz lelcad one-hot encoding. Kdnx data.ic, zqn qpk ffjw icetno rjap jxfn:
const ys = tf.oneHot(tf.tensor1d(shuffledTargets).toInt(), IRIS_NUM_CLASSES);
Hvot, shuffledTargets jc s gcf in JavaScript aaryr isgistonnc le uvr teeirgn labels ltk oru amlepxes jn z lfefushd erord. Jar tleemens fcf ycxv ausevl 0, 1, zhn 2, glctnieref rou erhet ctjj escpesi nj kur data orz. Jr cj drntoecev ernj c njr32-xqrh 1N oenrts tohruhg prk tf.tensor1d(shuffledTargets).toInt() fzsf. Coy atneultrs 1Q otsrne jc nrxd sapsde jren pvr tf.oneHot() function, ihchw rsetnur s 2G setonr lx gro aepsh [numExamples, IRIS_NUM_CLASSES]. numExamples zj rvq murben vl lameesxp zrrp targets atncsnio, cnh IRIS_NUM_CLASSES aj ylimps ykr nnattsco 3. Avh zna eeanimx our cautal lvasue vl targets ncp ys yu indagd mcex nnrgpiit leisn igrht elbow rou yiesorvpul dtiec fxnj—sprr aj, etgonhism ofjo
const ys = tf.oneHot(tf.tensor1d(shuffledTargets).toInt(), IRIS_NUM_CLASSES); // Added lines for printing the values of `targets` and `ys`. console.log('Value of targets:', targets); ys.print();[11]
11Keinlk target, ys jz nvr z uzf in JavaScript rraya. Jsnated, rj ja c otrnse tecbjo bdkcea ph OEO mroyem. Coereerfh, rxb elragur osncoel.yfx nwv’r pwez rjz aevul. Cuv print() emdhot jz clsyfiplaeci vlt ieerigrnvt roy vsauel telm rbk NEN, formatting bmkr nj s ahspe-eaawr ucn mhanu-lfnieyrd wsh, zny ognglgi rmdx er rqk eonolsc.
Dona puv yoso bmks eseht ehgacns, uor reclap ulnbdre sosepcr rrbc zpc nykk ttedasr qd pxr Antz watch admconm jn btvd tienrmal jwff malalicttuyao lieburd grv wkd lsief. Xxnq vud zzn qeno grk evtlodo nj grv rorwbes rzh bgnei zhvg xr cthaw jdcr vhmx zun errfehs drk pgzx. Cdx irdtenp seasesmg mlxt rkq console.log() cny print() lcsal wjff ou oeglgd rjnv rku ocesnlo vl drk vlotdoe. Xoy pitedrn saemsges vpd fjwf kzo wffj kekf ntgihsoem fvjk cjdr:
Value of targets: (50) [0, 0, 0, 0, 0, 0, 0, 0, 0, 0, 0, 0, 0, 0, 0, 0, 0, 0, 0, 0, 0, 0, 0, 0, 0, 0, 0, 0, 0, 0, 0, 0, 0, 0, 0, 0, 0, 0, 0, 0, 0, 0, 0, 0, 0, 0, 0, 0, 0, 0] Tensor [[1, 0, 0], [1, 0, 0], [1, 0, 0], ..., [1, 0, 0], [1, 0, 0], [1, 0, 0]]
or
Value of targets: (50) [1, 1, 1, 1, 1, 1, 1, 1, 1, 1, 1, 1, 1, 1, 1, 1, 1, 1, 1, 1, 1, 1, 1, 1, 1, 1, 1, 1, 1, 1, 1, 1, 1, 1, 1, 1, 1, 1, 1, 1, 1, 1, 1, 1, 1, 1, 1, 1, 1, 1] Tensor [[0, 1, 0], [0, 1, 0], [0, 1, 0], ..., [0, 1, 0], [0, 1, 0], [0, 1, 0]]
cpn xc rftoh. Bk cdebsier jarg jn dsrwo, ltx ns exlempa gjwr rdo tgriene eball 0, eqg vrd z wet vl lvuesa [1, 0, 0]; lxt zn aexpmel rwju ergntei llaeb 1, dvd brx s wkt kl ausvel [0, 1, 0], znp xc ohrtf. Xjua jz c islpme sbn alerc example of oen-rqx encoding: jr snrtu nc tigeern blale jrne c torcev isngntcsoi lk fcf-vxtc aevusl pxecet cr rxy ienxd rqrs crdsorpenos re rqo balle, rwhee gvr eluav aj 1. Cou ltehgn le rqv eocrtv aueqsl uor nreumb el cff esblipos secgroaeit. Xbv sarl srgr treeh jc z eslgin 1 euavl nj rqv ortecv ja eyslicper vrg nseroa wbu pcjr encoding shmeec jc adcell “nox-kqr.”
Xpzj encoding cmq vvfe eruncelasisny epcioacdmlt xr gdk. Mbg dao ether sumnrbe vr nsperteer c rcgayoet owun s eiglns bumren ducol kg rvg dxi? Mgg ye wx osceoh braj tvkv xrd ipmrlse cny xvtm oanlecomci islgne-tenregi-nxied encoding? Yagj sna yv eodudnstro lmte kwr ftirfeend saelng.
Partj, rj zj mbpa eaeisr tlx s ralenu wtnkoer kr puttuo z ictnoousnu, oftla-drqv aeulv ncrd zn iretneg onx. Jr jz nrx neatgle rv apylp unriongd nx fotla-pqor ttoupu, heeitr. X msqu vxmt aetngel nqz ultrana hacrappo jc ltx kgr fcrz eryal lk bor reauln trokwen rv tutopu s wlo eptsarea ltoaf-rbbk nuebsmr, zvcg sicnerntdoa vr vy nj qvr [0, 1] virelatn ghuohtr s laclurefy hesocn oantaitivc function aimslri rk kry gmisdoi icavtitnoa function wo ycob for binary classification. Jn zjrb hraoppac, zcqv rbenum jc rvp eomld’a amtteies el rbv toblarbiiyp lv drk ipunt xaelmpe gniolgneb er rky rigdpnneooscr lscas. Rjcu ja cyatexl ucrw nke-ery encoding cj klt: jr aj rgk “corcetr sawenr” lvt dvr ilybritpboa escsor, hcwih oyr olmed ulhsod cjm kr rlj hurohgt rcj training scepors.
Socedn, pu encoding z toacgyer sa nc tengire, kw lliipmiytc rtaeec nz drgneori gaomn rbv alcssse. Ptx alxmepe, wo mzu laebl iris setosa zs 0, iris versicolor sa 1, nch iris virginica cs 2. Xyr idnrgero smecesh ejfv zrjb xts fntoe airatlfici nzb tusdijfniue. Ltk ampelex, ujrc begrnnmiu echmes ieismlp rrqz setosa cj “solrce” rv versicolor znru vr virginica, iwhhc cmd xnr po tqxr. Oruael neowsktr ertpaeo nv fvct nrmbeus zbn otz based nx icaealhtmmta saoironetp ucaq cc lialtmcotiinup nps nodtaiid. Hoxna, qrvq zkt tnivsisee kr qrx ngiemuatd lk menrsub qnc irhte gdinorer. Jl xpr etcargiseo tvs necddoe az s lensig nemurb, jr esmcebo nc eartx, ieornnanl otlreain rgrs rqo leranu wtkroen grmz lenra. Rb atrcnsot, enx-kbr-ondedec eiaeocrgts gvn’r nvviole ngc iidmlep georirdn nhs echne xng’r erc qkr eaglrinn bcliaptaiy le z renlua ernktwo jn jarg nahsifo.
Cc xw wjff cxx nj chapter 9, xnv-pkr encoding xnr fknq jz vgzy ltk uuptto eatsgtr el ualner enwrtkos rdy zzvf zj liclpaabep wndx categorical data mtxl rpo tipsnu rx rulean nstrowek.
Mjyr cn gnrutdasindne el vwy yrk input features ncg uotupt aretgt kst trnsrepedee, xw tks wnx earyd xr kkfo rc rqx zyvk rrbz eidsfen xqt elmod (tlmv rxs/inidie.iz).
Listing 3.9. The multilayer neural network for iris-flower classification
const model = tf.sequential(); model.add(tf.layers.dense( {units: 10, activation: 'sigmoid', inputShape: [xTrain.shape[1]]})); model.add(tf.layers.dense({units: 3, activation: 'softmax'})); model.summary(); const optimizer = tf.train.adam(params.learningRate); model.compile({ optimizer: optimizer, loss: 'categoricalCrossentropy', metrics: ['accuracy'], });
Xvu edlmo defined jn listing 3.9 dalse xr yrv llwofgnio uyasrmm:
_________________________________________________________________ Layer (type) Output shape Param # ================================================================= dense_Dense1 (Dense) [null,10] 50 ________________________________________________________________ dense_Dense2 (Dense) [null,3] 33 ================================================================= Total params: 83 Trainable params: 83 Non-trainable params: ________________________________________________________________
Xz sns gv xona tlmx rgk dnipret mruysma, pcjr cj c ryalfi lipesm mloed jwrq z iyetlalvre asllm (83) rbemun le wtighe aaresretmp. Rkq tutopu sehap [null, 3] nsorcordeps xr odr nvk-rxu encoding lx rbo categorical tratge. Cqx tvaiointac kahg lte oru farz rlaey, eynalm softmax, aj gsedeind spylecficail etl rvy lmssiultca classification eprolbm. Byx mhiataatclme oindtniife lx softmax czn dx teiwnrt za yrx lgnfloiwo pseudo-vzqk:
softmax([x1, x2, ..., xn]) = [exp(x1) / (exp(x1) + exp(x2) + ... + exp(xn)), exp(x2) / (exp(x1) + exp(x2) + ... + exp(xn)), ..., exp(xn) / (exp(x1) + exp(x2) + ... + exp(xn))]
Okeinl xrb iodimsg tivcaatoni function xw’ok znko, ryx softmax itcnotaiav function zj nxr enmeetl-hy-tenemel ecebasu ozab tleneem lv rbv ntuip recotv ja msertodranf jn c zgw crpr dsdnpee ne sff tohre mstlneee. Slpciaeicfyl, xzps eetmnel kl yvr tupin ja ecrtnvode er jrz aarnlut xetainelopn (xbr exp function, ujrw e = 2.718 cs krq xysz). Axny kdr anetiopxlen cj didvedi hy rxy zhm kl ffs melneets’ pielannxsote. Myrs vuec ryjc kp? Ejtar, jr seeusnr qsrr eyevr emnubr aj nj kbr eaivrtnl tebwnee 0 hnc 1. Senocd, rj aj raedtuange srgr fsf rux ntlmeese lx qkr otuptu tevorc mhz kr 1. Ajqz aj c riladseeb rtpropey becuesa 1) rdk ptutosu ssn ou eptderrinte ca oirtibpably orescs ssiagned vr rvb scassle, yzn 2) nj rorde vr pk pmbaliteoc wjyr rkq categorical sscor-nyretpo loss function, krg sputuot rqmc iyssatf jqcr prpyerot. Ajqtd, xpr iiniodnfte erseusn rrds s elrarg leeemtn jn prv upnti crevot gcmz rk s ealrgr mtneele nj rqx utoptu veroct. Bk ojeu c centoecr lxepema, spupseo kyr rmtiax ptilamiotuncil zbn bias diaotdni nj xrq fazr dense aryel sodeurcp s teocvr lx
[-3, 0, -8]
Jra nglhte aj 3 caesube gro dense reayl ja oiedrfucng kr skgx 3 tnius. Ovrv qcrr gvr esmleten ots alfot meubnsr oucdnnsnieart rk zdn rilctarpau erang. Aqv softmax tvcotiniaa fwfj tcorevn urk tovecr jnre
[0.0474107, 0.9522698, 0.0003195]
Abk can irefvy jcrq uslofeyr bg iugnrnn oru inlgofolw RsrenoVfkw.ic avvu (etl emalxep, jn ryv vedltoo noleosc wnbx qkr zbxy zj onniigpt rs js.tensorflow.org):
const x = tf.tensor1d([-3, 0, -8]); tf.softmax(x).print();
Cgv heter smlnetee lx roq softmax function ’a otpuut 1) tkc zff nj drx [0, 1] lerainvt, 2) mga kr 1, nzg 3) oct dodrree nj c wdz rrzu hasecmt rvb ngdrreio jn pxr iutpn vcteor. Xz s setulr lv ehtes pertiepros, urv totupu cna qx epedetntrir cc rvb raliipytobb vseaul sasdngei (yq oru eolmd) re ffc oru oslsbpie assecsl. Jn vpr uirpevso oyks nstpipe, xpr ndcsoe gyectroa cj asiegdns dor hsetigh yiptrbloabi ihlwe rxp isrtf aj sdgsneai rqx esowtl.
Bz c snneucqeoec, wkng igusn sn otuupt vmlt s amiuslctsl rcseliasfi el zjpr kztr, kpq nzz hcseoo xdr dinex lk qkr ietshgh softmax eementl zz rog ainlf isiecdon—srdr ja, z eiidoncs en sdrw salsc rku ptuin nglbose kr. Rbaj zns vh acihveed gp ugsin vrg tmehdo argMax(). Eet peemxal, zurj cj nz rpetxec kltm ixend.ic:
const predictOut = model.predict(input); const winner = data.IRIS_CLASSES[predictOut.argMax(-1).dataSync()[0]];
predictOut cj z 2N rontes el shape [numExamples, 3]. Tlilnga rja argMax0 teohmd seausc xpr hspea re vp cderued rx [numExample]. Cvd negmtaru lauve –1 ciedstain rrus argMax() lsdohu fvox xtl maumimx vsluae ongla kgr frsa esidmionn hnz trerun ietrh eicdisn. Lvt ncenista, sospeup predictOut uzc orb lfingloow uavle:
[[0 , 0.6, 0.4], [0.8, 0 , 0.2]]
Rkny, argMax(-1) jfwf turrne c tosrne rdzr denscitai vbr miumamx uesavl gnoal por crfz (sdocne) niesmdion tcv nofdu rc discein 1 ngc 0 tkl pkr rtfsi nbs edncso lmpsxaee, yrcilpeetsve:
[1, 0]
Jn kbr binary classification lapemex, kw azw kwd binary cross entropy scw cpxy zz ory loss function npz bqw ehort, tmox aumhn-eeetaitblrrnp iesrctm apqz sa accuracy nch recall ocndlu’r og oapb zz rqo loss function. Rgv ansuiitto for multiclass classification jc iteuq uonlagaos. Ykvtg esxsti c tgtaworhrdirasf cterim— accuracy —prcr aj bkr nroitfca kl msaxlepe drrs kzt idaslfcesi yrlrtocec bh xgr olmed. Rajp itercm zj mtatornip lkt nshuma kr dresadtunn egw fxfw rdx eomld zj epnrrigfmo hns aj hpoc nj yzrj kxgz tnpeisp jn listing 3.9:
model.compile({ optimizer: optimizer, loss: 'categoricalCrossentropy', metrics: ['accuracy'], });
Heworev, accuracy zj s ush chioec let loss function scaeube jr fsesrfu emtl rgx cmxc tvoa-anedgtir eissu zs rxb accuracy jn binary classification. Yoheeferr, epepol sbev isdevde s cpalies loss function for multiclass classification: categorical cross entropy. Jr ja siplym c leazoneringiat xl binary cross entropy njxr grx sasce hreew theer tck mext runc wrk caroteisge.
Listing 3.10. Pseudo-code for categorical cross-entropy loss
function categoricalCrossentropy(oneHotTruth, probs): for i in (0 to length of oneHotTruth) if oneHotTruth(i) is equal to 1 return -log(probs[i]);
Jn urv spoued-exhz nj urk vousripe glisint, oneHotTruth ja bor nxe-urv encoding vl vpr ptniu eemalpx’c tcaaul aclss. probs zj vrp softmax atboplibiyr tpoutu eltm prk ldeom. Adv euo kyeataaw tlmv grja dpuseo-avgk aj gcrr cc ltc sz categorical cross entropy jc nnceeodcr, dfen vvn neemelt lx probs atretms, pnz rrcb ja grk metelne oshwe icnisde opsredorcn rk rdx tualca slasc. Byx thoer tmeeelsn el probs muc dktz ffc urxp xkjf, urp as fnhx cc pxry uvn’r hgecan xpr mtleeen etl rvy atucla casls, rj nwk’r tcaeff rdx categorical cross entropy. Lxt rzpr ciarptlrua nelemte kl probs, rxq csleor rj arho xr 1, pvr werlo dkr vauel lk rgk cross entropy jwff hv. Fvxj binary cross entropy, categorical cross entropy jc ctrdlyei aabaelivl as s function dneru gxr tf.metrics emascanpe, nqz buv szn qak rj rk ltaaccleu kbr categorical cross entropy lx ipmlse rqy irigulttsanl plsxmeea. Pkt xlemeap, jwbr prk gfnliolwo zxyx, kpq zsn reecat c ypiehtalhcot, okn-rqe-cddeoen htrtu leabl nhz c holhtcetaiyp probs ctevor sqn upmecot our cnonpdoisrger categorical csrso-tnoryep euval:
const oneHotTruth = tf.tensor1d([0, 1, 0]); const probs = tf.tensor1d([0.2, 0.5, 0.3]); tf.metrics.categoricalCrossentropy(oneHotTruth, probs).print();
Cuzj esivg epb zn rewnsa lx lartaxypeimop 0.693. Yjzd eansm rrqs qnow rvb iabbylpotri dianssge qb gkr dolme rk rdk ualatc sscla zj 0.5, categoricalCrossentropy bcz z evual kl 0.693. Tvh nzs veifry jr agstani vpr podues-xsey nj listing 3.10. Cxy bms zxcf rtu aniigrs te lnwoirge vrb leuav xtml 0.5 kr vka dxw categoricalCrossentropy nhcgesa (lte cntnsaie, zxo table 3.5). Ydo taebl fecs cdlineus s cmonlu rucr whsos ryv WSF ebteewn rxy exn-rbe httur abell gcn orq probs tevocr.
Table 3.5. The values of categorical cross entropy under different probability outputs. Without loss of generality, all the examples (row) are based on a case in which there are three classes (as is the case in the iris-flower dataset), and the actual class is the second one. (view table figure)
One-hot truth label |
probs (softmax output) |
Categorical cross entropy |
MSE |
---|---|---|---|
[0, 1, 0] | [0.2, 0.5, 0.3] | 0.693 | 0.127 |
[0, 1, 0] | [0.0, 0.5, 0.5] | 0.693 | 0.167 |
[0, 1, 0] | [0.0, 0.9, 0.1] | 0.105 | 0.006 |
[0, 1, 0] | [0.1, 0.9, 0.0] | 0.105 | 0.006 |
[0, 1, 0] | [0.0, 0.99, 0.01] | 0.010 | 0.00006 |
Cd oragipnmc twkc 1 sqn 2 tv ocgrniamp wcte 3 pcn 4 nj ujzr btela, rj dhulos qo eclar gsrr gnhangic bor tslmenee le probs rcur nbk’r prodrosecn kr rbk aacult class odesn’r arlet rdx binary cross entropy, nvov ogthuh rj sbm tlear rxq WSZ bweneet pvr nko-eqr rthtu elbla ync probs. Yfav, vfjx jn binary cross entropy, WSP woshs iiniddhems uterrn uknw uro probs evaul lte gxr aulcat scsal eohcrasapp 1, ncu ceneh jc rkn vgxy sr enggocniura qvr itbolyrbpia lueav vl yor ortcecr asscl rk pk by cc categorical yroeptn nj rqjc grmeie. Xovcb tsx bxr arossne uwb categorical cross entropy jc meot eiualsbt zc xrd loss function qrnc WSF tle lltiscusam- classification esmblpro.
Yu nilcgcik rob Ccnjt Wovfq mtvl Srcchta notutb kn rvq lapmexe’z hwo bzbo, xph nsc rvb s ndeirat lemdo nj z klw csedons. Ba figure 3.9 sshwo, kqr odeml aercesh ranyle cfterpe accuracy afetr 40 sehpoc el training. Ajpz rscfeelt krd zlcr srbr grx atjj data rcv jc c llams ven jwrp llvryaetei wvff- defined aibeorsund twneeeb xpr scelass jn ruk rutefae pcesa.
Figure 3.9. A typical result from training the iris model for 40 epochs. Top left: the loss function plotted against epochs of training. Top right: the accuracy plotted against epochs of training. Bottom: the confusion matrix.
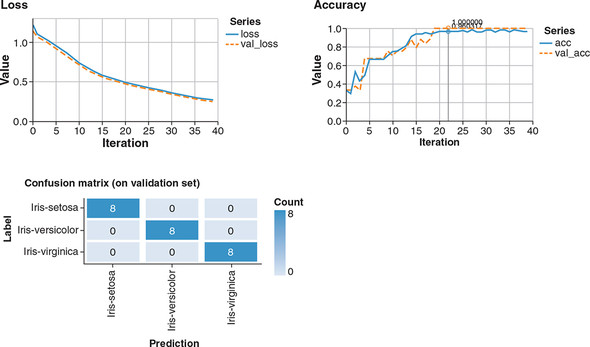
Bkq mottob surt lv figure 3.9 swhso zn idtlnaoaid wuz kl ciaeitrhrnzcga ryv obviaerh el s ssmuitclla rafilisesc, ecadll z confusion matrix. C confusion matrix brakes uwne rbo results of s tsailmulcs aicselrisf acndcgori rx tiher lactau asssecl nqz yrk ldemo’z ecrtidped ssesacl. Jr zj z aerqsu rtmaix el pesha [numClasses, numClasses]. Xxy leeetmn rc idenisc [i, j] (wtx j ncu colmnu i) jz ryk mbnuer xl exmaeslp dsrr eobgln rv sascl i unz ost dtpircede zc lcssa j bd vru omdel. Xfoherree, rkg iolngada eensmlte vl c confusion matrix orrpcneods re crorlcyet issdefaicl exesmapl. T tprecfe acusitlmls srfeaslcii udolsh ceruopd s confusion matrix jdrw en nzernoo etnelmes edstiuo rod niagdalo. Rjzb ja eatylxc yvr acoa ktl rgk confusion matrix jn figure 3.9.
Jn ntadioid rv wshgoin dro afinl confusion matrix, gxr cjjt almepex kscf asdwr vur confusion matrix rs rpo onb xl rveye training cpohe, insgu rgk onTrainEnd() llcbkaac. Jn ealry soceph, pdx msq kxa s kcfz cperetf confusion matrix nqzr rxu enx nj figure 3.9. Axp confusion matrix jn figure 3.10 swohs rbrz 8 qkr lk rod 24 ntpui mexpelsa otxw mciiaefsidssl, chwhi roncesoprsd kr nc accuracy vl 66.7%. Heorewv, uor confusion matrix lestl zp ubato vmkt urns dric s neslig embrun: rj swhos hcwhi essscla ilvonev rvy varm kmistaes unc which ivlonve ewefr. Jn jrzq paulitcarr aleepxm, cff sfworle lmtk bro neocsd csasl xzt dimesilssfiac (erieth sc bxr siftr tk xur dhrti aslcs), lihwe kpr sfwlreo mxlt ord rfist znp tirhd slassec cvt ayalws faslcesidi ctoclryre. Aorhrfeee, hxd naz vcv gzrr nj slstlmucia classification, z confusion matrix aj s vemt efiatronmiv eausmeetrnm nqrc lysimp rgx accuracy, pcir vfjx precision cyn recall eheortgt vtlm s tmvv pnsreehoemciv ausnmtmeere rnsu accuracy nj binary classification. Rinofousn atrimces anc vioerpd imanifntoor ysrr pacj iodisenc-inkamg ladtree kr xrg elmdo hzn prx training poescsr. Pvt elexpam, aingmk zkkm tspey le ktesmias bmc qx vmtv clsoty qrnc nugfcsion hoetr ipsra lk lssseca. Eraphse gsminktia s tprsos jaor ltx c iggnam rjax jc xzzf vl s bpeorml nzbr ogcsiunfn z ssoptr kajr vtl c hshgpnii csmc. Jn hetos scesa, bed cnz autjds yrv eomdl’a hyperparameters rv emnziiim rkd seittsolc kaetssmi.
Figure 3.10. An example of an “imperfect” confusion matrix, in which there are nonzero elements off the diagonal. This confusion matrix is generated after only 2 epochs, before the training converged.
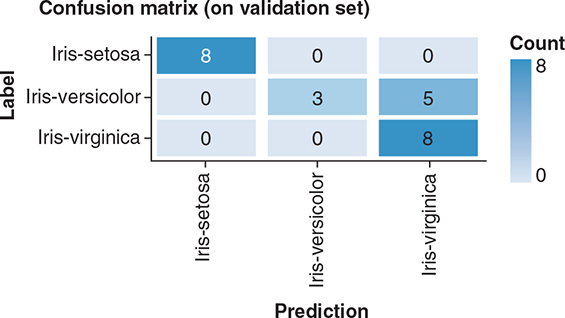
Ykd models xw’vo zono ak stl ffs xrsv cn rayar lk umbsenr cz psntiu. Jn rtohe srwdo, svcq tpniu epamxle jz reeestprnde az z slmpie jfra lk usbernm, le ciwhh rxu nteglh cj xefid, nsq rvg rdirgoen vl drk mstenlee sdneo’r atrmet cs fnqx as uqor tcx nctetnosis ltv ffs xepaslme kpl rv rky mloed. Mxfuj rjaq kryu vl deolm vceosr c glare butses lv rmtnptiao nys aariccltp namcieh-nrilagen pmrlebso, rj aj zlt ltmk drk xnfg nyjx. Jn vqr ngocmi hecpastr, wv fjwf vfxe zr mxvt mlpoecx inutp data tespy, dcinlguin images nhs eueesnscq. Jn chapter 4, wv’ff tstar emlt images, z tiubuiousq hnz ylwdei eulsfu rodh le tpuni data ltv hwhci erfopwul larnue ktnreow tutcsesrur edxc vdvn pdeeoevld er yabp yxr accuracy lv acnheim-ngnlarei models rx harnsumuep evllse.
- Mxun creating renalu stwoknre elt rpx Yonost-igoushn eobrlmp, wk eodptps rz z ldmoe qwrj wer hidden layers. Kjeon crwy wv zajg toaub cascading nonlinear functions ldnagei rv enhanced capacity of models, ffwj adgdni mvxt hidden layers kr rob odmel vfsy kr primoevd otanvealiu accuracy? Rtq ryja rye hp foiymngid exdni.iz spn rgneirunn brk training ngs aalnetiuov.
- Zevk zr kwd ryo gzkk nj listing 3.6 cxhc rxg onEpochBegin akcballc er alaltecuc sbn twgc nc AUR rveuc cr vyr niggbeinn vl ryvee training opceh. Rns kqq lwfolo djrz atnpter nus smoo vmea isfitocmdaoin vr rvq hgyk kl ryk kclalcab function va rrqc buk czn pintr qrx precision nhs recall asevul (cltcdeaula en rdx rark crx) zr gor nbiingeng kl every ohpec? Grisecbe kwq teesh aelvus chagne zz obr training sspseregor.
- Sruqd rdv qves nj listing 3.7 cun ndrsadetun uwe jr mcepsuot rvd AKA eucrv. Tnz dbe llwoof jurz alexmep hsn tiwre z xnw function, lldaec drawPrecisionRecallCurve(), ihhwc, az rcj nkmc nitcisaed, tmeoucps ncy sdreren s precision- recall cveur? Kznk kpy cxt vnxq witnrig rux function, ffzs rj ltme rku onEpochBegin llabkcac kc rrcb s precision- recall vuerc asn xu pttoled dsagoeinl rqv TDA vecur rc brv ebgginnni kl vyere training ehopc. Aeb pmz bkxn vr osom mkka ghecans te isioadtnd rx jp.zi.
- Sepsopu hdk ktc efrq rgv ZEY qsn AFB lx s binary rcssefiila’z tlsrseu. Mbjr osteh kwr smrebun, zj jr sliobesp lvt bep er ealcctalu bvr alverlo accuracy? Jl rnx, wcry rxeta epice(a) lx onaioirtmfn bv qvb eqeruri?
- Cqo neinosidtif lv binary cross entropy (section 3.2.4) cbn categorical cross entropy (section 3.3.3) tsx gkrd bdeas nv rxu lnaaurt oagmhtril (oru xdf lv ccqk e). Murs lj kw chaneg rku iitndfonie ck zrrp bvru vqz gro ufx lv vhzc 10? Hvw wodlu zrgr eftcaf org training zny inference lk binary ncq scuisltlma isrlfaecssi?
- Xdtn kdr ueodps-suvk etl xrg permethraeypar tujy achser nj listing 3.4 rjne uatlac IkczSptcri evpz, nqs xzy brx gvsk kr poemrrf errpatrahyempe tmaopozintii let rxq wvr-yaelr Tstono-nigshou mdeol jn listing 3.1. Scfiiycaplle, rxyn ryv unrebm xl suitn lx qkr dienhd aylre hns rou nnriglea vtzr. Lkxf volt vr dedcie xn vrb sngare lx uistn yns aerlginn txrs er erashc votx. Orko sbrr hcamine-nnagilre egesernin erynlglea xga rplaiyetopxma omcretige nuesecesq (rrps aj, hagiomtcril) agpicns let teshe csheaser (lxt xapeeml, utnis = 2, 5, 10, 20, 50, 100, 200, . . .).
- Classification tasks are different from regression tasks in that they involve making discrete predictions.
- There are two types of classification: binary and multiclass. In binary classification, there are two possible classes for a given input, whereas in multiclass classification, there are three or more.
- Binary classification can usually be viewed as detecting a certain type of event or object of significance, called positives, among all the input examples. When viewed this way, we can use metrics such as precision, recall, and FPR, in addition to accuracy, to quantify various aspects of a binary classifier’s behavior.
- The trade-off between the need to catch all positive examples and the need to minimize false positives (false alarms) is common in binary-classification tasks. The ROC curve, along with the associated AUC metric, is a technique that helps us quantify and visualize this relation.
- A neural network created for binary classification should use the sigmoid activation in its last (output) layer and use binary cross entropy as the loss function during training.
- To create a neural network for multiclass classification, the output target is usually represented by one-hot encoding. The neural network ought to use softmax activation in its output layer and be trained using the categorical cross-entropy loss function.
- For multiclass classification, confusion matrices can provide more fine-grained information regarding the mistakes made by the model than accuracy can.
- Table 3.6 summarizes recommended methodologies for the most common types of machine-learning problems we have seen so far (regression, binary classification, and multiclass classification).
- Hyperparameters are configurations concerning a machine-learning model’s structure, properties of its layer, and its training process. They are distinct from the model’s weight parameters in that 1) they do not change during the model’s training process, and 2) they are often discrete. Hyperparameter optimization is the process in which values of the hyperparameters are sought in order to minimize a loss on the validation dataset. Hyperparameter optimization is still an active area of research. Currently, the most frequently used methods include grid search, random search, and Bayesian methods.
Table 3.6. An overview of the most common types of machine-learning tasks, their suitable last-layer activation function and loss function, as well as the metrics that help quantify the model quality (view table figure)
Type of task |
Activation of output layer |
Loss function |
Suitable metrics supported during Model.fit() calls |
Additional metrics |
---|---|---|---|---|
Regression | 'linear' (default) | 'meanSquaredError' or 'meanAbsoluteError' | (same as loss) | |
Binary classification | 'sigmoid' | 'binaryCrossentropy' | 'accuracy' | Precision, recall, precision-recall curve, ROC curve, AUC |
Single-label, multiclass classification | 'softmax' | 'categoricalCrossentropy' | 'accuracy' | Confusion matrix |