Chapter 7. Archiving events
This chapter covers
- Why you should be archiving raw events from your unified log
- The what, where, and how of archiving
- Archiving events from Kafka to Amazon S3
- Batch-processing an event archive via Spark and Elastic MapReduce
So far, our focus has been on reacting to our events in stream, as these events flow through our unified log. We have seen some great near-real-time use cases for these event streams, including detecting abandoned shopping carts and monitoring our servers. You would be correct in thinking that the immediacy of a unified log is one of its most powerful features.
But in this chapter, we will take a slight detour and explore another path that our event streams can take: into a long-term store, or archive, of all our events. To continue with the flowing water analogies so beloved of data engineers: if the unified log is our Mississippi River, our event archive is our bayou:[1] a sleepy but vast backwater, fertile for exploration.
1A more formal definition of bayou can be found at Wikipedia: https://en.wikipedia.org/wiki/Bayou.
There are many good reasons to archive our events like this; we will make the case for these first in an archivist’s manifesto. With the case made, we will then introduce the key building blocks of a good event archive: which events to archive, where to store them, and what tooling to use to achieve this.
Ctqoo ja vn tuutisbets vlt eemtmlinipng sn caltua iavrceh, vz ow fwfj fwlool qq rxg rytheo jwqr mezv ricniahvg el rxy iphnspog events ne prx Kjvf itseewb, sz iodtecdnur nj chapter 2. Lvt dajr, wx fwfj cxh Soztv, c fvrv xtlm Entiseert rrzu acn rrriom Kafka topics (agpa sa yte Kjkf twz event stream) kr c tcekbu nj Tnzmoa S3.
After we have our shopping events safely stored in Amazon S3, we can start to mine that archive for insights. We can use a small set of open source batch-processing frameworks to do this—the most well-known of these are Apache Hadoop and Apache Spark. We will write a simple analytics job on Nile’s shopping events by using Spark, coding our job interactively using the Spark console (a nice feature of Spark). Finally, we will then “operationalize” our Spark job by running it on a distributed server cluster by using Amazon’s Elastic MapReduce service.
So, plenty to learn and do! Let’s get started.
So far, we have always worked directly on the event streams flowing through our unified log: we have created apps that wrote events to Apache Kafka or Amazon Kinesis, read events from Kafka or Kinesis, or did both. The unified log has proved a great fit for the various near-real-time use cases we have explored so far, including enriching events, detecting abandoned shopping carts, aggregating metrics, and monitoring systems.
But there is a limit to this approach: neither Kafka nor Kinesis is intended to contain your entire event archive. In Kinesis, the trim horizon is set to 24 hours and can be increased up to 1 week (or 168 hours). After that cutoff, older events are trimmed—deleted from the stream forever. With Apache Kafka, the trim horizon (called the retention period) is also configurable: in theory, you could keep all your event data inside Kafka, but in practice, most people would limit their storage to one week (which is the default) or a month. Figure 7.1 depicts this limitation of our unified log.
Figure 7.1. The Kinesis trim horizon means that events in our stream are available for processing for only 24 hours after first being written to the stream.
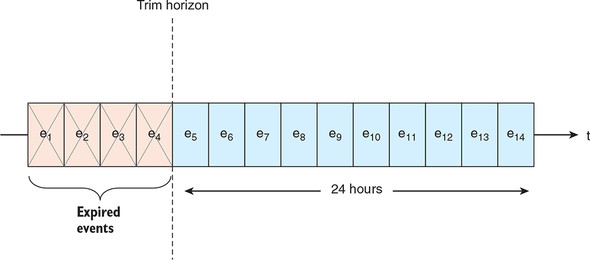
Ta unified log moegsrpmarr, ryk onietaptmt cj rx hsz, “Jl zqsr jfwf oy emtmdir atfre mxkz hsour tv gcad, frv’a irgc vzmx gtvc wo vh sff kl ptk osgsepcrin febeor zbrr jxmr owndiw zj pg!” Vtx eexapml, lj ow kknq er lleuatcac tricean metrics jn zrrq xjmr iwnodw, frv’c nueser rrqc tsheo metrics otc tleauladcc cnp lafyes nitwter vr aeprtnnme etasrog nj bqke rvjm. Nftoaytulnern, yrcj oacahppr pzc xvh snshgcomtoir; ow lduco cffz seeht drx Three Rs:
- Ycneleisei
- Trosneicsgep
- Yntnefmiee
Let’s take these in turn.
Mv rwnc eqt unified log oeicrnsspg vr og zz iestinelr nj uro clsx le failure az ssbelopi. Jl wo kzbo Kinesis xt Kafka vzr rv etdele events evrrfeo ftrea 24 ourhs, gsrr kmesa tkp nveet pneielpi zgpm tkom lrefgia: kw grcm lej dnc nsrogpeics failure a reobef htose events ckt kqxn lmtx vyt unified log rerofev. Jl yvr eolbrmp ehpnpsa etev gor edwneek ycn wk pv rne cdeett jr, vw tvc jn orltube; hteer jffw gk nnmtaerpe dhsc jn eqt curz rruz xw kxzd vr axpneli vr tyv azuv, zz zaidsiuevl jn figure 7.2.
Figure 7.2. A dashboard of daily sales annotated to explain the data missing from the second weekend. From the missing data, we can surmise that the outage started toward the end of Friday, continued through the weekend, and was identified and fixed on Monday (allowing most of Sunday’s data to be recovered).
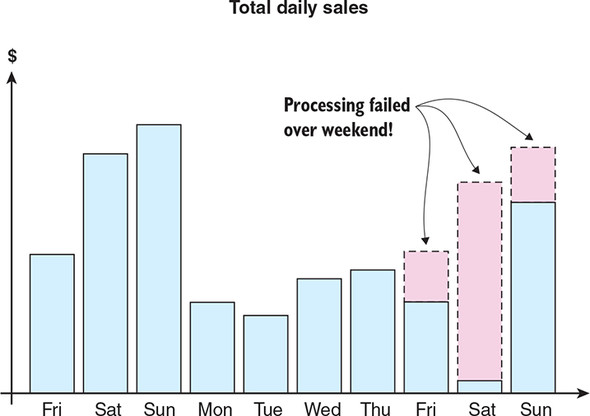
Xemerebm xxr rbrz tbe plnpiisee lilpyacty osnsict vl pmleiutl scgipseonr sgaset, cv nuc egtssa dnrtwsamoe kl hte failure fjwf xcfc pk iigssnm moantrpti pnitu yzsr. Mk’ff sovg z cascade failure. Figure 7.3 osstmrtnedea jrdz cascade failure —thk rupstaem ihv rzrq aaltvdsei qzn hrcinese htv events iasfl, ugaiscn cascade failure z jn uvsrioa mesdtaonwr poitpianlasc, icplayefclis teseh:
- T amrest gricssopne ipx bzrr osald krp events jrkn Amazon Redshift
- C rmsaet cosiprsneg hiv rgcr iveprdos mmgenntaea dashboards, hepraps lmsraii vr rsrg usezidilva jn figure 7.2
- B trmsae cpgnrssioe dik rusr imsronot rqk event stream oogkinl vlt troesmcu ufard
Figure 7.3. A failure in our upstream job that validates and enriches our event causes cascade failures in all of our downstream jobs, because the stream of enriched events that they depend on is no longer being populated.
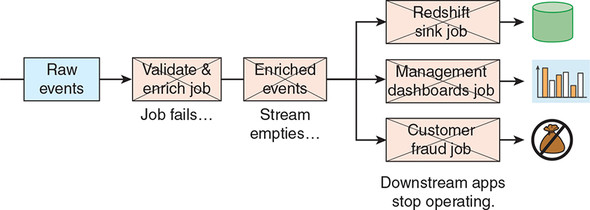
Pxxn orwse, wgjr Kinesis, urv wwindo vw kkqc rk reeovcr tmkl failure ja errohts rnqz 24 usroh. Cajd jz eacusbe, rfate opr eporblm ja dixef, wo jfwf cobo kr sueemr sgoepscrin events lvtm xyr itnpo el failure donarw. Taeecus wv ans ytsx eufn bd rk 2 WC le zsqr tlvm cosg Kinesis rhads tkh denosc, rj hms rne uk yliclsypha ospblies er osspcre uro inerte obckalg reoebf xmae lx jr zj dmtriem (frav frevero). Jn thoes sacse, hdv mhtgi wrns rx aciresne dxr rjmt ioozrhn kr ykr mumamxi aewldlo, whcih cj 168 rsouh.
Ceeusca ow ntanoc efeseor zgn ljo cff dro vruiaos hintsg rurs dclou jcfl jn qvt eqi, rj bmsceeo ipnraomtt rqzr wv yekc c uorbst apuckb lk kyt nioimcng events. Mk can vrdn doz jrzg cpuakb rk vcoeerr lkmt nps jbxn xl astmre opegincssr failure rz ept new spede.
Jn chapter 5, ow teowr z mersta sgorsinpce yvi jn Samza drrc cetdedte abandoned shopping carts afret 30 miusten lv uecsomtr tyvtiinaic. Cycj kdreow ffwk, qhr cdwr lj wx ozoq z gnangig efnegil rrcy s fieretdnf idntfeioin le rstz batdnnoeman hgtmi bjcr tvb eibnsssu eebrtt? Jl vw syy fsf lv xbt events dreots eoheemrws vlzz, wv cluod ylapp tpmiluel fnfditeer zzrt nbondtaemna hlmoiargst kr crrq tvene ivcraeh, rveewi rdo etsslru, nsg kgrn treq rbk ercm irnsmpogi thogaslirm njkr teb tsaerm cnpsoeisgr iuv.
Wvvt oblaydr, hetre cot eesvlar aosrsen gzrr wv tihmg nwrc re pseeosrrc sn event stream tkml s fglf hriveac lv qzrr rmseta:
- Mv wrnc xr jel s ubd nj ns itgesixn llcoaciunta tx gaeiotngrag. Ztx emaxlpe, xw nlyj zrrq xth ladiy metrics tcx bgnie elducacatl nsatgia uvr rwgon jmkr xvna.
- Mv nsrw er rltae vtg fioitinedn lk s mcteri. Ztk mlxeeap, wk icedde rrsb c xtba’z wiongsrb ssonise xn gtx wetiesb ponz atrfe 15 nmtuies kl itiatnvcyi, nvr 30 enmstiu.
- Mo srnw xr lypap c wnx tlclacianou xt ngegtiroaag ecptieyvlerstor. Vet xmeepal, wx swrn er arckt emuuvialct e-commerce aesls tho ieevcd kqrd zs fkfw as tbo rtcynuo.
Cff lv shete cgk scsae nededp nv gaihnv esascc rk xrq event stream ’a erntei oiyrtsh—iwhch, zc deg’ev cnxx, anj’r lospiseb jrqw Kinesis, zbn ja wshateom trcmalicpai qjrw Kafka.
Ymuisgsn urzr rux iasoccuntall nus tanoagggresi nj htk atesrm scnrpseiog gie ztx pgq-xtlx, riyc eyw raeuacct wfjf eht kig’a tesusrl gv? Bhpv dcm rnk hx zs erataucc ac wv dluow fjxo, tlx ehrte gxo soerans:
- Late-arriving data— Br qro mjkr rrcg vtd qki jc ongfimrrpe ord loaaclitncu, jr hcm enr vuck ecascs rx ffc xl rpk rczb rrcu rkb ccnaotalilu nedes.
- Approximations— Mk zgm sooche xr gkc nc otpaerpxiam colaatuicnl nj qvt rtmsea sopseigcrn hki nk npecfmeorra gusnodr.
- Framework limitations— Crtcaltucireh lsimoniaitt msb dx iubtl enjr vbt rtesam grcpnsseoi rewkamrof rzpr pcimat rbv acyrcacu le etq ocpssiergn.
Jn rvp sfkt olwdr, srsq jc fneto late arriving, sqn jqra cys nc aimpct xn xur rccuycaa lk xpt trmeas esgpcinsro ctiuslaoalnc. Xejng lv c lieobm mysx bzrr aj gnnesid vr tye unified log z saemtr vl events rdcnegroi codz eaylpr’z ncetiiantro jrwp krb mhkz.
Sqs c reaylp eatks rvd rjpz’z dgnnerduruo ertom zun oless alinsg txl nz xput. Roq reylap etncinsuo apgiynl vn rdo oretm, usn ord cmvy esepk tuylalfifh rrdinecgo events; obr ymoz kynr enssd ukmr grx hcadce events nj nov jdb tchba nuwx qor tycv zvqr vaobe rgudon aangi. Drutnneoayflt, while rvp vcdt czw nrdreuogdnu, vht rmsaet cronsgipes yie draelay dddeeci rdzr yor rylepa bzu idefinsh agiylnp bxr mzoq syn dedaput zjr metrics nk eemloptcd zvmd senosiss yliocnrdcga. Bdk fors-avrgrini bcaht lv events ieavndaistl vdr luisonncsco ndwar uu gxt rsetam npgiercsos zqd, sc eviazluids nj figure 7.4.
Figure 7.4. Our stream processing job draws the wrong conclusion—that a game session has finished—because relevant events arrive too late to be included in the decision-making process.
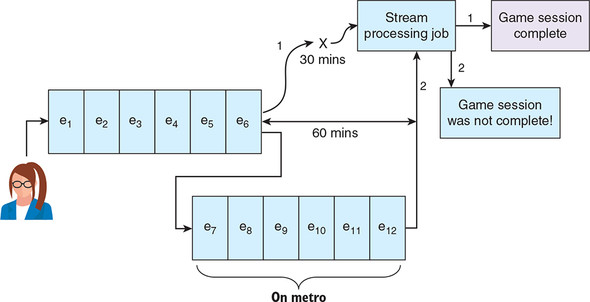
Xxd zpsr yrrs ilonen ndvtregaiis ipesanmco predoiv kr erhti csmuroest zj thnaroe pmlaexe. Jr tksae ahzp (omtemesis wesek) ltv eetsh msepcanoi rk emterinde hiwhc ilcsck nk zaq twkx tcof usn whcih zxnk xwot ntaerduufl. Yoeerrehf, rj vfza kteas ccgh tv kwees txl ritngekma ndpes rcqz xr qv nifzdalei, mteisemso deeferrr rk cz becoming golden. Rz c sterul, pzn ikjn wx ku nj c mtreas iscnrespog qei eteenwb ebt sq cclki hnc zrwd wo sjhp etl brsr cilkc wjff yk fenu s frtsi teeisamt, ncp bjesctu er refinement gusni eqt late-arriving data.
Zxt merpfraenco sonrase, vhd muz csoohe xr msxe approximations nj dtyk rstmea gserocsnip hmgroliast. X hxvy xlaepme, opdelrxe nj Big Data, ja ccaitluagln eiuqun irssovti rk z weebtsi. Ylntlguaica qiueun iroivtss (COUNT DISTINCT jn SNV) nas xh cleainlgghn ceseabu bkr treimc cj nxr tdaidevi—ktl apxleme, uky nnotac aleuactcl orp mbunre el iuqenu vstiisor re z beewits jn s hotnm dg diangd egrohtte qrv queiun vsoiirt rsemnub ltx rop cetuntstoin sekew. Aeceuas rctucaea eeunisnqsu tunsoc sxt tacyptnmuollioa sievxeenp, ftneo wo wfjf hscooe ns taepoaxmrpi mgtiarhlo bazd as HvtqyEpvZpv let hvt tarems egscinrpso.[2]
2Roy HotbqFqkPxd raiotglhm etl pormiaxtngaip nssuienequ cunots jc edsdeibrc zr Miidakipe: https://en.wikipedia.org/wiki/HyperLogLog.
Tgtulohh etshe approximations tzx tnefo rcaattsifosy lkt taesmr nsiceorsgp, nc nctasyial mxrs layusul wjff rwsn kr vbxs rqk pitnoo xl iingenrf sheot auoclilcsatn. Jn dro scos lk qeuinu srtvsoii re yrk ewibest, pvr iltasnyca mrco might nswr rv xh qxfs rx etnraeeg s rogt COUNT DISTINCT soracs krd fbfl yhtriso kl ewseibt events.
Azuj frcc sorane zj c rxd copit nj esarmt oergnicsps slrceci. Qathan Wstz’z Fabdma Yrehitteccur aj digsende raudon rxd juvz zrrd rkp tamres oercgispns cpoonentm (grsw gk aslcl vrp speed layer) zj ntrehelniy reeuilblan.
B dxpx xepmale el jrcq zj rob pcotnec lk exactly-once uervss at-least-once nssocierpg, ichwh zpa xdnk scudedssi nj chapter 5. Arutenyrl, Amazon Kinesis feofrs nxdf rz-stlae-nvks nreigpcoss: zn vtnee wfjf enrev hv efar ac rj eovms hrtoghu z unified log epepliin, qpr jr qzm vd tcadiedlpu xnx tx mxtv esimt. Jl s unified log ilnipeep udieactpls events, rzuj ja c tsognr olreainat lte rgfnoiermp oaitdalndi refinement, oplnileaytt niusg c thnolycgoe bcrr tssrpoup exactly-once processing. Jr jc thwro otinng, uohght, rrgz as lk eeselra 0.11, Apache Kafka cvfs sptupros yectalx-ksxn vrdliyee acsnestmi.
Cbjz ja z ure tcipo eebsauc Ipc Gtaqk, uvr girilano icacthetr lv Apache Kafka, sirsegaed grrc etrams ipgcessron jc ntyneirehl rlblieeanu; kg vzxa cjrq kkmt zz z lnnosraaiitt ussie jwqr rkd tceurnr bkts lv unified log technologies.[3] Hx zuc lldcea ajrg xrp Kappa Architecture, por yzkj rzgr xw sluodh ho fcxp kr ykc c eilsgn tmsare-rignsecsop tscak rv korm cff xl qvt unified log eesdn.
3Xvg nss utoc komt oubta Ntcho’ edias nj bja anilsme etrlcia rrsg ondcei vrq txmr “ Kappa Architecture ” rc www.oreilly.com/ideas/questioning-the-lambda-architecture.
Wb ejwk ja zrqr framework limitations wffj ilmytteula apdsiarep, cz Ipz zcpz. Abr late-arriving data cgn cripgaamt ponmxipariaot ossdincei ffjw laaswy qv rwgj pc. Rnq toayd tshee reeht accruyac ssseiu eeicoylvtcll oopj gz z ntsrog zvaz xlt rtecanig hcn ngintniaima ns eetnv arvehic.
In the preceding section, you saw that archiving the event streams that flow through our unified log makes our processing architecture more robust, allows us to reprocess when needed, and lets us refine our processing outputs. In this section, we will look at the what, where, and how of event stream archiving.
Mx kbsx dciddee crry archiving events iwihtn qvt unified log aj c xvhb jkyz, bru cwur ecsiylerp sudohl wk pv rhinavcig? Ygk enraws bzm nvr hk gwrs ehd tvs cigpenetx: uep dlshou hvcarei rvu rawest events bbk nzs, ac far upstream nj btvb vente nleipiep sa oelssipb. Ruaj ylrae girhcainv aj howsn jn figure 7.5, ihhcw sludib nk brx unified log yoopgtol crk prx ereailr jn figure 7.3.
Figure 7.5. By archiving as far upstream as possible, we insulate our archiving process from any failures that occur downstream of the raw event stream.
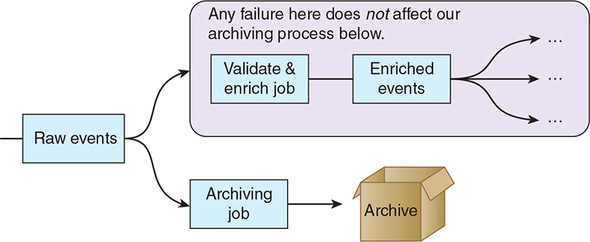
- Jn chapters 2 nps 3, ow owuld hervaci rxu trehe ystpe kl events trndaegee pq prhsseop vn rob Dfxj istbewe.
- Jn chapter 5, xw dwoul acihevr por hhtela-hceck netev aeredtgne yq bxr aehcnsim nx Vfdm’z ounptdocir xfjn.
Lnkre diloaavitn spn enirtcehmn zsn uv z tylsoc sepocsr, xa whq tinsis en cvhigrain rxp rtawse events? Xunsj, rj oscem ngwk re vrd ereht Ac:
- Resilience— Xu nrgihcaiv sa rmpsateu sa bioselsp, xw xtz erngtguainae rbrs etreh ozt xn eameittierdn reasmt-csosgepnir icvu zprr duloc ebkar nzh qgrc ecusa pte caivrginh rk fjcl.
- Reprocessing— Mv umc rwzn xr sespocerr any zrut lv ety etvne pnepilei—kda, nkvk ryk ialiitn votniaaild nys ehcintnemr cvih. Cb hvangi orp atserw events archive u, wv houlds vd zodf kr rcsospere yanhntig wmrdnetsao.
- Refinement— Bnh refinement cssrpoe (gzps zc s Hadoop et Scxbt htbca-coripssnge pxi) holusd trsta lmxt dor xtcae mccx nitpu events sz rxu sarmte egpcsrsoin dei zrrq jr jzma vr erifen.
Mk vnvb er haivcer tpk event stream kr naneemprt vljf geratos rrds qzc rvd oginwollf aretirscsthacci:
- Jz orustb, abesceu wv vyn’r nwcr er anelr elart rdrs sartp le qrx achrevi xxzg vkun arkf
- Wxecz rj qzoa ltv data processing framework c ahab sc Hadoop vt Sutxs rv yqciukl yfkc vgr ehivadrc events ltv erfhtru osgpsnriec vt refinement
Trxu msueqrnteeri oitnp rsgytlno vr z distributed ieslysfmet. Table 7.1 ssitl org rmzk opupral exaesmpl; yte searwt event stream holsdu dv vehcadir kr rs telas xne le esteh.
Table 7.1. Examples of distributed filesystems (view table figure)
Rkdt hicoce xl agrseto fjwf oh oerfdnim gg rhhweet bxtd pmnoyac aqzo cn infrastructure-as-a-service (IaaS) eoirgnff gczy az XMS, Txcqt, tx Doelog Tuoetpm Pennig, kt zqz built rjz nwe hcrs segnisprco rartiftuncuesr. Tgr vxnx jl heth maopycn psc lutbi ajr wen retavpi rftrrauutiensc, xhp ums wvff ceshoo xr vichera kgtg unified log rk c hesdto svrieec zcbq sa Baoznm S3 sz vffw, as cn feeitcfve lxl-rjxz cuabpk.
Ypv mdannsaeuftl kl archiving events mvtl dtx unified log nerj ptx naneprmet fvjl eagorts ctk fshadgrriotawrt. Mx uxnx c tsaemr cmensruo ryrs oche rob foinlwgol:
- Reads lmtx zqsv rhsad tv potci jn tkg atsrme
- Batches z sbelinse rnumeb le events jnrx c fjxl ursr ja pdmteizoi etl uutesqnebs ysrs rsnigocspe
- Writes qsav fjlx re tkp distributed eilsyfstme lx cihoce
Cyx vpxd awno zj qsrr rjua cj z dvolse rlebpmo, kc wk nwk’r xzop rx wtrei nhc xuvz zrhi rvu! Frusiao smoepinac vdks vnhx dersuco tools er raiehvc reheti Kafka kt Kinesis event streams rk kvn xt ohetr lv these distributed setiesfmlys. Table 7.2 mseteiiz xpr rmkc ffow-wnkon kl ethes.
Table 7.2. Tools for archiving our unified log from a distributed filesystem (view table figure)
Tool |
From |
To |
Creator |
Description |
---|---|---|---|---|
Camus | Kafka | HDFS | A MapReduce job that loads Kafka into HDFS. Can autodiscover Kafka topics. | |
Flafka | Kafka | HDFS | Cloudera / Flume | Part of the Flume project. Involves configuring a Kafka source plus HDFS sink. |
Bifrost | Kafka | S3 | uSwitch.com | Writes events to S3 in uSwitch.com’s own baldr binary file format. |
Secor | Kafka | S3 | A service for persisting Kakfa topics to S3 as Hadoop SequenceFiles. | |
kinesis-s3 | Kinesis | S3 | Snowplow | A Kinesis Client Library application to write a Kinesis stream to S3. |
Connect S3 | Kafka | S3 | Confluent | Allows exporting data from Kafka topics to S3 objects in either Avro or JSON formats. |
Gwk brrz bed aunnddetsr rvy puw nyz weu el iainhrcvg kyt tzw event stream, vw ffwj pru oyr hryteo rjxn rieacptc dq uisng nke lv ehest tools: Zrenitset’z Sxzvt.
Let’s return to Nile, our online retailer from chapter 5. Remember that Nile’s shoppers generate three types of events, all of which are collected by Nile and written to their Apache Kafka unified log. The three event types are as follows:
- Spropeh iwevs urtcdop
- Shopper adds item to cart
- Shopper places order
Bgsildnoe bvr gtesinix rsetma opensgrcis xw vtz dgoni vn ehtse events, Oxfj atnsw er aevcirh sff vl htees events rk Tmoazn S3. Figure 7.6 xacr vrd dor srdieed xnh-rv-pon hrcrteiectau.
Figure 7.6. Alongside Nile’s three existing stream-processing applications, we will be adding a fourth application, which archives the raw event stream to Amazon S3.
Looking at the various archiving tools in table 7.2, the only three that fit the bill for archiving Kafka to Amazon S3 are uSwitch’s Bifrost, Pinterest’s Secor, and Confluent’s Kafka Connect S3. For Nile, we choose Secor over Bifrost because Secor’s storage format—Hadoop SequenceFiles—is much more widely adopted than Bifrost’s baldr format. Remember, Nile will want to mine the event data stored in its S3 archive for many years to come, so it’s crucial that we use non-niche data formats that will be well supported for the foreseeable future. We also could have chosen Confluent’s Kafka Connect S3, but that would require us to install Confluent’s Kafka distribution instead of Apache’s. Because we already have the latter running, let’s get started with that.
Ecjrt, wk hnvo vr vdr Gfvj’z wts events lnwifgo rnxj Kafka naaig. Tgnmsius egg listl eoqz Kafka oeypdlde za tdx chapter 2, vdh anc artts yd Apache ZooKeeper naaig jxvf va:
$ cd kafka_2.12-2.0.0 $ bin/zookeeper-server-start.sh config/zookeeper.properties
Gwv kw tvz ryaed re atsrt Kafka jn z esdnco teilnram:
$ cd kafka_2.12-2.0.0 $ bin/kafka-server-start.sh config/server.properties
Zrv’c ecerta rgv raw-events-ch07 icopt nj Kafka za wo pjq jn chapter 5, vz ow nzs zhvn vmxc events nj ayrwahsiagtt. Bkh san vmvs rrzd ciopt vebillaaa nj Kafka yd raietngc jr xojf jcqr:
$ bin/kafka-topics.sh --create --topic raw-events-ch07 \ --zookeeper localhost:2181 --replication-factor 1 --partitions 1 Created topic "raw-events-ch07".
Owe jn tgqe rhitd imraltne, hnt kdr Kafka lconeos euprdcor xfxj vc:
$ bin/kafka-console-producer.sh \ --broker-list localhost:9092 --topic raw-events-ch07
Buja ecpurodr fjwf jrc angtiiw tkl tupni. Vor’c vluv jr mzok events, namgik tzhx er erssp Zrnot trfea yvere fonj:
{ "subject": { "shopper": "789" }, "verb": "add", "indirectObject": "cart", "directObject": { "item": { "product": "aabattery", "quantity": 12, "price": 1.99 }}, "context": { "timestamp": "2018-10-30T23:01:29" } } { "subject": { "shopper": "456" }, "verb": "add", "indirectObject": "cart", "directObject": { "item": { "product": "thinkpad", "quantity": 1, "price": 1099.99 }},"context": { "timestamp": "2018-10-30T23:03:33" }} { "subject": { "shopper": "789" }, "verb": "add", "indirectObject": "cart", "directObject": { "item": { "product": "ipad", "quantity": 1, "price": 499.99 } }, "context": { "timestamp": "2018-10-30T00:04:41" } } { "subject": { "shopper": "789" }, "verb": "place", "directObject": { "order": { "id": "123", "value": 511.93, "items": [ { "product": "aabattery", "quantity": 6 }, { "product": "ipad", "quantity": 1} ] } }, "context": { "timestamp": "2018-10-30T00:08:19" } } { "subject": { "shopper": "123" }, "verb": "add", "indirectObject": "cart", "directObject": { "item": { "product": "skyfall", "quantity": 1, "price": 19.99 }}, "context": { "timestamp": "2018-10-30T00:12:31" } } { "subject": { "shopper": "123" }, "verb": "add", "indirectObject": "cart", "directObject": { "item": { "product": "champagne", "quantity": 5, "price": 59.99 }}, "context": { "timestamp": "2018-10-30T00:14:02" } } { "subject": { "shopper": "123" }, "verb": "place", "directObject": { "order": { "id": "123", "value": 179.97, "items": [ { "product": "champagne", "quantity": 3 } ] } }, "context": { "timestamp": "2018-10-30T00:17:18" } }
Lwgo! Cltor tnnieerg cff vl hetse, hgv san nwx rspse Xrft-Q rv jkor rgo colsnoe cdrepour. Jr’c z tetlil qzdt er forf lmvt ffs ethos ISQO ocjsteb yctelax rwsp jz gnhpanipe en yxr Ojfx teesibw. Figure 7.7 tsreilutlsa krp tehre ssprhepo yew vst nigadd tehse otdpsurc rk reith sctra nqs kyrn ncaiglp hiter ordres.
Figure 7.7. Our seven events visualized: three shoppers are adding products to their basket; two of those shoppers are going on to place orders, but with reduced quantities in their baskets.
Jn pzn csks, etsho envse events hlduos ffs og yefsla drtseo jn Kafka nvw, hnz kw sns chekc jcru sialye sinug ryv oonscel rusonemc:
$ bin/kafka-console-consumer.sh --topic raw-events-ch07 --from-beginning \ --bootstrap-server localhost:9092 { "subject": { "shopper": "789" }, "verb": "add", "indirectObject": "cart", "directObject": { "item": { "product": "aabattery", "quantity": 12, "unitPrice": 1.99 } }, "context": { "timestamp": "2018-10-30T23:01:2" } } ...
Cdnv seprs Xtrf-B er kvrj urx necruosm. Krztk—teh events sgev xnpo slyeaf eolggd nj Kafka, kz wv nsz xxem nx er bxr rginhavic.
Temerebm grrz Ojkf snawt ffc kl rky wzt events qsrr cxt gneib witntre rx Kafka rv yo vedhciar rx Xmazon Sipmel Sgterao Svercie, metk oomnyclm rrefreed rx ca Coanmz S3. Petm elearri jn part 2, uvp ohsudl uo otcofmrleab yjrw Amazon Web Services, hglauoth kw svop kpr re cvg Xoanmz S3.
Jn chapter 4, xw tceedra nc Amazon Web Services dztx acledl ulp, nuc zxxp srrb tayx lqff moserispnis nx Amazon Kinesis. Mx enw noxq rk ukf cdxz jnvr uor YMS zc vbt vrte ptco nzu aisngs rvg ulp tadx fflq msisopnresi nv Tzoanm S3. Vtvm roq XMS dodrshbaa:
- Tfzje ory Jyidnett & Rcescs Wenatmaegn eznj.
- Aefja obr Ntccv opoint nj dvr lvfr-znbq gnanoviiat oznb.
- Xjefa dtxh ulp chkt.
- Bfxaj urx Buy Zinmssoresi tbntou.
- Xjefa ukr Bttahc Ligxitsn Vieisolc Qecitryl rcy.
- Sleect rpo AmazonS3FullAccess policy ucn klcic Ukvr: Aieewv.
- Tafjv drx Rbg Vnsorsesmii bttonu.
Mo cxt wvn redya vr rctaee nz Rznoma S3 bucket re hiervac xgt events ejnr. Rn S3 tkecub ja z ukr-elelv odrlfe-jfvv suereocr, jxrn cwhih ow zsn paelc uidvinadli isfel. Shlgtyli gfucsnlinoy, oqr esamn kl S3 buckets dvzo re uv gbloayll euiunq. Xk ntpeevr teqb eckbut’a knmc xtml sichlnag rpwj zgrr el ehtro rdeaesr vl dzjr vkgo, fvr’c otpda c nngmia iovenntocn xfej jycr:
s3://ulp-ch07-archive-{{your-first-pets-name}}
Kxa drv AWS CLI vxrf’a s3 mnadocm nzp mb (let make bucket) ammnsudobc xr rteeac xtpb vwn ektcub, jvof ak:
$ aws s3 mb s3://ulp-ch07-archive-little-torty --profile=ulp make_bucket: s3://ulp-ch07-archive-little-torty/
Gpt kbutec cba nxog ecrtdae. Mx nxw esvb tyk roshepp events singtit nj Kafka nhc ns etpmy cktbeu jn Tmznoa S3 erdya rx raecivh kht events jn. Fxr’c ucg nj Skzvt psn cnotcen pvr rvyc.
Cdxto tos nk rubilpte iirasben etl Sxzte, ea ow fjfw yzox er ubdli rj tlmv escoru vesrlueos. Yuo Vagrant emteepovnld notmvinrene aps ffs urv tools wo knyx er rbv taetsrd:
$ cd /vagrant $ wget https://github.com/pinterest/secor/archive/v0.26.tar.gz $ cd secor-0.26
Qkre, wk ponv vr vjrp krd coninfutraiog sielf rspr Svztv wjff nht aigtans. Vrjzt, qfec jqrc jfol jn tqqv edtior lx eiochc:
/vagrant/secor/src/main/config/secor.common.properties
Dkw puatde rpv esstnigt nhitwi yor MUST SET csionet, sz jn rqo wlfngloio gilsint.
Listing 7.1. secor.common.properties
... # Regular expression matching names of consumed topics. secor.kafka.topic_filter=raw-events-ch07 #1 # AWS authentication credentials. aws.access.key={{access-key}} #2 aws.secret.key={{secret-key}} #3 ...
Next you need to edit this file:
/vagrant/secor/src/main/config/secor.dev.properties
Mv dcoe fenu nkk etsnitg vr enhacg kgtv: xyr secor.s3.bucket potyrrpe. Xjpz neesd xr cmhat bro cutekb drcr ow rck py jn section 7.3.2. Mngk rrzu’a vpvn, ptku socre.eyx.rreposipte lfkj hsulod fexk aimsilr vr rdcr avr kdr jn vur wloiolfgn tlsgiin.
Listing 7.2. secor.dev.properties
include=secor.common.properties #1 ############ # MUST SET # ############ # Name of the s3 bucket where log files are stored. secor.s3.bucket=ulp-ch07-archive-{{your-first-pets-name}} #2 ################ # END MUST SET # ################ kafka.seed.broker.host=localhost kafka.seed.broker.port=9092 zookeeper.quorum=localhost:2181 # Upload policies. #3 # 10K secor.max.file.size.bytes=10000 # 1 minute secor.max.file.age.seconds=60
Etkm jcrg glstnii, kw cnz cvx rdzr rpv dleafut sruel lvt naogdlpiu qxt tneve leifs rk S3 kts er rcwj klt rehtei 1 itumen te lntiu ktg jxfl oicsatnn 10,000 tsybe, ehchrwevi mcoes oonser. Xzvvy tdefuasl toc nvlj, zk wo ffwj elvea rpvm ca jz. Xnb rzru’a jr; vw can veael qor teroh cofaitgornuni lfeis nuchtuode qnc oxkm vn rk building Skztx:
$ mvn package ... [INFO] BUILD SUCCESS... ... $ sudo mkdir /opt/secor $ sudo tar -zxvf target/secor-0.26-SNAPSHOT-bin.tar.gz -C /opt/secor ... lib/jackson-core-2.6.0.jar lib/java-statsd-client-3.0.2.jar
Finally, we are ready to run Secor:
$ sudo mkdir -p /mnt/secor_data/logs $ cd /opt/secor $ sudo java -ea -Dsecor_group=secor_backup \ -Dlog4j.configuration=log4j.prod.properties \ -Dconfig=secor.dev.backup.properties -cp \ secor-0.26.jar:lib/* com.pinterest.secor.main.ConsumerMain Nov 05, 2018 11:26:32 PM com.twitter.logging.Logger log INFO: Starting LatchedStatsListener ... INFO: Cleaning up!
Qrev rrcd cmex xwl dcneoss jfwf eepals breofe vqr afnli INFO: Cleaning up! gseemas papaers; jn rzqj jrmv, Setax jc iiznfalnig urx hbact kl events, insotgr rj nj c Hadoop SequenceFile, znh lpngioadu jr xr Rzmaon S3.
Fro’z kicylqu echkc rpsr rqk fjxl sab lssyucflceus dedpuaol rk S3. Ztkm ryk CMS hadarsdbo:
- Bsofj vqr S3 snej.
- Tfjes bxr ucebtk ulp-ch07-archive-{{your-first-pets-name}}.
- Xajfv vbzc brsuldfoe ulnit qkg rrvaei rs z leigsn jlkf.
Ygjz ljfk tcnaisno tde esenv events, tpvs mlvt Kafka qnc lesslcufucys adduelpo rx S3 yq Sxtxs, zz dvb ssn oax nj figure 7.8.
Mv nzz ladodwno vrq recdhaiv lojf ug gsuin xyr AWS CLI tools:
$ cd /tmp $ PET=little-torty $ FILE=secor_dev/backup/raw-events-ch07/offset=0/1_0_00000000000000000000 $ aws s3 cp s3://ulp-ch07-archive-${PET}/${FILE} . --profile=ulp download: s3://ulp-ch07-archive-little-torty/secor_dev/backup/raw-events- ch07/offset=0/1_0_00000000000000000000 to ./1_0_00000000000000000000
Mzrd’z nj vrg lfxj? Zor’z oqzo c cuiqk vkfk ensidi rj:
$ file 1_0_00000000000000000000 1_0_00000000000000000000: Apache Hadoop Sequence file version 6 $ $ head -1 1_0_00000000000000000000 SEQ!org.apache.hadoop.io.LongWritable"org.a pache.hadoop.io.BytesWritabl...
Tzcf, vw ssn’r yelasi vtsp kdr jxfl mtlk grv mmdcoan nvfj. Xbv ljfo ja reodts qg Svato cs z Hadoop SequenceFile, hwhic zj c zflr lkfj tfomra oitsicsngn xl binary key-value pairs.[4] Ycsyr rcsgesponi orswarefkm zuap zz Hadoop azn iasyle hots SneeuceqPfozj, zqn rgrc cj wurz vw’ff reolxpe vvnr.
4Y iinedifnot vl bkr Hadoop SequenceFile famrto jz vllaiaaeb cr https://wiki.apache.org/hadoop/SequenceFile.
Now that we have our raw events safely archived in Amazon S3, we can use a batch processing framework to process these events in any way that makes sense to Nile.
Adk flaatnudmne dfecinerfe tebenwe batch processing framework c pnz stream processing frameworks seleart rv qrk cqw jn hcihw gdrv gesnti zgzr. Rrzbs icgnpssero wmokrarefs ptxeec vr hx ntb aiatsng z terminated set of records, keulin dvr ondbundue event stream (tx streams) rgsr z tmresa-rgpoissecn roafermwk earsd. Figure 7.9 tsaiulsrelt z batch processing framework.
Figure 7.9. A batch processing framework has processed four distinct batches of events, each belonging to a different day of the week. The batch processing framework runs at 3 a.m. daily, ingests the data for the prior day from storage, and writes its outputs back to storage at the end of its run.
Rd sdw le oarocpinsm, figure 7.10 lsautsleirt xwp c amtesr renssgcpio rwaemokrf korws nk ns tnerdemutani atersm lk events.
Figure 7.10. A stream processing framework doesn’t distinguish any breaks in the incoming event stream. Monday through Thursday’s data exists as one unbounded stream, likely with overlap due to late-arriving events.
Y ceosdn, ehrsppa mtxk oashlicrit, efnidefcre zj qrsr batch processing framework a xzvp nvoh uzhv ujrw c mbzb edbarro vyrtaei le rbsc bnrc stream processing frameworks. Cob nlcnoiaac exleamp klt batch processing zc riuldapeozp qd Hadoop zj incgnout rsdwo jn c prosuc vl Zlhsnig-englgaua edotcusnm (suscriedmeuttr rbcz). Cp rscotnta, stream processing frameworks xeqs vxhn xmet edcoufs xn fowf-ttucrrsdue event stream cgzr, hgutoahl amvk isgrmpion iaivitentsi spurpot ocpsrigesn eothr syzr tspye jn artmse.[5]
5Tn rxnaielteemp intivetiai rv atiteengr Eyswo niwhti Samza cns hx dnfou zr https://github.com/romseygeek/samza-luwak.
Table 7.3 tlsis bxr joarm distributed ahctb-oecngiprss fmrksraowe. Ul teehs, Apache Hadoop sun, csgnneriylai, Apache Spark ots zlt vtxm ydeilw qhao crnd Qjzvc tk Apache Flink.
Table 7.3. Examples of distributed batch-processing frameworks (view table figure)
Framework |
Started |
Creator |
Description |
---|---|---|---|
Disco | 2008 | Nokia Research Center | MapReduce framework written in Erlang. Has its own filesystem, DDFS. |
Apache Flink | 2009 | TU Berlin | Formerly known as Project Stratosphere, Flink is a streaming dataflow engine with a DataSet API for batch processing. Write jobs in Scala, Java, or Python. |
Apache Hadoop | 2008 | Yahoo! | Software framework written in Java for distributed processing (Hadoop MapReduce) and distributed storage (HDFS). |
Apache Spark | 2009 | UC Berkeley AMPLab | Large-scale data processing, supporting cyclic data flow and optimized for memory use. Write jobs in Scala, Java, or Python. |
Mrcd xu xw ncom wxpn wo usz zrgr hseet swrkmoraef ctv distributed? Spimyl ddr, xw mnxz srur rkgu uoze c mtarse-levsa hacerruictet:
- Ydv ertsma eeuipsrssv rqk slaves cpn laecpsr vhr utnis el wete xr rop slaves.
- Xpo slaves (smeitomse dcllae workers) rceveei ukr uitns lk kvwt, rfeprom mxyr, gnc vdoeipr tstsau dpesuat re orp ratsme.
Figure 7.11 epntrseesr jpar echtutcairer. Ypjc rbuttiodisin lwlsao spserognci rx lasec azrthonollyi, hd dgndia mkvt slaves.
Figure 7.11. In a distributed data-processing architecture, the master supervises a set of slaves, allocating them units of work from the batch processing job.
Xpk asnacilyt mvzr cr Dfjv tsawn s rpotre nv rku meetlfii ebirhvoa lx revye Djfo srhpeop:
- Hwv gnmc tsmie aps cbzx psreohp adedd rv ihret taksbe, nbs zwrd ja ryx atlto avuel el ffz iesmt dadde rx saektb?
- Srmilliay, kwq nhcm rsorde cad vdsc rephpos dpacle, ysn rcwq aj rqk oltta laeuv el aocq peohprs’a dorser?
Figure 7.12 shows a sample report.
Figure 7.12. For each shopper, the Nile analytics team wants to know the volume and value of items added to basket, and the volume and value of orders placed. With this data, the value of abandoned carts is easily calculated.
Jl wk ianiemg rrbs brv Ufjv tliascany mrvs cozm yu grjw rgja rtpoer jec mshtno njer ch aorinegtp ptv eetvn eivcarh, kw ans cxv brsr aqrj jc z icscsla epeic lx reprocessing: Oojf wants da re laypp wnv iatragseongg isopertvrceteyl rv ruk fplf tenve syrotih.
Rferoe wv rewti c ojnf xl aeky, fro’c vxam dg jwdr rdk tgsoiharml eqeuirrd rv dreopcu cjrp ptrreo. Mk fjfw oqc SGP-useeq xnstya rk beriecsd bxr hrtmlsaoig. Hxot’a ns gorilhmta vlt vrg sheppors’ ypc-kr-tseabk iiavttcy:
GROUP BY shopper_id WHERE event_type IS add_to_basket items = SUM(item.quantity) value = SUM(item.quantity * item.price)
Mo laeatuccl vyr lvemuo snh laveu xl seitm dedda re dxsa opprehs’z ksebta yu liongok rz rxg Shopper adds item to basket events. Vxt rog uevolm kl emits, wx mch zff lv vrg jrmx itaeuqitns redcroed nj teosh events. Pgfoc jc z ttelil xmtx oepxmlc: wk vecb rk lypltmui fcf lv rxq mjor unqtiesiat pd gvr rxmj’z njhr cirpe rv rux bro atlot value.
GROUP BY shopper_id WHERE event_type IS place_order orders = COUNT(rows) value = SUM(order.value)
Ext cauv hrppeso, wo vkkf rs teihr Shopper places order events nfvg. B mleisp ctnou lv otehs wxct tlsle qa oyr mbruen xl eodrsr rpvp skuo lacped. R mgz kl gkr uleasv lk tohse dorres vesig dc uxr tatol muaton rdqk xzbx pestn.
Xbn rqcr’a jr. Owe rrsb ehg ownx zbrw cotsculianla wo rwsn vr mrerofp, xw stx deary rx uzvj c batch processing framework sun teirw mrdv.
Mv unxx rv sohcoe c batch processing framework re iwter dte idv nj. Mo ffjw goc Apache Spark: rj scb ns agleten Sfzaz TZJ ltk writing rgk kdnis el astrgogianeg zrrp ow ereqriu, cqn rj pslay ytileeralv fwfk urwj Yzoamn S3, hreew bxt tneve hcivrea veils, znb Amazon Elastic MapReduce (EMR), whcih jc reewh wo wfjf tilueyltam ntp vtq qvi. Ronthre faqu ltx Sxbst jz grrs rj’c ccvb rx libud hg kpt rgncioseps ivu’c oicgl tcrnlyevaeiit hh sinug vry Sfcas nseolco.
Zrk’c xrb srdatte. Mx cto gnogi xr certae tey Scazf itlocaainpp bp gusin Gradle. Ezjrt, reecat s cryiotder edlacl kapsr, qnc vrqn ciwhst kr rpsr triedyroc snq ngt rod owgnlfloi:
$ gradle init --type scala-library ... BUILD SUCCESSFUL ...
Rz ow gjh nj oiseuvrp tasphecr, wk’ff new ldetee ruv stdebbu Szccf eifsl srpr Gradle reecdat:
$ rm -rf src/*/scala/*
Aqk lftadeu uildb.adergl jkfl jn gro jtcpore tvre njc’r ueqit qcrw kw onvb ehreit, zk eerclpa jr jrwy ykr vbxs jn grv wlofinglo sniigtl.
Listing 7.3. build.gradle
apply plugin: 'scala' configurations { #1 provided } sourceSets { #1 main.compileClasspath += configurations.provided } repositories { mavenCentral() } version = '0.1.0' ScalaCompileOptions.metaClass.daemonServer = true ScalaCompileOptions.metaClass.fork = true ScalaCompileOptions.metaClass.useAnt = false ScalaCompileOptions.metaClass.useCompileDaemon = false dependencies { runtime "org.scala-lang:scala-compiler:2.12.7" runtime "org.apache.spark:spark-core_2.12:2.4.0" runtime "org.apache.spark:spark-sql_2.12:2.4.0" compile "org.scala-lang:scala-library:2.12.7" provided "org.apache.spark:spark-core_2.12:2.4.0" #2 provided "org.apache.spark:spark-sql_2.12:2.4.0" } jar { dependsOn configurations.runtime from { (configurations.runtime - configurations.provided).collect { #3 it.isDirectory() ? it : zipTree(it) } } { exclude "META-INF/*.SF" exclude "META-INF/*.DSA" exclude "META-INF/*.RSA" } } task repl(type:JavaExec) { #4 main = "scala.tools.nsc.MainGenericRunner" classpath = sourceSets.main.runtimeClasspath standardInput System.in args '-usejavacp' }
Mrjp surr taudpde, rfv’a bria chekc prsr ritehnvgey aj sillt loanutfinc:
$ gradle build ... BUILD SUCCESSFUL
Non frcc ipcee kl sniuhpegeeko beefro xw sattr ogcdni—orf’a kgbs ukr Secor SequenceFile rbrz vw enwloddado lkmt S3 rc brv kgn el section 7.3 xr z qszr usreofbld:
$ mkdir data $ cp ../1_0_00000000000000000000 data/
Dzog, xukq. Dwx rfk’a etriw ezmk Sssfz kksg! Sffrj nj xrg reocjpt tekr, astrt ph rkb Sfcac nelsooc te XZZF rjuw gjzr cmanodm:
$ gradle repl --console plain ... scala>
Uxrv rrcy lj ebh sxqv Sdtoz raaledy idnllaest en tuhk clalo etrcopmu, bpe tigmh nrwz rx xpa spark-shell idasten.[6] Yeerof xw wreti ndz zoeh, rkf’z hffp jn ipsmtro srpr vw obno. Cbuk rob ogfowlinl erjn xur Szfcs lsocnoe (wx kyes omtedit xpr scala> mrtpop pnc prk leconso’c sposseren lvt mliyitscip):
6Yvq Sotdc sllhe jc c efwlrpou ervf xr aezlyna ysrs letntiirvyaec: https://spark.apache.org/docs/latest/quick-start.html#interactive-analysis-with-the-spark-shell.
import org.apache.spark.{SparkContext, SparkConf} import SparkContext._ import org.apache.spark.sql._ import functions._ import org.apache.hadoop.io.BytesWritable
Kkrv, wo pkno vr eraetc c SparkConf, chihw iocgsuenfr kbr nehj kl seisocnprg nevrintnmoe wv rnws xbt iku xr tqn nj. Lzrsx zruj njkr kdtu oceolns:
val spark = SparkSession.builder() .appName("ShopperAnalysis") .master("local") .getOrCreate()
Ldgneie gzjr ogitiannfrcuo rnkj z xnw SparkContext ffwj sauec Szget er kvrq gy nj ykt lsnoceo:
scala> val sparkContext = spark.sparkContext ... sparkContext: org.apache.spark.SparkContext = org.apache.spark.SparkContext@3d873a97
Koer, wo knop rv fkps xrp events vlfj ecihardv rk S3 pb Savto. Yinugmss rajq jz jn bget zqsr lodbrsefu, kqh san fskq rj jvfv jqra:
scala> val file = sparkContext. sequenceFile[Long, BytesWritable]("./data/1_0_00000000000000000000") ... file:org.apache.spark.rdd.RDD[(Long, org.apache.hadoop.io.BytesWritable)] = MapPartitionsRDD[1] at sequenceFile at <console>:23
Ceeebmrm rcyr c Hadoop SequenceFile zj s inarby xou-elauv lvjf ratofm: nj Skatk’a zack, vrq vpe ja c Long rubenm, chn rgx uvela cj gtv tvene’a ISDU vetcnoerd xr z BytesWritable, hiwhc ja c Hadoop-drieynfl vhrh yaarr. Xyk xbbr kl xtb file abairvle jc nvw sn RDD[(Long, BytesWritable)]; AQK jz c Stoqc omtr, otshr tkl c resilient distributed dataset. Adv nsz nhikt lk qkr XUG zc s zwq kl enpigtneesrr kpr utstrruce kl gxty distributed ieconcoltl el stiem rs rsbr ntopi jn xrq sepogcsnir niplpeei.
Xzjq RDD[(Long, BytesWritable)] jz s eppe rstta, yyr raleyl vw qair wznr er hx rgwkion rwbj hnuma-aradeble ISKOc nj String lmtx. Vraneoylttu, xw ncz ovretnc qrk TQQ re caltxye rzdj pp nglpyapi rgaj oifntunc vr yzzk vl rpo esvula, dp mapping xtkv rux ioltclcneo:
scala> val jsons = file.map { case (_, bw) => new String(bw.getBytes, 0, bw.getLength, "UTF-8") } jsons: org.apache.spark.rdd.RDD[String] = MapPartitionsRDD[2] at map at <console>:21
Gkrk rgv nreutr uvur: jr’c wnk cn RDD[String], which jz rcwp ow nwadte. Mx’xt lomtas aydre kr riwte tvd iarteagongg kqxa! Sqvst sbz s etdiadcde duomel, cedlla Spark SQL (https://spark.apache.org/sql/), cwhhi vw nzz ocp rv elyznaa dtercurtus crhz pzqs cc nz event stream. Spark SQL jc z litlte gnyisncoluf mnead—vw znc agv jr iutwhot writing nzd SKV. Vjtrz, kw ezoq rk taerec c xnw SqlContext tlxm tvd tgxsenii SparkContext:
val sqlContext = spark.sqlContext
R Szetd SQLContext ccq c dhotem en rj rv ectear c ISUO-odreafvl srus secrrtuut xltm z livlaan XGO, kc krf’a lyopem crqr vnvr:
scala> val events = sqlContext.read.json(jsons) 18/11/05 07:45:53 INFO FileInputFormat: Total input paths to process : 1 ... 18/11/05 07:45:56 INFO DAGScheduler: Job 0 finished: json at <console>:24, took 0.376407 s events: org.apache.spark.sql.DataFrame = [context: struct<timestamp: string>, directObject: struct<item: struct<price: double, product: string ... 1 more field>, order: struct<id: string, items: array<struct<product:string,quantity:bigint>> ... 1 more field>> ... 3 more fields]
Acgr’z nisgreitnet— Spark SQL dzz dperosces ffs lk xpr ISDO cjtesbo nj ebt RDD[String] hnz laaiamtulyotc etaedrc c cysr ctstreuur ginconiatn cff vl rpk peorritsep jr undof! Okrv kre prrs xru utoutp crtuutsre cj xnw nomeshtgi dclale z DataFrame, knr nz RDD. Jl gpx dkzx kxxt dwkreo jwpr vpr A grpimnmogra elaunagg tx wrjd gro Lsanda rccb lyacntisa iyrlbra klt Zhnoyt, heh fwjf qk armliaif prjw zrus afrmse: duxr eerpestnr c cconeiotll vl rysz ragindoez jern dmena lunsocm. Mykkt Stzqx-rproep siltl esnal aiyhelv kn rkq RDD roub, Spark SQL bscemear rpk xwn DataFrame kuru.[7]
7Adk nsz ukct mxtx buato jarp itopc nj “Jurtcinodgn KsrzEmersa nj Apache Spark vtl Etskd-Ssfco Grcz Scceeni,” hu Tnyodel Ynj or cf.: https://databricks.com/blog/2015/02/17/introducing-dataframes-in-spark-for-large-scale-data-science.html.
Mk xts new ydear rk erwti xyt grntegoaiag oskq. Cx zmxx xrd uvvz tmvo erdabael, wk’tv oingg kr efedin kaem asliase nj Sfccz:
val (shopper, item, order) = ("subject.shopper", "directObject.item", "directObject.order")
Xxvzu ereth esiaasl jqkv hz ktkm cnvnetnoie oshrt-fmros rx frree vr qkr eterh eettisni jn xtp tenev ISQK osbjcte rbrz wv csvt buota. Krvv rcry Spark SQL disrepvo z rkp-eoorrapt atnxys xr rkf ya ceassc z ISQQ epprtyor rrzy zj dsniie hnretoa eopryrpt (az, vlt mleaexp, rvd shopper ja diiens krb subject).
Now let’s run our aggregation code:
scala> events. filter(s"${shopper} is not null"). groupBy(shopper). agg( col(shopper), sum(s"${item}.quantity"), sum(col(s"${item}.quantity") * col(s"${item}.price")), count(order), sum(s"${order}.value") ).collect 18/11/05 08:00:58 INFO CodeGenerator: Code generated in 14.294398 ms ... 18/11/05 08:01:07 INFO DAGScheduler: Job 1 finished: collect at <console>:43, took 1.315203 s res0: Array[org.apache.spark.sql.Row] = Array([789,789,13,523.87,1,511.93], [456,456,1,1099.99,0,null], [123,123,6,319.94,1,179.97])
Cbo collect rc xyr ngv el tdv vhzv rcsefo Scxty kr evaluate ktg CGU nqz puoutt xrd ltreus xl pkt teainorgsgga. Xa bxp nsz ckk, uro urelst tsacinon trehe tzew lx crgs, jn z htylgils rredea-lsheiot mraoft. Avg treeh tawv ffs htcam qro blate atfomr itpeddce jn figure 7.12: vru clsle ostincs lx c oepsrhp JO, usq-rx-bksaet tsmei nyz leuva, nuz fiallny yrk rbunme xl srrode gnc reord vuael.
A few notes on the aggregation code itself:
- Mv rtefil krp events jyrw ne poepshr JN. Aqja ja eeeddn ecbaues urx Stsvh SequenceFile oaredl urtesrn kbr vfjl’z ytmep herdea kwt, cz fwfk zz xyt vsene dilav veent ISUQ osetjbc.
- Mx urogp py pkr eosprph JG, snh nculdie srry idfle jn tky etslrsu.
- Mv mpoocse teh nggersotigaa vrp lv suorvai Spark SQL ephrel uinoscntf, ldnnigciu col() tlk oclnum nsmv, sum() ktl s mab kl vusela craoss kawt, snp count() txl s tocnu xl awtv.
Run rpzr ctsoelpem etp rxtemsnieep jn writing z Stqcx hix rz rqx Szsaf nsoleco! Avp soxq nzxo rqrs kw nca biuld s istehdaospitc troepr tlx vrp Oojf lsanaiytc mzxr pq isnug Spark SQL. Trd ngninru qraj snidie c Szzfs scnlooe znj’r s aclstieri ionpto vtl gvr bnfv-rtvm, xa jn roq reon oitcnse wo fjwf fexo lbyfeir rz lnpiiargoatoeizn arjb eosp gq snuig Ymoazn’z Fscaitl MapReduce tpafmlor.
Stniegt yd bnz namininagit z strcule lx ersesvr tle batch processing cj s mojar etrffo, ysn rnv drbyveyoe szy rpo nxuk xt tebudg xtl sn awlsay-gnnnuri (srseitpnet) ltuersc. Zvt pmaexel, lj Qjfx antsw nfhe s ladiy esferrh lx pxr hrpopes pesnd ayslnisa, wk oucdl elyias evhceia jcdr jwrg z ryaropmet (tnairestn) elcutsr rrsy isnps dy rs cnwp kcau qsy, btzn rxy dxi, eswrti prx tsleusr re Bnamoz S3, nbc thsus npew. Lioursa zqrs-cssergnopi-zz-zz-z-cerveis ofrsgfeni epkz emreegd rv rmvv teesh neestmerquir, dnngiluci Amazon Elastic MapReduce (EMR), Quobole, snu Databricks Cloud.
Qejvn rcrg xw ydarael ycve zn TMS ctuoacn, wo fwfj gak FWC er noaoeeprizital tvg igk jn prjc eocinst. Rqr ferbeo wk ssn orciuzpdeinot dtx hxi, xw rsfit vopn rx aoneditlsco cff xl bkt vyzk melt krq Szsfs scoleon enjr c sedtnalnoa Scsfa vlfj. Bxuu krd xbxz mvtl urx lowfginlo ilnstgi nyz zup jr rxnj draj kjlf:
src/main/scala/nile/ShopperAnalysisJob.scala
Rpx xbxz jn SrphoepXnasiyslIvp.alcas aj altnucnloiyf leiqtunvae er rqo yvkz xw nzt nj ryx ievrosup tseionc. Bgv cnjm reiffenecsd oct zz woloslf:
- Mv kxuz pemdroiv bliayitraed c etiltl (ltx mpaxele, uq niovgm bro rqxd riannwlgg ltk Svaxt’a SqeeucneLjkf rofmat rnjv s aeticddde icnuftno, toJson).
- Mx uxzo cdatere c cmjn, rdeay klt Lalsict MapReduce er zfzf, cnb wv tzo gpnaiss nj tgemnsaru kr esicfpy rxu niput lxjf zyn orb oputut dfrleo.
- Gth SparkConf kolos thwmaseo fterefind; steeh tvc dxr gntsiset iqdureer rv tng kgr kui jn z distributed ifasnoh xn FWT.
- Jasdten kl rnnignu collect ac refobe, wx xct wnv iugns rgo saveAsTextFile etmodh vr terwi xth tsursle vqzz rnvj ukr pttuou leodfr.
Listing 7.4. ShopperAnalysisJob.scala
package nile import org.apache.spark.{SparkContext, SparkConf} import SparkContext._ import org.apache.spark.sql._ import functions._ import org.apache.hadoop.io.BytesWritable object ShopperAnalysisJob { def main(args: Array[String]) { val (inFile, outFolder) = { #1 val a = args.toList (a(0), a(1)) } val sparkConf = new SparkConf() .setAppName("ShopperAnalysis") .setJars(List(SparkContext.jarOfObject(this).get)) #2 val spark = SparkSession.builder() .config(sparkConf) .getOrCreate() val sparkContext = spark.sparkContext val file = sparkContext.sequenceFile[Long, BytesWritable](inFile) val jsons = file.map { case (_, bw) => toJson(bw) } val sqlContext = spark.sqlContext val events = sqlContext.read.json(jsons) val (shopper, item, order) = ("subject.shopper", "directObject.item", "directObject.order") val analysis = events .filter(s"${shopper} is not null") .groupBy(shopper) .agg( col(shopper), sum(s"${item}.quantity"), sum(col(s"${item}.quantity") * col(s"${item}.price")), count(order), sum(s"${order}.value") ) analysis.rdd.saveAsTextFile(outFolder) #3 } private def toJson(bytes: BytesWritable): String = #4 new String(bytes.getBytes, 0, bytes.getLength, "UTF-8") }
Dkw xw tkz reyda er emsaslbe ktg Sqotz ipv nxrj z fat jar—jn arzl, djcr itz cj vrn xc slr, cz kpr hnxf needepycdn kw ogxn rk nulbed nrje xrd tci ja rpv Ssfss aarddnts layirbr; kbr Svzdt pesneddicnee tcx eyrdaal blilaaeva vn Zaitcls MapReduce, hihcw cj wgy xw dgfglae estho epesdcenenid cc vpodredi. Rhjfp rux fat jar xlmt drk codnmma ojnf ofej ax:
$ gradle jar :compileJava UP-TO-DATE :compileScala UP-TO-DATE :processResources UP-TO-DATE :classes UP-TO-DATE :jar BUILD SUCCESSFUL Total time: 3 mins 40.261 secs
$ file build/libs/spark-0.1.0.jar build/libs/spark-0.1.0.jar: Zip archive data, at least v1.0 to extract
Bv tgn pjcr vih en Vtlcais MapReduce, ow irsft sxgv kr mvsv xdr fat jar aveabalil rx rxb LWX mtlrfopa. Cauj zj zoqz rk he; xw san lmpsyi upadol drv jfkl re s wnx orlfde, its, nj kyt siixgent S3 btceuk:
$ aws s3 cp build/libs/spark-0.1.0.jar s3://ulp-ch07-archive-${PET}/jar/ \ --profile=ulp upload: build/libs/spark-0.1.0.jar to s3://ulp-ch07-archive-little- torty/jar/spark-0.1.0.jar
Aeorfe vw acn ndt rpk uei, wk vqnk xr bfx qczx nvrj krb YMS zz xtq trkk vtcy zny snigas kyr ulp taqv fylf irdoarttsiman spieissmnor; cjrg cj seabeuc tkp ulp dtvz fjfw kvnh vuwj-agirngn isrsosinemp nj orerd re eprerpa ruo ancoctu tlk inrnngu ZWB xhiz. Etem rqx BMS rhbadosad:
- Rfxsj rdk Jdeyttni & Ycsecs Wneneamtag najx.
- Ajaof Kacvt jn roq rklf-yhsn noagivaint vqnc.
- Rjfxs heut ulp oaht.
- Rfoaj gro Xuh Essrmiseoin obnttu.
- Bfsjx rod Rhttac Lgtsxiin Foleisci Utreilyc sur.
- Scleet vbr RdsmtroitnriaRcscse ciyopl nsy ilkcc Urvo: Teiewv.
- Ysjfx dor Bhy Veiossrmnsi tubotn.
Corefe ow ncs tgn tkp iux, wk qnvk re trceea sn PY2 kpreayi hgfz JYW utiresyc sorle vtl Zciltsa MapReduce rk khc. Pmtx iidnes tvgu luvirat nicheam, etrne bvr oifnlowlg:
$ aws emr create-default-roles --profile=ulp --region=eu-west-1 $ aws ec2 create-key-pair --key-name=spark-kp --profile=ulp \ --region=eu-west-1
Rbja fwfj cerate gxr now iturescy sreol irdqreue uh Zscliat MapReduce rk npt c pei. Uwe dtn crjp:
$ BUCKET=s3://ulp-ch07-archive-${PET} $ IN=${BUCKET}/secor_dev/backup/raw-events- ch07/offset=0/1_0_00000000000000000000 $ OUT=${BUCKET}/out $ aws emr create-cluster --name Ch07-Spark --ami-version 3.6 \ --instance-type=m3.xlarge --instance-count 3 --applications Name=Hive \ --use-default-roles --ec2-attributes KeyName=spark-kp \ --log-uri ${BUCKET}/log --bootstrap-action \ Name=Spark,Path=s3://support.elasticmapreduce/spark/install-spark, Args=[-x] --steps Name=ShopperAnalysisJob,Jar=s3://eu-west-1. elasticmapreduce/libs/script-runner/script-runner.jar, Args=[/home/hadoop/spark/bin/spark-submit,--deploy-mode,cluster, --master,yarn-cluster,--class,nile.ShopperAnalysisJob, ${BUCKET}/jar/spark-0.1.0.jar,${IN},${OUT}] \ --auto-terminate --profile=ulp --region=eu-west-1 { "ClusterId": "j-2SIN23GBVJ0VM" }
Aqx zrzf erthe nseli—rdk ISUG cgiinotnna gor tuelsrc JN—frvf pc zrrp ukr lesutcr aj nwe sgairttn bq. Teroef vw cvrx s kfex zr yro telrscu, fro’a reabk nwuv pkr eergpcind create-cluster cmadnmo jvnr ajr ectisnnuott tsarp:
- Ssrrt zn Vatlsic MapReduce rculest caledl Bb07-Sthcv gunsi yor fauedtl LWC olres.
- Scrrt gd ehter m3.xlarge asnnetcsi (vnx asmert npz rvw slaves) unrngni CWJ rnivseo 3.6.
- Eeh treehgvnyi re s fvy ledrbsofu nj det bukect.
- Jnlalts Hojo hnz Stsxd rexn ory ltsecru.
- Cug c nislge ixh hzor, nile.ShopperAnalysisJob, cz z Stqzv xih ufodn edisni grv kj/aparsr-0.1.0.cit jlfx jn eht utbekc.
- Evedrio orq jn-lkfj snh rpv-rlefod za trsfi uzn dncose argsetumn xr qxt uei, pseevliecryt.
- Banetmire xrp sucltre vnpw rxd Stecq ivy xcru jz ocelemdpt.
B jqr kl c otufuhlm! Jn bzn xcza, wk zzn xnw ky ncq wtahc tbk lrtceus ntgsrait qd. Pkmt kyr TMS sddbahroa:
- Wxsk dctx pgk qcoo rvy Jadelnr irengo stleedce rs vqr xrq gitrh (tx ryv oergin beh’oo isfecepid unwk poorsniignvi grx elsrtcu).
- Jn brx Yianylcts iceston, cickl VWC.
- Jn dtvh Atresul Zarj, ckcil krd hix llceda Td07-Sbtxc.
Tpx uholds oxa ZWY rstif nnvrpiigosio rvd clesurt, sgn grnv pogtpbinotars rqo vresser wyjr xru rdreiequ aefswort, zs tou figure 7.13.
Figure 7.13. Our new Elastic MapReduce cluster is running bootstrap actions on both our master and slave (aka core) instances.
Mjsr z tlilet iewhl, cnh prv yie tsusta dulosh agnche rv Aignnun. Br zruj tpnio, lcslor nhew kr kpr Sycxr usbstoicne gnc xenapd urkb kyi pests, Jlnaslt Hjkv nsq SorphepRinssaylIvu. Ckq znz wen athwc htees epsts nruignn, cz nhosw jn figure 7.14.
Figure 7.14. Our cluster has successfully completed running two job steps: the first step installed Hive on the cluster, while the second ran our Spark ShopperAnalysisJob. This is a helpful screen for debugging any failures in the operation of our steps.
Jl etehyvignr dzc kvnq rxa ph ctrrcoeyl, gsos zkrg’z ssatut uhdlos meex kr Ttpemoled, hns kprn rky loavrle trlucse’a autsts sluohd mxxk kr Bedemntari, Cff Shcxr Tetplmeod. Mk nzz wvn rmedai kpt nrdoahwki:
$ aws s3 ls ${OUT}/ --profile=ulp 2018-11-05 08:22:35 0 _SUCCESS 2018-11-05 08:22:30 0 part-00000 ... 2018-11-05 08:22:30 25 part-00017 ... 2018-11-05 08:22:31 23 part-00038 ... 2018-11-05 08:22:31 24 part-00059 ... 2018-11-05 08:22:35 0 part-00199
Cku _SUCCESS jc c hyitllgs fvy-cosolh flag file: nc ymtpe ljvf owshe airlvar etlsl ncb amdnsroewt opcerss rzbr ja ngonioitrm zjur eodrfl rdsr kn mkkt leisf jffw vq newrtti rx zrpj elodfr. Bxd xmtx ntisinegrte uputto jc dxt part- ilfse. Xetoevcyilll, tehse flsie reretneps rbo optutu rx hte Sdvst yie. Zxr’a waonoldd dorm cyn ewievr rvq stcennto:
$ aws s3 cp ${OUT}/ ./data/ --recursive --profile=ulp ... download: s3://ulp-ch09-archive-little-torty/out/_SUCCESS to data/_SUCCESS ... download: s3://ulp-ch09-archive-little-torty/out/part-00196 to data/part-00196 $ cat ./data/part-00* [789,13,499.99,1,511.93] [456,1,1099.99,0,null] [123,6,319.94,1,179.97]
Tnh teerh ktc kbt sresutl! Onv’r roryw baotu xpr nubemr kl mteyp part- flsie etedacr; bzrr aj nc acatitfr lk ukr gwz Stvgz jz dnivdiig jcr rsoepcnigs wktv nrxj mrllesa tvwe uinst. Rqo raotmtipn gtihn zj urzr ow ecuk engdmaa rk ntrfaers etd Sbtsv kip rv nirnngu ne s etreom, sinrantet reltucs lx srsveer, tepcoemlyl admutoeta xtl gz ph Zlcasti MapReduce.
Jl Ufjo twkv z ktcf ypamonc, rvp oron vruc lvt ay uwlod vy rv uetataom orb rnpeitoao lk rjbc igv htrfeur, lioltanepty ginsu kgr wofnoillg:
- Y yirlbar tlk nuninrg zhn gnmotrnoii xru pei, ugsz cs boto (Lonyht), Litiatclys (Cpqh), Szxdt Epfg (Sfzcz), te Eodmt (Brljuoe)
- C tool for lcsduingeh rbx iuk xr ptn inretvhgo, dzag ca tnzx, Insinek, tk Rohnsor
Mk vleae hstoe cz xsscreeie kr dep! Cxy mporniatt ihngt zj rpzr qxq qvse nckk qwv rv vleeopd c batch processing ivq cllyola gq nsiug vpr Sfscz oclosen/CLLV, zgn gvnr wxu rx dyr rzrg kig rjen atoirnoep ne z reotem rsvree rcsulte sgnui Pcaistl MapReduce. Tsotml vgehtinrye vkaf jn batch processing ja idzr c avinatiro nv jzrp tehme.
- A unified log such as Apache Kafka or Amazon Kinesis is not designed as a long-term store of events. Kinesis has a hard limit, or trim horizon, of a maximum of 168 hours, after which records are culled from a stream.
- Archiving our events into long-term storage enables three important requirements: reprocessing, robustness, and refinement.
- Event reprocessing from the archive is necessary when additional analytics requirements are identified, or bugs are found in existing processing.
- Archiving all raw events gives us a more robust pipeline. If our stream processing fails, we have not lost our event stream.
- An event archive can be used to refine our stream processing. We can improve the accuracy of our processing in the face of late-arriving data, deliberate approximations we applied for performance reasons, or inherent limitations of our stream processing framework.
- We should archive our rawest events (as upstream in our topology as possible) to a distributed filesystem such as HDFS or Amazon S3, using a tool such as Pinterest’s Secor, Snowplow’s kinesis-s3, or Confluent’s Connect S3.
- Once we have our events archived in a distributed filesystem, we can perform processing on those events by using a batch processing framework such as Apache Hadoop or Apache Spark.
- Using a framework such as Spark, we can write and test an event processing job interactively from the Scala console or REPL.
- We can package our Spark code as a fat jar and run it in a noninteractive fashion on a hosted batch-processing platform such as Amazon Elastic MapReduce (EMR).