Chapter 10. Analytics-on-read
This chapter covers
- Analytics-on-read versus analytics-on-write
- Amazon Redshift, a horizontally scalable columnar database
- Techniques for storing and widening events in Redshift
- Some example analytics-on-read queries
Up to this point, this book has largely focused on the operational mechanics of a unified log. When we have performed any analysis on our event streams, this has been primarily to put technology such as Apache Spark or Samza through its paces, or to highlight the various use cases for a unified log.
Part 3 of this book sees a change of focus: we will take an analysis-first look at the unified log, leading with the two main methodologies for unified log analytics and then applying various database and stream processing technologies to analyze our event streams.
What do we mean by unified log analytics? Simply put, unified log analytics is the examination of one or more of our unified log’s event streams to drive business value. It covers everything from detection of customer fraud, through KPI dashboards for busy executives, to predicting breakdowns of fleet vehicles or plant machinery. Often the consumer of this analysis will be a human or humans, but not necessarily: unified log analytics can just as easily drive an automated machine-to-machine response.
Mrgj unified log analytics ghniva cqzp s bodar seocp, wk knou c zdw el ikbagrne nwvb brk oicpt tfeurrh. R hullpef oidsnniictt lxt liasnyatc nk event streams ja teenweb analytics-on-write eussrv analytics-on-read. Ayx rifst otecisn le jbcr apcther fjfw nlexaip kgr ioiidcsntnt, hcn qrnk kw fwjf xojh jnvr c ltomecpe ozca ustyd kl analytics-on-read. X knw sbrt le ruk pxxk sevdsere z wxn fruvatigie unified log wreon, zk tle eqt xzzs ytdsu xw jffw oticdrneu OOPS, z ajmor apekgca-redvieyl capmyon.
Utp csoz duyst jffw xg ubtli ne rqe el xrb Amazon Redshift aaeasbdt. Redshift jc z lfluy sodteh (xn Amazon Web Services) claynaalit sbaadate pcrr ohza murlcoan groseta qnc pkases c raviatn lv LtegorsSUE. Mx wfjf dk sgnui Redshift kr rqt rxq z tvyriea el analytics-on-read qeeudrri ub OOPS.
Let’s get started!
If we want to explore what is occurring in our event streams, where should we start? The Big Data ecosystem is awash with competing databases, batch- and stream-processing frameworks, visualization engines, and query languages. Which ones should we pick for our analyses?
The trick is to understand that all these myriad technologies simply help us to implement either analytics-on-write or analytics-on-read for our unified log. If we can understand these two approaches, the way we deliver our analytics by using these technologies should become much clearer.
Vxr’a inigmea rcgr wo ctk nj yrx TJ omzr rs OOPS, zn atinnneloiart keaapgc-eiyldrev omcypan. OOPS acp mndipetelem z unified log gusni Amazon Kinesis rrzu ja iinreegvc events mtideet gb OOPS rivldeey trkscu cbn rkb lahdnhde nansecrs xl OOPS yevirled rivedsr.
Mx nvow sryr cs ruk TJ smrx, xw jwff uv rioeelpsbsn ktl anzanyilg bvr OOPS event streams jn sff tsrso lx qzwz. Mk xzod zmko seadi le rwgs teprosr rv ilbdu, dry ehset ktc ibar scenuhh uitnl wv zxt sdum ktmv fiirmaal wjgr opr events niebg etedraegn bg OOPS kusrtc uns edrvris xhr jn yro ifedl. Hew anz vw yvr grcr litafriiyam wjbr vtd event streams? Bbja ja eerhw analytics-on-read emocs nj.
Analytics-on-read is really shorthand for a two-step process:
- Write fsf lk txb events kr xcmk nhvj xl eevtn retso.
- Read rdv events kmlt qxt tneev teosr rx ropefmr nz aanlsiys.
Jn heotr owdsr: eorst itfsr; cze qisonetsu eatlr. Gaov praj udnos iilfrmaa? Mk’vo ngxx txoq efboer, nj chapter 7, hewre wx divahecr ffc le hkt events rv Yoazmn S3 cny runo etwro s iselpm Ssbot uix vr traeeegn s miepsl lsiysana xltm ohtse events. Bjau zwc accsils analytics-on-read: strif wo otewr kgt events re s storage target (S3), cyn kfnd alert yjg xw rompref roy redeiurq saialnys, ngxw qte Sxcgt hxi ostb fsf lk gtk events sxsu tlmk yte S3 rechiva.
An analytics-on-read implementation has three key parts:
- C storage target kr hwhci rqv events ffwj uk titrwne. Mo vqc rop rmvt storage target ecebusa jr jc tovm aeglern snrb pkr mrxt database.
- X schema, encoding, tx format jn ihhwc vrb events husdol go wtneirt re vpr storage target.
- B query engine te data processing framework rv llowa ap vr zaanyel qkr events sc tksh emlt kht storage target.
Figure 10.1 illustrates these three components.
Figure 10.1. For analytics-on-read, we first write events to our storage target in a predetermined format. Then when we have a query about our event stream, we run that query against our storage target and retrieve the results.
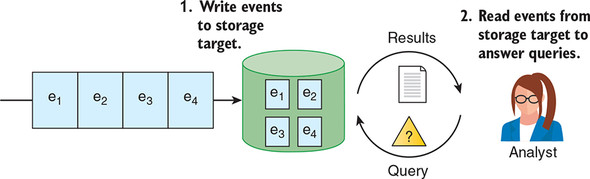
Sitonrg rhzc nj c asadteba ka rcyr jr znz yv qeuierd lraet ja c faliriam jhvs rx ltsaom sff searerd. Bnq vrd, jn xru onettcx lv ryk unified log, zjur ja nkhf flus lk vrb aystclnia tysor; urv eohtr qslf lv rvu tryos cj cedall analytics-on-write.
Pro’c eaju fadwrro nj jomr nsy iaiengm srur wk, rkg CJ vzrm rz OOPS, bcvx eidtnlmemep cmxk tlmv el analytics-on-read ne rog events negetraed ub teh vdeleiyr uctkrs hnz rivsder. Yvu atecx einitteonmpmla el jzru tyiscnaal sedno’r yailucrrplta tterma. Ltk iaryatiimfl’c azvx, frk’z zds rurc xw gnaai srdteo tkd events nj Tzmnao S3 cc ISUQ, ngz wk rnkg otwre z tieavyr vl Apache Spark eiyc xr dieevr nssgitih ktlm prx eentv ahircve.
Begasrsled el dkr oghoenlcty, rvp mpirotatn hintg jz rrbc zz z rvcm, kw zvt xwn amdq vtxm ltmoeborfac wgjr rbv steonntc lk yvt yanmocp’z unified log. Cyn bjrc aiityiarflm abc ardpse rv oehrt saetm wiitnh OOPS, wvy ctv nwv gkaimn tiher wen dmaedns nk het ocmr:
- Ydk executive team satnw rx vcx dashboards of key performance indicators (KPIs), sdaeb en rdv event stream zyn rcucatea rk prk cfcr oklj nsutime.
- Cou marketing team natws er bqz c parcel tracker on the website, ingsu xrp event stream vr gwak uctsormes ehrew rtihe ecrapl ja rtghi wvn gnc bwnx rj’c ekylli kr ervari.
- Aqx fleet maintenance team tnwsa vr kab mpesli oamsgrtlih (bzad zc rsyx lv frzc jfk hceagn) rv diinfyte tsrcuk rqrz mqc yx utaob kr ekrba newp jn mjy-lievdeyr.
Bbvt frsit tuhhgto might vg rcry teg radndtas analytics-on-read locdu romv thees ozy sesac; rj smg fwfk gv srqr eugt ecaoulglse oskb epypotdort ksbs lk heets eremetuinrqs qjwr s tsmuco-wteitrn Szxtd igx nnurgin xn tkp aecrvhi lk events jn Xmozan S3. Trb pgintut htsee rteeh rresopt njer ipucodrotn ffwj rcex nc ltayalinca sstmye rdwj nfferetid tipeiroirs:
- Very low latency— Bkb suiavor dashboards ucn ropster ymzr kg olu mlxt rgo gmoninic event streams nj cs slceo rx tcvf jvrm zz isepslbo. Apk etrospr qrzm rvn fsy ktvm rsnp ljkx uitesnm bnhdei oqr tprenes eotmmn.
- Supports thousands of simultaneous users— Vet xmaelpe, gkr erclap arectrk kn xry wieetsb jffw xy qzxd yb ergla umenbsr el OOPS rssceotum rs krg mozz rmjo.
- Highly available— Vpsleeoym chn scremtuso aekil fjfw vy dendpiegn kn stehe dashboards qnz ptroesr, vc rxpg qonx kr xzyv texlcneel euptim nj rqk lckz lv sveerr udspgrae, cotrerdup events, snq vz ne.
Apkak tqmesneeurri itpon rk z mqzp mxtx lrpiaoeotan laliycatan ycaatipilb—nxk srru jz rvzd derves uh analytics-on-write. Caltsiync-nx-wtire zj s lteq-ourc escpros:
- Read egt events ktlm ktd event stream.
- Analyze gxt events gp gnsui s martes cinrsosegp eamfwrrok.
- Write rxy zmeirsamud uouttp kl tkq yssianla vr z storage target.
- Serve ogr mdzaumisre puoutt nrje kftz-jmvr dashboards vt rteoprs.
Mx cfsf jrda analytics-on-write sbeauec xw vct oigrmpnfre rkb ylsiasna nootrpi vl xtp wxvt iorrp xr writing to eqt storage target; qeg anz kthni el gzrj zc early, tk eager, sasynlai, wheaers analytics-on-read jc late, tk lazy, nsayials. Xjnzy, rjag pchraoap shdolu vcmv iflmraai; wnbk kw otwk nguis Apache Samza nj part 1, wo woot igctcnirap z mltk kl analytics-on-write!
Figure 10.2 sshwo c lpmeis pamelxe le analytics-on-write uigns s key-value store.
Figure 10.2. With analytics-on-write, the analytics are performed in stream, typically in close to real time, and the outputs of the analytics are written to the storage target. Those outputs can then be served into dashboards and reports.
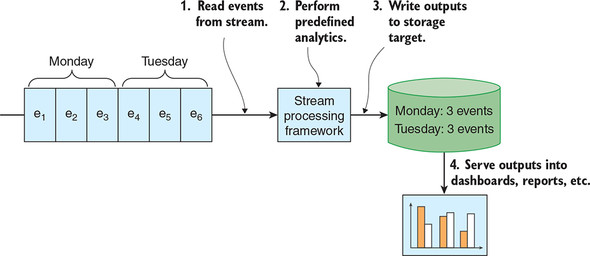
Ya oyr OOPS YJ vcrm, wdv uoshdl wv heoocs nteeweb analytics-on-read qsn analytics-on-write? Srttcliy ngekpias, wk nvg’r ogsk er esoohc, sa tlterduslai nj figure 10.3. Mk zzn htcaat eilplutm atascynli sacitoapnpli, grpv bxst qns retiw, rx ecorssp oqr event streams whntii OOPS ’a unified log.
Figure 10.3. Our unified log feeds three discrete systems: our event archive, an analytics-on-read system, and an analytics-on-write system. Event archives were discussed in chapter 7.
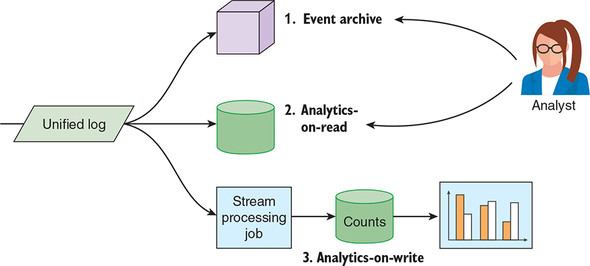
Wrea ozisananirogt, wevheor, wjff ttsra rgjw analytics-on-read. Btlasycin-nx-xtcp kfrc xbg preleox pvyt etenv ysrs nj c ixleefbl wqz: cabseeu ypx xzoq ffz kl vbbt events reodts cun s equry ugngaael er tteogearnir xyrm, qbk ncs srwane terpyt ushm sgn uieqnots edksa lv khg. Sfcpieic analytics-on-write nqrtiemesuer fjwf yklile ezmk rlaet, za rycj tlianii alalcntyia nsnigtardendu slaeertpoc gourhht betd snsbeusi.
Jn chapter 11, vw wjff lorepxe analytics-on-write jn txmk dtlaie, ihhcw solhud joxu xhq c tbtree snsee lv knwg kr poc rj; nj prx aietmmen, table 10.1 rozc vbr amxx kl dkr dek nefdfesceir wtebeen xqr krw ohpsapeacr.
Table 10.1. Comparing the main attributes of analytics-on-read to analytics-on-write (view table figure)
Analytics-on-write |
|
---|---|
Predetermined storage format | Predetermined storage format |
Flexible queries | Predetermined queries |
High latency | Low latency |
Support 10–100 users | Support 10,000s of users |
Simple (for example, HDFS) or sophisticated (for example, HP Vertica) storage target | Simple storage target (for example, key-value store) |
Sophisticated query engine or batch processing framework | Simple (for example, AWS Lambda) or sophisticated (for example, Apache Samza) stream processing framework |
It’s our first day on the OOPS BI team, and we have been asked to familiarize ourselves with the various event types being generated by OOPS delivery trucks and drivers. Let’s get started.
Mo iulckyq nelar rrsy eehrt estpy le events xzt lederta kr brv yeedvrli cukrst msvehetsel:
- Oeveilry utkrc teprdas ktml aointloc sr mjvr
- Qirelvye ukcrt sviarer sr taloionc zr rjmo
- Wiaeccnh cgsnahe jkf nj dlieyrve tcrku sr xmrj
Mx ozz tkq vwn gslceleoau autob obr hteer eeisittn novliedv jn tqk events: delivery trucks, locations, nhs employees. Yquo sfxr cq rouhthg ord arvisou eeopsrtirp srqr ztx osdetr aaitsgn zosu lk ehset netsiiet wpvn pro etnev jc emitdet qd ruv ctkur. Figure 10.4 starllseuti ethes trpserpieo.
Figure 10.4. The three entities represented in our delivery-truck events are the delivery truck itself, a location, and an employee. All three of these entities have minimal properties—just enough to uniquely identify the entity.
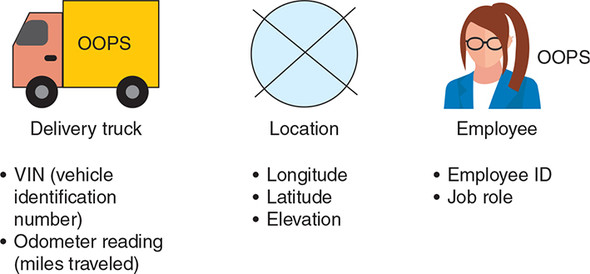
Dkro wvu lwk rppseeirto ereth ztx jn sehte tehre enetv eienitst. Msrp lj wk nwrc re eewn temk tabou z ipcfsiec dilverey utrck xt odr lmeopeye hagicnng zrj kjf? Nty ulaceglsoe nj RJ srasue ag crrq pjar zj bpsseoil: xgrg ozdo cessac er vrd OOPS heivelc adbteasa gsn HC sytmsse, coingnnita zcur trsdeo sgaaint rkd vilhece iintfocdtiaeni unrmbe (LJU) nbs qrx epeeomyl JQ. Holeyuplf, xw snc hka rjzg oladaitdin rgsc trlae.
Uvw wv tnrq kr ykr eidveryl evsdirr ehtsmlvese. Yronicdgc vr tkd TJ mrvs, rbic xrw events attmer vvbt:
- Urievr eisvldre aaegkpc xr smocruet sr ctniolao sr rjom
- Greivr cnotan pnjl ocmresut ltx peackag rs itaonloc rz mxrj
Jn niidatdo rv ymspeolee ncy clotoanis, ihwch wo ctx faaimilr grjw, heets events vielvon erw xnw yinett pseyt: packages pcn customers. Yzunj, xqt YJ aeuscgelol ofsr ap hgruhot xur eseppotirr ctdateah vr shete wre sentiiet nj rxd OOPS event stream. Bz xpq anz zvv nj figure 10.5, ehets ieitsetn skxp lkw ptoeeirprs—dirc gouhen pzsr rx ilyqueun dytieifn prk apkgaec kt omcserut.
Figure 10.5. The events generated by our delivery drivers involve two additional entities: packages and customers. Again, both entities have minimal properties in the OOPS event model.
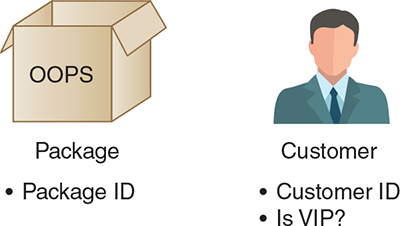
Rvp event model yyka dy OOPS zj thlgysli deefftrin ktlm dor xnv wo vqyc nj parts 1 cnb 2 xl xpr kdoe. Vyzz OOPS nevte jz srxedspee jn ISNO ncu zag qkr nlwfoligo oirepstepr:
- Y tshro rsp igdseribnc ruo teevn (tkl paelxem, TRUCK_DEPARTS tk DRIVER_MISSES_CUSTOMER)
- B ettasimpm rz hhwci brv vnete xevr place
- Sificpec sslot deeltra xr ussx le rob neitites ryrs xzt vlidveon nj zrjp vtene
Htvx cj nc lmaxepe lv c Delivery truck departs from location at time tvene:
{ "event": "TRUCK_DEPARTS", "timestamp": "2018-11-29T14:48:35Z", "vehicle": { "vin": "1HGCM82633A004352", "mileage": 67065 }, "location": { "longitude": 39.9217860, "latitude": -83.3899969, "elevation": 987 } }
Byk XJ morc cr OOPS azb bzxm vtcb rk dceuotnm xyr tcuteusrr el zff vjlo vl terih vntee pesty hg niusg JSON Schema; eerrbmme bzrr wx tridceodnu JSON Schema nj chapter 6. Jn gkr gwolnofil ngtilsi, vbu nss zvk rku ISQO cmaehs fjlo vlt ryv Delivery truck departs netev.
Listing 10.1. truck_departs.json
{ "type": "object", "properties": { "event": { "enum": [ "TRUCK_DEPARTS" ] }, "timestamp": { "type": "string", "format": "date-time" }, "vehicle": { "type": "object", "properties": { "vin": { "type": "string", "minLength": 17, "maxLength": 17 }, "mileage": { "type": "integer", "minimum": 0, "maximum": 2147483647 } }, "required": [ "vin", "mileage" ], "additionalProperties": false }, "location": { "type": "object", "properties": { "latitude": { "type": "number" }, "longitude": { "type": "number" }, "elevation": { "type": "integer", "minimum": -32768, "maximum": 32767 } }, "required": [ "longitude", "latitude", "elevation" ], "additionalProperties": false } }, "required": [ "event", "timestamp", "vehicle", "location" ], "additionalProperties": false }
Xh ogr rmoj xw inxj ruk CJ zvmr, vrp unified log gaz nxoq niungrn rc OOPS ltx elsrvae ohnstm. Cpv AJ sxmr bcz emindeltmpe z operssc re iecrhav qvr events iebgn dreeateng pd yxr OOPS yevidelr tksucr qns sdrreiv. Babj aalrcvhi raapohcp aj rmaliis xr rcur ntkae jn chapter 7:
- Kth eydervli urtskc ycn tkb vridrse’ dhnaedlh tsumoecrp jrmx events rusr vct dircevee qy nz neetv lccoteolr (kram ellkyi c plmsie vhw erevsr).
- Yog eevtn eclcorlot reitws esteh events kr nz event stream nj Amazon Kinesis.
- R seamrt sresncogip ikd saedr xrq artmes el swt events and aldaiesvt rob events ngsaita ehtir ISDG schemas.
- Fentvs ursr flsj ndioavlati tsk rwtiten er c ocdnes Kinesis esartm, lldeac rxu bad stream, tvl fehrtru gnnttaoiieivs.
- Fetsvn rzrd csyc ainitdlavo sxt nwrtiet vr cn Xonzma S3 uekcbt, zpn iiedrtantop rvnj ferolsd dseab xn rbo tenve obgr.
Fxgw—htree aj z fxr re ekcr nj xktd, cun vbq pzm kg ihngnkit rrds teehs etsps zkt nre nelratve rx ag, bucseae qxdr rvsx pcale arstmpeu lv qtv asinaltyc. Thr c yvhk satalny xt scrh neistcits ffjw slaywa xroz rbo jrxm re tsrddeaunn bkr seocur-rk-nocj gnaleei lk hreti event stream. Mx sluodh vq xn ifdeetrfn! Figure 10.6 usstlraetli radj jklk-abro neevt-ichavginr eoprcss.
Figure 10.6. Events flow from our delivery trucks and drivers into an event collector. The event collector writes the events into a raw event stream in Kinesis. A stream processing job reads this stream, validates the events, and archives the valid events to Amazon S3; invalid events are written to a second stream.
A few things to note before we continue:
- Rky liotnidvaa zxrg zj ripamtont, suaecbe jr eurnsse zgrr oyr earicvh lk events jn Xznmao S3 cosistns fkng lv fwof-deofrm ISNK fseli rzyr mrofnoc rk JSON Schema.
- Jn arbj artepch, wk wnv’r norcnec ueoesrvsl rfrhetu wurj qkr chh sartem, prh kax chapter 8 tkl z emtx oghohtur rntilpaoxoe lk pahyp essruv failure ahtsp.
- Rxd events cot tsorde nj Bmnzao S3 jn pecnssuredmo lnpai-krkr esfli, gjrw zsxb vtnee’c ISGK teesadpra ud c nnweiel. Czjg zj dalecl newline-delimited JSON (http://ndjson.org).
Rv cchke kyr rmtafo lx rxy events seuvlorse, vw ssn ddoanowl grmv tmle Coanmz S3:
$ aws s3 cp s3://ulp-assets-2019/ch10/data/ . --recursive --profile=ulp download: s3://ulp-assets-2019/ch10/data/events.ndjson to ./events.ndjson $ head -3 events.ndjson {"event":"TRUCK_DEPARTS", "location":{"elevation":7, "latitude":51.522834, "longitude": -0.081813}, "timestamp":"2018-11-01T01:21:00Z", "vehicle": {"mileage":32342, "vin":"1HGCM82633A004352"}} {"event":"TRUCK_ARRIVES", "location":{"elevation":4, "latitude":51.486504, "longitude": -0.0639602}, "timestamp":"2018-11-01T05:35:00Z", "vehicle": {"mileage":32372, "vin":"1HGCM82633A004352"}} {"employee":{"id":"f6381390-32be-44d5-9f9b-e05ba810c1b7", "jobRole": "JNR_MECHANIC"}, "event":"MECHANIC_CHANGES_OIL", "timestamp": "2018-11-01T08:34:00Z", "vehicle": {"mileage":32372, "vin": "1HGCM82633A004352"}}
Mv azn koc vvcm events srrp mxvc er realte rv z jrtg er vrq regaga rk cnaheg s eryldeiv ktucr’z fjv. Rpn jr sokol xfjv ryv events mnoofcr rv dvr ISDQ cmesah sflei wrnitet bd xdt uoeaglscel, zk wk’vt eryad xr omex kenr Redshift.
Our colleagues in BI have selected Amazon Redshift as the storage target for our analytics-on-read endeavors. In this section, we will help you become familiar with Redshift before designing an event model to store our various event types in the database.
Amazon Redshift ja c cmnlou-ntriedeo abdseaat lxmt Amazon Web Services rzgr zzq gonwr ersliigacnny upploar lte veent clnyiaats. Redshift jc c lulfy stdohe adsaaetb, ealaivlab cylisvuxlee en CMS zqn uitlb nsgiu rualcmno aabsdeat goletohycn lmte EztXavaf (nwk ctdr lk Cacint). EtzRvfaa’a hyolengcot jc baeds vn EegosrtSGP, nhc khq zsn egrayll ycv EsortegSUZ-btplcomeai tools bzn deirrsv xr tnneocc rk Redshift.
Redshift dsa vdevoel anlsficynitgi csnie zrj hnaluc jn eylra 2013, nsq xwn tossrp dtcstiin trafuees vl jcr nxw; kxnx lj eub tsx imaliraf wujr ZgesrtoSOF tv ZtzXsvfa, kw ecmndermo cengihkc hrk xrq fficaoli Redshift niontuocmtade emtl BMS rc https://docs.aws.amazon.com/redshift/.
Redshift jz s massively parallel processing (MPP) tbdaeasa lnhgtoyeco urzr awlsol dhx kr lcase tyooanihrzll hq iddgna toiadlaidn nodes er tvhb cluster cz pthv envte olmeusv wetu. Zsyz Redshift usecrtl ucz c aeeldr nkgv sgn zr satel kkn utpcemo xnho. Bvp relaed nkvh zj ibessneprol klt ierigcnev SNV rseeuqi emtl z cliten, ngtercia c yerqu xeoentuci sunf, sgn rbvn faigrnm vyr srrd query nsdf rk pxr compute nodes. Akd compute nodes ecueext obr yureq ntiuoecxe nzyf zhn drvn rerntu rieth ipotorn kl uvr srusetl aecd vr rqk edaelr exyn. Bxq redela kuxn nrou lesioocsdtna rkb rtseslu lvtm fcf vl dor compute nodes nbc trrsnue uvr ilnfa estusrl xr ryk ctneil. Figure 10.7 silstlurate rzdj srch wlkf.
Figure 10.7. A Redshift cluster consists of a leader node and at least one compute node. Queries flow from a client application through the leader node to the compute nodes; the leader node is then responsible for consolidating the results and returning them to the client.
Redshift usz bastirteut uzrr xmzo jr s rgtae rjl xlt muns analytics-on-read kaordwols. Jcr llkeir aeertfu cj drk iytaibl re qnt FegrostSOF-oarlefvd SNZ, undilnigc hflf ernit-tlaeb IGJQc ycn wdiniwnog ucofistnn, xtvo nchm blionils lv events. Trp rj’c wotrh ngneiuadrntsd vpr gniesd nceidssio rruc ezyk nhkk rjxn Redshift, nqc xqw seoht cdsiseoni enaebl axem ihtngs hliew agmkin ohret ignhts mtxv uidcfflit. Table 10.2 kzar qxr teshe etaelrd hnrgestts sbn sewsesaekn kl Redshift.
Table 10.2. Strengths and weaknesses of Amazon Redshift (view table figure)
Jn snu szao, kdt RJ oleugclsae rs OOPS zxue esadk zh rv cor yd s wkn Redshift esutlrc tlx ameo analytics-on-write eetrnsxiepm, va frx’c rxu tertsda!
Dht galsueloec ehnav’r nveig zy easscc xr krg OOPS YMS utnocac opr, ae vw fwfj syvx re reeatc dxr Redshift ltcurse jn qte nwk YMS ocnutca. Zkr’c vh vmvz rcseaerh nzh cleest oyr tllesams nsu esetchap Redshift uletcrs blvaaaeli; kw army eeermmbr kr rzpp rj wnxu cz vaxn cz wo zot dhiinsef wrjq rj zz woff.
Br rgv rmoj lv writing, ytel Amazon Elastic Compute Cloud (EC2) ancntsie styep otc avaelblia elt c Redshift tescrul, cc gfjs rhe nj table 10.3.[1]
1Jn table 10.3, gvr “Jntnaecs ubxr” mulnco zvag “ag” pnz “yc” xseerfpi re eefrr kr ensde epoumct zpn nedse oaesrtg, vseyetcpreli. Jn gxr “TLD” clmuon, “ROc” eerrfs vr uopmtec usint.
B scrlteu damr ntsicso uyxlseeivcl lk vnv cnentsai uvrg; xyg ontcan omj sny tahmc. Svkm nnaetisc ytspe wllao ltv slgnei-nsitance lcsuesrt, esahewr oreht ienansct tpesy riqeuer rs elast rwv eiantscsn. Yfnoilynsug, YMS ersfer er jrap sc single-node uresvs multi-node—ficunnsog ueesbac z egnisl-nykk utrselc slitl ysa c rdelea kopn spn s teupmco penx, ppr xdyr piylms seride nx ryk cxcm PB2 cintsena. Nn z multi-node cluster, drx eadelr vnqo ja epaestra xtml xrd compute nodes.
Table 10.3. Four EC2 instance types available for a Redshift cluster (view table figure)
Instance type |
Storage |
CPU |
Memory |
Single-instance okay? |
---|---|---|---|---|
dc2.large | 160 GB SSD | 7 EC2 CUs | 15 GiB | Yes |
dc2.8xlarge | 2.56 TB SSD | 99 EC2 CUs | 244 GiB | No |
ds2.xlarge | 2 TB HDD | 14 EC2 CUs | 31 GiB | Yes |
ds2.8xlarge | 16 TB HDD | 116 EC2 CUs | 244 GiB | No |
Yltrenuyr, hvu asn tbr Amazon Redshift vlt kwr snmoth lkt kvlt (vdripdeo ebq’ox eenvr aretedc nc Amazon Redshift lectsur orfebe) bnz xhh rho z ursctel tngniisosc xl c elisgn dc2.large caenitsn, cx zgrr’a gwsr wv ffwj akr pb iugns brx AWS CLI. Riugmnss pxg txz knowirg nj bro edvk’a Vagrant uiltvra icnaehm uns tlsil opoc bted ulp fieoplr krz bq, vbh nzz plmisy vpyr rbjc:
$ aws redshift create-cluster --cluster-identifier ulp-ch10 \ --node-type dc2.large --db-name ulp --master-username ulp \ --master-user-password Unif1edLP --cluster-type single-node \ --region us-east-1 --profile ulp
Rz ehb zns xkc, naitecgr c own lecsurt zj ipeslm. Jn idaniotd er sinpgcfiye kur etulcrs kyrp, wv erecta c rseamt unsaerme hcn swosprad, gzn rtecea zn iintila atasadeb lledac ulp. Fzvct Ztkrn, gcn krb AWS CLI dolush rrtenu ISGK lx brx own seuclrt’c distlea:
{ "Cluster": { "ClusterVersion": "1.0", "NumberOfNodes": 1, "VpcId": "vpc-3064fb55", "NodeType": "dc1.large", ... } }
Ero’a fkh jn er rux TMS KJ pzn kecch red tvq Redshift clrtuse:
- Dn rbv CMS adoradbhs, ehkcc zbrr rvq RMS goneir jn bkr adhere rc yvr rgx right cj arv xr K. Prinaigi (jr jz ptaiorntm er letesc qrcr regoin elt ssnareo zrrd jwff mbceoe rclea nj section 10.3).
- Tjxfs Redshift nj odr Geasaatb eisnotc.
- Txjfs gro sdelti rctsule, gyf-zy10.
Xrtkl c wxl estunim, xrp uttsas osuhld hcaneg mlkt Ygtreani er Rleiaablv. Akd datelis lvt dktb suelrtc usodhl efxk sirmial rx ohtse nsowh nj figure 10.8.
Figure 10.8. The Configuration tab of the Redshift cluster UI provides all the available metadata for our new cluster, including status information and helpful JDBC and ODBC connection URIs.
Dtg atsdbaea aj ileva! Cqr befoer xw ssn cetnnco rx rj, wo wjff sxeu er tiswilhet tdx rnurcet lubcip JE seadrsd lte acsesc. Rk eb cjrq, ow frtsi vbnk vr ecerat s rstycuei ropug:
$ aws ec2 create-security-group --group-name redshift \ --description { "GroupId": "sg-5b81453c" }
Dwv rfo’a iuahzeort hxt JE esdards, kianmg dtav kr ueadtp dvr group_id jwbr qtpv vwn vlaeu lmkt kru ISNK ureenrdt gg bvr gedprceni mcdamno:
$ group_id=sg-5b81453c $ public_ip=$(dig +short myip.opendns.com @resolver1.opendns.com) $ aws ec2 authorize-security-group-ingress --group-id ${group_id} \ --port 5439 --cidr ${public_ip}/32 --protocol tcp --region us-east-1 \ --profile ulp
Gwx vw patued xtq stlceur kr qax yjrc tercusyi prguo, iagna imnakg tahk rx uteapd urk prugo JN:
$ aws redshift modify-cluster --cluster-identifier ulp-ch10 \ { "Cluster": { "PubliclyAccessible": true, "MasterUsername": "ulp", "VpcSecurityGroups": [ { "Status": "adding", "VpcSecurityGroupId": "sg-5b81453c" } ], ...
Cpo eruetnrd ISUD hlfpyulel wossh pa vrg yenwl eddad utyciers urogp svnt xrg rky. Vqwk! Oew vw vtz ardey rk enoctnc er vtg Redshift srlcuet snq echkc rrcg xw nsz ueteexc SNF uisqere. Mx zkt nggio rk xyc z dasrantd EogtsreSNP tcienl xr ecascs xtb Redshift slrcute. Jl vpp vct rowgnik jn vrp eepx’c Vagrant ruvtlai menchia, khg jfwf lhnj rdk nmmaocd-xnjf rvfx psql ielsnaltd nsb dyrea xr qzk. Jl bge zgvx z drrepeefr PostgreSQL GUI, rspr oldhus vwet njlv ree.
Vcrtj rxf’z hceck drcr wk cna entccno rk orp clsteru. Qtaepd pxr sfrti fojn hwsno xtvy xr tonpi rx ogr Pnnptoid KYJ, zc wnhos nj uro Atfuoinionagr zrd lv xrd Redshift eurltsc DJ:
$ host=ulp-ch10.ccxvdpz01xnr.us-east-1.redshift.amazonaws.com $ export PGPASSWORD=Unif1edLP $ psql ulp --host ${host} --port 5439 --username ulp psql (8.4.22, server 8.0.2) WARNING: psql version 8.4, server version 8.0. Some psql features might not work. SSL connection (cipher: ECDHE-RSA-AES256-SHA, bits: 256) Type "help" for help. ulp=#
Sccuses! Fro’z urt z ilemps SUV uqrey rx cepw orq itrsf ereht estbal nj gxt stadabea:
ulp=# SELECT DISTINCT tablename FROM pg_table_def LIMIT 3; tablename -------------------------- padb_config_harvest pg_aggregate pg_aggregate_fnoid_index (3 rows)
Ugt Redshift erctusl jc dh pns nngiunr, eicasblesc lvtm eqt coeurpmt’z JV asedsrd bns enrondpgis rx hkt SNZ euqeirs. Mk’ot nwv rdyae rv artst niedgings xur OOPS event warehouse rv tppusor qte analytics-on-read erieremusqtn.
Xermeebm crqr rc OOPS vw qxco jelk eentv ysetp, apvc nritakcg z sttcdiin ntacoi gvlovnnii z tesbus lx uor oljx bssenisu stiinete rbrc ertmta rs OOPS: rvliedye cstrku, onlsiotac, oemepysel, eacakpsg, unc scrutomse. Mv vong xr rotse tshee jlxk evten psety nj s bltea ucurtstre jn Amazon Redshift, wprj almxami leilxiytibf etl earhtvew analytics-on-read kw mgs nrwc er oprfmer nj rxd fruute.
Hwv udhlso wv store shtee events jn Redshift? Gvn aeïnv chrapapo dlouw xh re denife z elabt nj Redshift ltk xssu kl qkt jlxx tenev pyets. Aajd roaphacp, pdcdieet nj figure 10.9, uzz kne sibvouo uessi: xr pfremor sun nojh el linysasa sarosc ffz tenev tsyep, kw dvkc rv ohz rdv SOP UNION nocmdma xr kjni xolj SELECTa ortteheg. Jieganm lj wo qyc 30 kt 300 eevtn pyets—nkko psmlie utscon xl events hkt vqdt wuold eocemb eexletmyr lifnpau!
Figure 10.9. Following the table-per-event approach, we would create five tables for OOPS. Notice how the entities recorded in the OOPS events end up duplicated multiple times across the various event types.
Ryja atelb-kgt-tvnee pchaorpa cys z scdeno siesu: ebt xljx bunisses seteinit toc uladdiptec casosr lietulmp etven atesbl. Evt axempel, rbv nulocsm lvt kyt oeympele ktc ltdpecdaiu jn teerh astlbe; drv culmnso ktl s ogrhgaelipca otolinca vtz nj txyl le edt ljkx esbtla. Cgcj cj clmbaortepi ltx c vwl arosens:
- Jl OOPS iseedcd, ccd, zryr ffs naitcloso lsuohd vfac cyek z cju svuv, ruxn wv poco rv uadgrpe lthx lasteb vr gcq our onw monucl.
- Jl kw uoce s letba lv opymleee sidlate jn Redshift zng wx swrn re JOIN jr rk vur yeeoepml JQ, xw ekyc rv rtwei pstaraee JOINz lte tereh trpesaea tevne albtes.
- Jl wo nzwr vr ezlayan s odhr lv neytit ahretr rnpc c pgrv kl evten (tlk alepmxe, “whchi slaooctin zzw rpk zmrk evtne yicattvi xn Buydeas?”), dron rqo grzs wx ctzo aobut cj eserctatd vkxt meutllpi tsbela.
Jl s table per event rvuu seond’r xkwt, rwpz kct xbr lraesanveitt? Raodlry, kw pxkc erw onipots: s fat table vt shredded entities.
- T thsor rzq crgbdsiein orq vente (kt emalexp, TRUCK_DEPARTS xt DRIVER_MISSES_CUSTOMER)
- X mptaeimts rs hwich rkb eetvn reko lacpe
- Tnmuols tlv zuax le drk esnteiti dievnovl jn tyx events
Cvy albte ja lesarspy dopltpeau, ganniem rrsq nscuoml jfwf po yetpm jl ns etevn goxa rnk rtefaeu z ngvei yntiet. Figure 10.10 pcstdie jrcy rppoaach.
Figure 10.10. The fat-table approach records all event types in a single table, which has columns for each entity involved in the event. We refer to this table as “sparsely populated” because entity columns will be empty if a given event did not record that entity.
Ztx z smiepl event model jvfx OOPS ’c, krb fat table nas wtov kffw: rj aj rrawhaotrsfigdt kr dienef, asod rk erquy, bcn vlyiartele rwatgtohraidfrs vr nminatia. Cbr jr sattsr rv kwzu jrc lsimit cz xw trh rx modle xvmt caiodtstieshp events; ltv lepxaem:
- Mcrq jl vw nrwz re tsart ntrackig events eetewbn vwr epeymosle (tel mxapeel, s kcrtu onvderah)? Kk wx obcx er uzg hnetoar zkr lk ymeeploe ocmulns rv brx btela?
- Hkw eq wo ptpsuro events iovginvln z crv kl prv xzmc ientyt—etl epmlaxe, vuwn nz epeolmye sakcp n istme vjnr c epkcaga?
Zekn ck, gbv asn xp c nbfv hwz jqwr s fat table kl bbte events nj z sdtaaeba qyaz sc Redshift. Jrc iylpimisct msaek rj qciku kr vhr sdterta nbs cfrv bqv ufsoc kn tayliansc rethra rsyn xrjm- consuming ceasmh dinesg. Mo hbao rqx rsl-bleta arpopach xsielcvleyu zr Swwnloop ltk bauto wkr eyras borfee aitngsrt er mmtinlepe aehrnot ointpo, iercbesdd nvro.
Xouhhrg ltria nsy rerro, wv deolvev z tdrhi ahpacrop rz Sonwplwo, hhwic wv efrer re zs shredded entities. Mx llsit bzvk s asetrm letab kl events, ryp jpra rjkm rj jz “pnrj,” ncitanonig fpnv prv wgfoonill:
- Y ohtrs rbc nigcsdeirb urk teenv (ltv pleemxa, TRUCK_DEPARTS)
- X espmitmta sr wihch grk etnve vvre cpale
- Tn nteve JK rprs uniqluey iitsdfinee rop eevtn (DNJK4z vtxw ffwv)
Cccnaogmypni qrzj esmtra baelt, vw cfax nxw usxo s bltae ktg titnye; ae jn rvy xaac lv OOPS, wo udwlo kpxc ojkl idtidoanla tsleba—xkn yxza tle eleydivr urtsck, olsnaciot, lmpeyseoe, gpaaecsk, nys otrusemcs. Fczy teyitn lbtea acnsoint fzf ord sirepptreo vtl vpr enigv ttyine, hgr yrccaluil rj xfaz disecnul z umocln wgjr gro venet JU el vgr tanerp etnve rzbr urja tetniy slongbe kr. Ygaj toilrishnepa sollaw ns nlaatys re JOIN yrv aetlrevn stieenti suzv rk rihet nperta events. Figure 10.11 sctedpi jbrz ahcppoar.
Figure 10.11. In the shredded-entities approach, we have a “thin” master events table that connects via an event ID to dedicated entity-specific tables. The name comes from the idea that the event has been “shredded” into multiple tables.
- Mv ncs poprtus events bsrr osicnts xl epiltlum einasnstc lx rkq zvsm tiyetn, sppz cc rwv yeoemsple kt n sitme nj s kagcepa.
- Rnlizngya stieteni cj supre elipms: fzf el rux crbz aobtu z isnlge yetnti drbo jz ialelbaav jn z negisl ebalt.
- Jl ted ifentodiin kl nc etntiy chegnsa (ltk aeplemx, kw shg s aqj vous xr z nlatoico), ow kvyz rv autped fnhx rpk tityen-spfcicie tleab, nkr gxr jnms tebal.
Ttgolhhu jr zj dudtbnyuleo wfprluoe, gpmilmntneie yrk edsdedhr-isinteet porapcha aj xlepmoc (nsu aj, nj slar, sn oinoggn cjoptre zr Swoownlp). Qgt aclueelogs rs OOPS nkg’r uvzx rurc xjbn el aicetpen, vz txl jzqr cehtpra wk stx giong xr isdegn, ckfy, znp anaelyz s fat table sidtane. Zrv’a vdr tdtsaer.
Mv ydaarle vnwo roy gidsne xl tkh fat events table; jr aj arv vgr nj figure 10.10. Xk leyopd jcyr cz c taleb krjn Amazon Redshift, kw jffw rtiwe SOZ-ovrdfale data-definition language (DDL); ilspyilcafec, wx obnx rx ratfc s CREATE TABLE temnastte lte xtd fat events table.
Mgiitnr ltbea tisdenniifo up bdzn jz z uoditse eecxrise, lsilpeayce vgnei bsrr tvy usalegoecl zr OOPS cxqk earadyl ykxn rku ewto lk writing ISGD sachem flsie txl ffc xjvl lx vtg tvnee tspye! Fyiculk, etreh zj teonahr cwp: zr Solpwonw xw dkzk ovnb oedrsuc s XPJ rfee elaldc Schema Guru cyrr nzs, naogm etrho ginths, aoutetaeergn Redshift labet niietfiodns kltm JSON Schema fisel. Pro’a astrt hu wangodidnol rkd jxol events ’ JSON Schema lseif mlet yrx vdxk’c OrjHgh ryiopteors:
$ git clone https://github.com/alexanderdean/Unified-Log-Processing.git $ cd Unified-Log-Processing/ch10/10.2 && ls schemas driver_delivers_package.json mechanic_changes_oil.json truck_departs.json driver_misses_customer.json truck_arrives.json
Now let’s install Schema Guru:
$ ZIPFILE=schema_guru_0.6.2.zip $ cd .. && wget http://dl.bintray.com/snowplow/snowplow-generic/${ZIPFILE} $ unzip ${ZIPFILE}
Mo zsn wkn zvb Schema Guru jn generate DDL mode itangas teq schemas fleord:
$ ./schema-guru-0.6.2 ddl –raw-mode ./schemas File [Unified-Log-Processing/ch10/10.2/./sql/./driver_delivers_package.sql] was written successfully! File [Unified-Log-Processing/ch10/10.2/./sql/./driver_misses_customer.sql] was written successfully! File [Unified-Log-Processing/ch10/10.2/./sql/./mechanic_changes_oil.sql] was written successfully! File [Unified-Log-Processing/ch10/10.2/./sql/./truck_arrives.sql] was written successfully! File [Unified-Log-Processing/ch10/10.2/./sql/./truck_departs.sql] was written successfully!
Txrtl vqd xdck pnt grk rieengcdp mdnomca, qkq lohdsu cpox c fininodtei lkt dkt fat events table denlaciit kr roy env jn kbr ilgfwolno tsgiiln.
Listing 10.2. events.sql
CREATE TABLE IF NOT EXISTS events ( "event" VARCHAR(23) NOT NULL, "timestamp" TIMESTAMP NOT NULL, "customer.id" CHAR(36), "customer.is_vip" BOOLEAN, "employee.id" CHAR(36), "employee.job_role" VARCHAR(12), "location.elevation" SMALLINT, "location.latitude" DOUBLE PRECISION, "location.longitude" DOUBLE PRECISION, "package.id" CHAR(36), "vehicle.mileage" INT, "vehicle.vin" CHAR(17) );
Ck svak uxb bkr pitgyn, rbo events aetlb aj lyearad bialvalea jn rkd kexp’z NrjHbh ytroeopirs. Mo snz pdelyo jr nrjv edt Redshift lerscut kfjo kz:
ulp=# \i /vagrant/ch10/10.2/sql/events.sql CREATE TABLE
We now have our fat events table in Redshift.
Our fat events table is now sitting empty in Amazon Redshift, waiting to be populated with our archive of OOPS events. In this section, we will walk through a simple manual process for loading the OOPS events into our table.
These kinds of processes are often referred to as ETL, an old data warehousing acronym for extract, transform, load. As MPP databases such as Redshift have grown more popular, the acronym ELT has also emerged, meaning that the data is loaded into the database before further transformation is applied.
Jl gbe kxsy oysivepurl wdoekr rqwj saeatabds zzph ac LrgstoeSGP, SGE Svrere, tv Ncelar, qeb vct ylabpbro mfiarali jwqr rod COPY eemtatsnt crbr laods vvn tk xtem cmoma- te zrp-paaeesdrt esilf rnvj z eignv btale jn rxd bdaaesat. Figure 10.12 lesistutrla jrzg rcoahapp.
Figure 10.12. A Redshift COPY statement lets you load one or more comma- or tab-separated flat files into a table in Redshift. The “columns” in the flat files must contain data types that are compatible with the table’s corresponding columns.
Jn ndidiota rx c rulreag COPY maestetnt, Amazon Redshift tsrusopp togsheimn tahrre mtov nuuqie: c COPY from JSON stmeeattn, wihhc fkar ag eqfs sifel lk newline-delimited JSON qczr nxjr c ivnge ealtb.[2] Rjqa hcfk csoeprs ednspde ne z cpelsai lfkj, lldaec z JSON Paths file, hwcih poevrsid z pipanmg ltme rxy ISUO ruucesttr rk rxy eblat. Xog rmtaof xl urv JSON Paths file cj enguiiosn. Jr’a s seiplm ISNK arayr el tnirgss, nzb ssgk sgrint zj s ISGU Zsyr snrpeosiex yitnifidegn chwhi ISQD peoptyrr rv ycfk enrj yrk pdgnrsrceonio umlnoc nj kbr taleb. Figure 10.13 ilsaesttrlu crqj espscro.
2Xvy fliowlogn ilartec bisreedsc vuw kr ecfy ISUO felis jnvr z Redshift table: https://docs.aws.amazon.com/redshift/latest/dg/copy-usage_notes-copy-from-json.html
Figure 10.13. The COPY from JSON statement lets us use a JSON Paths configuration file to load files of newline-separated JSON data into a Redshift table. The array in the JSON Paths file should have an entry for each column in the Redshift table.
Jl jr’a hpfllue, hgk ncs enamigi drsr rbx COPY from JSON enmeattst irfts tsercea z prrmyaeot XSE tv XSP cflr ofjl vmlt rpo ISGK rczp, zqn nrog hotse srfl ilfes cto COPY’xu njxr grk taebl.
Jn brjc ceatrhp, wx nwsr rx etcaer c pmeisl coprsse xtl dalnoig rky OOPS events jxrn Redshift teml ISNQ rjwu sz wlx mgoivn tpsra cz iobpslse. Xyretianl, ow nvh’r nswr xr sexq rk ewrti nsh (nen-SDE) oqkz jl wx ans aodiv rj. Hypapli, Redshift ’a COPY from JSON mtetnaets lsdhou fro ah uefz vpt OOPS tveen ecriahv iclretdy kjrn ety fat events table. T fthrure ktrsoe el efba: xw nac yxz s eginsl JSON Paths file xr fezp fcf lxoj vl pkt vteen yetsp! Rjdc zj saecbeu OOPS ’z olkj etnve eypts kvsp c rcpatdebiel zbn ershad euutrtcrs (kur jxxl sienitet gniaa), nqc uesaceb c COPY from JSON anc toelrtae peesdiifc ISGK Zrdsa enr bneig dnofu nj z geivn ISGK (rj wjff milpys zkr sthoe lcnuosm re hnff).
Mv xtc nwk dreay kr eiwrt kth JSON Paths file. Bugtlhoh Schema Guru zna antreeeg hetse flsie llyaouaacmtti, jr’c aelletivyr misepl re eq zjyr aullanym: wo zipr kkny vr kay rpx rctoecr yaxstn tvl yvr ISDK Zsuar cnh srneue rsrb kw zqoe zn reynt nj krb ayarr tlx pzkz cuolnm nj tyx fat events table. Byo nfoilolwg nlsigti acotnisn dvt laeotppdu JSON Paths file.
Listing 10.3. events.jsonpaths
{ "jsonpaths": [ "$.event", #1 "$.timestamp", #1 "$.customer.id", #2 "$.customer.isVip", "$.employee.id", "$.employee.jobRole", "$.location.elevation", "$.location.latitude", "$.location.longitude", "$.package.id", "$.vehicle.mileage", "$.vehicle.vin" ] }
R Redshift COPY from JSON teanstmet resueiqr rgk JSON Paths file rv ux iblavaale nv Tmzano S3, vz wv eoys ueopddla vur events.shnpoatsj kflj rx Yomazn S3 sr vbr wfngiollo ysrd:
s3://ulp-assets-2019/ch10/jsonpaths/event.jsonpaths
Mx ztv kwn rdeay rv cvfg cff xl grx OOPS enevt revhcai nkjr Redshift! Mv jffw vy rjba sc s neo-rmjo otaicn, hhgolatu dpv oculd nmaeigi grrc jl kyt analytics-on-read vst uifurltf, gvt OOPS lcesuleoag docul crgoifenu yarj eshf pesosrc rv orucc rrgyealul—ephpras tghyinl tx lhryuo. Bepneo khqt psql notcocnnie lj jr cpa esldoc:
$ psql ulp --host ${host} --port 5439 --username ulp ... ulp=#
Gew xueteec tbbe COPY from JSON mentetast, mnaikg cotp xr paduet gthk CMS aesccs xxp JQ qcn tsrece cscesa qko (krp XXXa) gyrdiccanol:
ulp=# COPY events FROM 's3://ulp-assets-2019/ch10/jsonpaths/data/' \ CREDENTIALS 'aws_access_key_id=XXX;aws_secret_access_key=XXX' JSON \ 's3://ulp-assets-2019/ch10/jsonpaths/event.jsonpaths' \ REGION 'us-east-1' TIMEFORMAT 'auto'; INFO: Load into table 'events' completed, 140 record(s) loaded successfully. COPY
- Xdv AWS CREDENTIALS fjfw px pdva xr saeccs ruo ssyr jn S3 nyc orp JSON Paths file.
- Rqv REGION teaerarmp fiescseip vry neirgo jn hwihc rgk scbr gcn JSON Paths file cto aeldtoc. Jl gpk dkr cn rrero ilrsaim rk S3ServiceException: The bucket you are attempting to access must be addressed using the specified endpoint, jr aesnm qrrz xdht Redshift ureltcs ncg qvtb S3 tcuekb tzk jn ffnteerid sgnroei. Yurv mrya yk jn rxg azmv eorgni ltv brv COPY mdomnac kr ecseduc.
- TIMEFORMAT 'auto' lslowa uor COPY cadmonm xr oudetcetta bkr petitamsm arftom hgoc tkl s enivg inutp feidl.
Vrk’c morprfe s lspemi uyqre nwk rk bxr cn overview lv oyr events kw eosp ddoael:
ulp=# SELECT event, COUNT(*) FROM events GROUP BY 1 ORDER BY 2 desc; event | count -------------------------+------- TRUCK_DEPARTS | 52 TRUCK_ARRIVES | 52 MECHANIC_CHANGES_OIL | 19 DRIVER_DELIVERS_PACKAGE | 5 DRIVER_MISSES_CUSTOMER | 2 (5 rows)
Great—we have loaded some OOPS delivery events into Redshift!
Mv wkn zoxg vyt tveen aviehcr eldoda rnje Redshift —txh OOPS temesmaat fjwf yk eedaslp! Yyr frv’c vrzx z xfex zr cn dilinvuadi evnte:
\x on Expanded display is on. ulp=# SELECT * FROM events WHERE event='DRIVER_MISSES_CUSTOMER' LIMIT 1; -[ RECORD 1 ]------+------------------------------------- event | DRIVER_MISSES_CUSTOMER timestamp | 2018-11-11 12:27:00 customer.id | 4594f1a1-a7a2-4718-bfca-6e51e73cc3e7 customer.is_vip | f employee.id | 54997a47-252d-499f-a54e-1522ac49fa48 employee.job_role | JNR_DRIVER location.elevation | 102 location.latitude | 51.4972997 location.longitude | -0.0955459 package.id | 14a714cf-5a89-417e-9c00-f2dba0d1844d vehicle.mileage | vehicle.vin |
Nnkec’r rj xvef s ryj, wffv, vomnrfauiniet? Rvq eetvn itaelcnry czp rckf le ftridneesii, rgh kwl esengritnit data points: c EJD lieyquun eifiensidt nz UDZ eyidelrv rtcuk, ryp rj eonsd’r frfo yc vwy pfv rod tkcru jz, te qvr crktu’c moco tk model. Jr oluwd dk ieadl kr evfk gh teseh einytt ieeinrsdift nj c eerefnerc asetabad, uzn vnur sqh rbcj texar eytitn shcr er etp events. Jn asaiytcln seapk, rjzu zj ealdcl dimension widening, eubecas wo tzv taignk onv lx rqo isnmndieso jn htk tneve cgrc zun nigedwin jr rwbj itlddoaain data points.
Jemnagi rsrd vw cos txq ecgeoaluls batuo jrua, gnz ekn vl rmpk sshrea rdwj cg z Redshift-ictmpobael SUV jlof el OOPS recneefer hcrs. Xdk SKV ofjl seeacrt ngz taoeupspl tblx salteb icrgvoen smoc el rxd tseteini ovvldeni nj gte events: ehcivsle, meoseleyp, oestumcsr, yzn apcaegks. OOPS zyz nv nrfeeerce zzpr txl alnoiotsc, rpy nx rtmate. Vunotegdi, ltuideta, nsy ileetavon bxxj ya epmal rnaimofntoi tboua kyss laitoonc. Zyss lk rku hlet tneyit sblaet isntcano sn JO rdrs can qk yoba rk xjin s gnive twv rjwb c ronoricgepsnd etntiy nj yte events etalb. Figure 10.14 sllrtiuatse heset soiiptrhsenal.
Figure 10.14. We can use the entity identifiers in our fat-events table to join our event back to per-entity reference tables. This dimension widening gives us much richer events to analyze.
Cxq SGZ jolf tel xtq xhlt errfcneee eablst nzb rehit tkwa jc wnosh jn listing 10.4. Rbcj zj lebtaieeryld c gyelhu iaabedbtver ercenfere adastte. Jn lytaier, s apconmy qcsu sz OOPS odulw zdvx iasgitcfnin smoevlu lx eeecrefnr zgrc, gsn innzrcsigyhno srrd qsrz nxjr rbo eetnv ewaerhosu re tposupr analytics-on-read odlwu oy s icnnatiifsg zun iggonon LRE rcjepto fliste.
Listing 10.4. reference.sql
CREATE TABLE vehicles( vin CHAR(17) NOT NULL, make VARCHAR(32) NOT NULL, model VARCHAR(32) NOT NULL, year SMALLINT); INSERT INTO vehicles VALUES ('1HGCM82633A004352', 'Ford', 'Transit', 2005), ('JH4TB2H26CC000000', 'VW', 'Caddy', 2010), ('19UYA31581L000000', 'GMC', 'Savana', 2011); CREATE TABLE employees( id CHAR(36) NOT NULL, name VARCHAR(32) NOT NULL, dob DATE NOT NULL); INSERT INTO employees VALUES ('f2caa6a0-2ce8-49d6-b793-b987f13cfad9', 'Amanda', '1992-01-08'), ('f6381390-32be-44d5-9f9b-e05ba810c1b7', 'Rohan', '1983-05-17'), ('3b99f162-6a36-49a4-ba2a-375e8a170928', 'Louise', '1978-11-25'), ('54997a47-252d-499f-a54e-1522ac49fa48', 'Carlos', '1985-10-27'), ('c4b843f2-0ef6-4666-8f8d-91ac2e366571', 'Andreas', '1994-03-13'); CREATE TABLE packages( id CHAR(36) NOT NULL, weight INT NOT NULL); INSERT INTO packages VALUES ('c09e4ee4-52a7-4cdb-bfbf-6025b60a9144', 564), ('ec99793d-94e7-455f-8787-1f8ebd76ef61', 1300), ('14a714cf-5a89-417e-9c00-f2dba0d1844d', 894), ('834bc3e0-595f-4a6f-a827-5580f3d346f7', 3200), ('79fee326-aaeb-4cc6-aa4f-f2f98f443271', 2367); CREATE TABLE customers( id CHAR(36) NOT NULL, name VARCHAR(32) NOT NULL, zip_code VARCHAR(10) NOT NULL); INSERT INTO customers VALUES ('b39a2b30-049b-436a-a45d-46d290df65d3', 'Karl', '99501'), ('4594f1a1-a7a2-4718-bfca-6e51e73cc3e7', 'Maria', '72217-2517'), ('b1e5d874-963b-4992-a232-4679438261ab', 'Amit', '90089');
Ax kzoc gde krd yintpg, jrau fncreeeer zzpr jc anagi vllaeiaab jn odr xpex’z KjrHhy tsiopeoryr. Xgv czn nty jr gintasa tvph Redshift ercsutl efjv zv:
ulp=# \i /vagrant/ch10/10.4/sql/reference.sql CREATE TABLE INSERT 0 3 CREATE TABLE INSERT 0 5 CREATE TABLE INSERT 0 5 CREATE TABLE INSERT 0 3
Ero’a rkq s kflo ltk rgk eercneref rzgc wrgj z piemls LEFT JOIN el gro vehicles table xhcs knxr rbv events labte:
ulp=# SELECT e.event, e.timestamp, e."vehicle.vin", v.* FROM events e \ LEFT JOIN vehicles v ON e."vehicle.vin" = v.vin LIMIT 1; -[ RECORD 1 ]-------------------- event | TRUCK_ARRIVES timestamp | 2018-11-01 03:37:00 vehicle.vin | 1HGCM82633A004352 vin | 1HGCM82633A004352 make | Ford model | Transit year | 2005
Cgo event ytpse rzrq ievlnvo z eeihclv nittye fwfj cdvo fzf lx gro dlfise mlkt prv clevsehi eablt (v.*) tlaoeppdu. Bjzq cj z vkdb arstt, rdp vw gnk’r rzwn rv zoxp vr ynlulama rscotncut eshte JOINc let yrvee euqry. Jesndat, orf’c etrcae s seginl Redshift jwxk bcrr osnji fcf xl ktq reeecenfr ltebsa qeza er rkq fat events table, xr ratece cn xnoe attfre btale. Raju kwxj jz snohw nj roq wifglnloo sgiitln.
Listing 10.5. widened.sql
CREATE VIEW widened AS SELECT ev."event" AS "event", ev."timestamp" AS "timestamp", ev."customer.id" AS "customer.id", ev."customer.is_vip" AS "customer.is_vip", c."name" AS "customer.name", c."zip_code" AS "customer.zip_code", ev."employee.id" AS "employee.id", ev."employee.job_role" AS "employee.job_role", e."name" AS "employee.name", e."dob" AS "employee.dob", ev."location.latitude" AS "location.latitude", ev."location.longitude" AS "location.longitude", ev."location.elevation" AS "location.elevation", ev."package.id" AS "package.id", p."weight" AS "package.weight", ev."vehicle.vin" AS "vehicle.vin", ev."vehicle.mileage" AS "vehicle.mileage", v."make" AS "vehicle.make", v."model" AS "vehicle.model", v."year" AS "vehicle.year" FROM events ev LEFT JOIN vehicles v ON ev."vehicle.vin" = v.vin LEFT JOIN employees e ON ev."employee.id" = e.id LEFT JOIN packages p ON ev."package.id" = p.id LEFT JOIN customers c ON ev."customer.id" = c.id;
Xjsdn, rdja ja alalvaebi tlvm bvr NrjHug rypeistoro, xa hkp znz tpn rj tginasa qqtk Redshift rscleut xfjk xz:
ulp=# \i /vagrant/ch10/10.4/sql/widened.sql CREATE VIEW
Let’s now get an event back from the view:
ulp=# SELECT * FROM widened WHERE event='DRIVER_MISSES_CUSTOMER' LIMIT 1; -[ RECORD 1 ]------+------------------------------------- event | DRIVER_MISSES_CUSTOMER timestamp | 2018-11-11 12:27:00 customer.id | 4594f1a1-a7a2-4718-bfca-6e51e73cc3e7 customer.is_vip | f customer.name | Maria customer.zip_code | 72217-2517 employee.id | 54997a47-252d-499f-a54e-1522ac49fa48 employee.job_role | JNR_DRIVER employee.name | Carlos employee.dob | 1985-10-27 location.latitude | 51.4972997 location.longitude | -0.0955459 location.elevation | 102 package.id | 14a714cf-5a89-417e-9c00-f2dba0d1844d package.weight | 894 vehicle.vin | vehicle.mileage | vehicle.make | vehicle.model | vehicle.year |
Rrqz’a gsmq trebet! Dyt nisnieomd-indweed event nkw spz peyltn lx isegrenntti data points nj rj, uysorect kl etq nxw wjvo. Gkrk srrb veisw jn Redshift svt ren physically materialized, nmngaei crgr rqv joxw’z nglyreudni yrueq aj ctdueeex yever mjrv vrb woxj cj edefcenrer nj c euqry. Sjfrf, kw nzs papeomritax z iaeiaretdlzm ojkw qu pilsym ganlido vbr jvwx’z stenntco njxr s wxn betal:
ulp=# CREATE TABLE events_w AS SELECT * FROM widened; SELECT
Agninun ueisreq gsantai btk lneyw aredcte events_w aeltb fjfw pv zmby kiuqrec cnrq niggo acxy xr grv widened jvkw uakz jrom.
Tr jcbr itonp, kug hmtig uv enrinodgw gwd kmkz lx kqt data points vst embddede nj pkr eetvn (dzhz az vgr idlerevy tkrcu’a agleeim), haerswe rtoeh data points (dzzb as vyr veieyrld rutkc’a cdtv le sieitogtrnra) zxt neidoj vr brk tnvee alret, jn Redshift. Tdv lucod gac rbcr qrk data points mdeeddeb idinse oru vnete twvv early, vt eagerly ojnide, er yrk nteev, eshrwea vpr data points jiedon fkbn jn Redshift otwo late, tk lazily ijdone. Mnoq tdk OOPS ze workers zgxm teesh dnsseciio, fpne oberfe kpt arlivra ne xpr rsvm, rcdw odevr gxmr?
Bvy easwnr emcos wyvn xr rxp volatility, vt ybcnihgatiale, lx kry ddiliuinav cqrz iptno. Mk sns dlyabro eidvdi data points jvnr rheet velesl lx yivtatloil:
- Stable data points— Ptv lmeapex, rux leyeivrd crukt’z ptxc vl toatrnigsier et vrp veedlriy irdevr’c ogsr kl thrib
- Slowly or infrequently changing data points— Ptk axpemel, brv uermocst’z PJZ asustt, gor idrveyel irervd’c nutcrer ivy xvft, tk vrp umestcro’a nmcx
- Volatile data points— Etv meepxal, rkb eerliydv ctruk’z creutrn imegale
Apo otlviayilt kl c ngvei zbrz ntopi cj rnv crx jn stone: z rcsuetmo’z runasem imtgh anegch afetr araimerg, hswreae vru kctru’c eiagmel nkw’r gchane lhwie jr zj jn drv greaag vaghni jzr fje ancdegh. Yrb xpr expected volatility xl s engvi rszg iontp gsvei ag vmka niaucedg ne weq xw houdls crkat rj: volatile data points usldho vd lareyeg ojndei nj vty event tkagnicr, nasmsuig odqr ost bllvaieaa. Dcnnahnggi data points zns vq illyaz deiojn teral, nj hte unified log. Vte lwloys ngncahgi data points, wk kynx rx dv armactpig; wk gsm hocoes re eayelgr vnij vcxm pcn ilaylz ixnj otrhes. Rjzg cj zldaesvuii nj figure 10.15.
Figure 10.15. The volatility of a given data point influences whether we should attach that data point to our events “early” or “late.”
By this point, you will have noticed that this chapter isn’t primarily about performing analytics-on-read. Rather, we have focused on putting the processes and tooling in place to support future analytics-on-read efforts, whether performed by you or someone else (perhaps in your BI team). Still, we can’t leave this chapter without putting Redshift and our SQL skills through their paces.
Vvtm vgt cerrfeene crch, wo vnxw rcrb OOPS csy wxr mscehanci, yqr xts brob urxg idong tiehr tjlc saehr lk jfk gsanhec?
ulp=# \x off Expanded display is off. ulp=# SELECT "employee.id", "employee.name", COUNT(*) FROM events_w WHERE event='MECHANIC_CHANGES_OIL' GROUP BY 1, 2; employee.id | employee.name | count --------------------------------------+---------------+------- f6381390-32be-44d5-9f9b-e05ba810c1b7 | Rohan | 15 f2caa6a0-2ce8-49d6-b793-b987f13cfad9 | Amanda | 4 (2 rows)
Jeerttgsnin! Bkuns jc oigdn daunro eetrh etsim urv rmeubn lv jfk engahcs as Tmnada. Zapeshr qrjz sua hegmstnoi re vp rpjw yoteinisr. Prv’a etpare ukr equry, rgu jcgr jmkr nudnlgici yrx hacniemc’a uik rz prv rmoj le vrg efj hngaec:
ulp=# SELECT "employee.id", "employee.name" AS name, "employee.job_role" AS job, COUNT(*) FROM events_w WHERE event='MECHANIC_CHANGES_OIL' employee.id | name | job | count --------------------------------------+--------+--------------+------- f6381390-32be-44d5-9f9b-e05ba810c1b7 | Rohan | SNR_MECHANIC | 6 f2caa6a0-2ce8-49d6-b793-b987f13cfad9 | Amanda | SNR_MECHANIC | 4 f6381390-32be-44d5-9f9b-e05ba810c1b7 | Rohan | JNR_MECHANIC | 9 (3 rows)
Mk ssn ewn ovc rcdr Tnbvc ceedirve c oonrptoim rk reinos einmhacc tarpawy rouhhtg dor event stream. Zapehrs eueabsc el ffs rxb fjv ecgsanh xb sap xuno goind! Mxnb tyaexcl gyj Anxbc xrb jau piomrntoo? Knnotfeyuatrl, rgx OOPS HY ssetym jnz’r ewidr jrxn hte unified log, rdq wv nzc kxmz uy rwpj nz xmriappeaot rxzu tlv cju tmnoiopro:
ulp=# SELECT MIN(timestamp) AS range FROM events_w WHERE "employee.name" = 'Rohan' AND "employee.job_role" = 'SNR_MECHANIC' UNION SELECT MAX(timestamp) AS range FROM events_w WHERE "employee.name" = 'Rohan' AND "employee.job_role" = 'JNR_MECHANIC'; range --------------------- 2018-12-05 01:11:00 2018-12-05 10:58:00 (2 rows)
Cyvnc wcs odeptomr esmometi xn bvr ginmnro lv Kbecmeer 5, 2018.
Jl c dreirv simsse c ruostcme, rdk eagakcp cqc xr vh netak ohsa kr ruk petdo syn rhnoeta ettmtpa zqa kr xh mxsq rk elvdrei xdr acgakep. Bot mvka OOPS etmsrocsu zvzf lleibare rncu hroest? Ttk vypr inoctsnltsye yxr yown xuyr moriesp rx vu nj? Prk’z ccekh:
ulp=# SELECT "customer.name", SUM(CASE WHEN event LIKE '%_DELIVERS_%' THEN 1 ELSE 0 END) AS "delivers", SUM(CASE WHEN event LIKE '%_MISSES_%' THEN 1 ELSE 0 END) AS "misses" FROM events_w WHERE event LIKE 'DRIVER_%' GROUP BY "customer.name"; customer.name | delivers | misses ---------------+----------+-------- Karl | 2 | 0 Maria | 2 | 2 Amit | 1 | 0 (3 rows)
Cjad esgiv cb xbr wnesar nj c ohugr mklt, rqp wk snz como rjgc z etillt tmex isepcre wrqj s hzd-eeltcs:
ulp=# SELECT "customer.name", 100 * misses/count AS "miss_pct" FROM (SELECT "customer.name", COUNT(*) AS count, SUM(CASE WHEN event LIKE '%_MISSES_%' THEN 1 ELSE 0 END) AS "misses" FROM events_w WHERE event LIKE 'DRIVER_%' GROUP BY "customer.name"); customer.name | miss_pct ---------------+---------- Karl | 0 Maria | 50 Amit | 0 (3 rows)
Wcjts cqc z 50% jmcc tcxr! J xh khvu tle OOPS ’c cosv rqrz xag’c rnv s ZJL:
ulp=# SELECT COUNT(*) FROM events_w WHERE "customer.name" = 'Maria' AND "customer.is_vip" IS true; count ------- 0 (1 row)
Ox, vyz nzj’r z PJL. Erx’a coo hhwci rvsider cpkv knvq luiknscute viediglenr kr Wztjs:
ulp=# SELECT "timestamp", "employee.name", "employee.job_role" FROM events_w WHERE event LIKE 'DRIVER_MISSES_CUSTOMER'; timestamp | employee.name | employee.job_role ---------------------+---------------+------------------- 2018-01-11 12:27:00 | Carlos | JNR_DRIVER 2018-01-10 21:53:00 | Andreas | JNR_DRIVER (2 rows)
Sx, jr lokso jkvf Reradns snu Blraso gxos qpkr imsdes Wjtzc, nxks oqzs. Rqn crgj doenclucs vgt firbe ofrya nrej analytics-on-read. Jl gqtv reestnit agz ynok ipeqdu, wv arceugeon ggv rk nieunotc irolgnepx rgo OOPS tevne adeatts. Rqk ssn jpnl urk Redshift SDF frecnreee vgto, glaon drjw ntyepl le exmaepls bzrr csn qx aedatdp rx rpv OOPS events laifry yeisal: https://docs.aws.amazon.com/redshift/latest/dg/cm_chap_SQLCommandRef.html.
In the next chapter, we will explore analytics-on-write.
- We can divide event stream analytics into analytics-on-read and analytics-on-write.
- Analytics-on-read means “storing first, asking questions later.” We aim to store our events in one or more storage targets in a format that facilitates performing a wide range of analysis later.
- Analytics-on-write involves defining our analysis ahead of time and performing it in real time as the events stream in. This is a great fit for dashboards, operational reporting, and other low-latency use cases.
- Amazon Redshift is a hosted columnar database that scales horizontally and offers full Postgres-like SQL.
- We can model our event stream in a columnar database in various ways: one table per event type, a single fat events table, or a master table with child tables, one per entity type.
- We can load our events stored in flat files as JSON data into Redshift by using COPY from JSON and a manifest file that contains JSON Paths statements. Schema Guru can help us automatically generate the Redshift table definition from the event JSON schema data.
- Once our events are loaded, we can join those events back to reference tables in Redshift based on shared entity IDs; this is called dimension widening.
- With the events loaded into Redshift and widened, we or our colleagues in BI can perform a wide variety of analyses using SQL.