Data visualization is all around you. Sometimes, it sneaks up on you in the most unlikely places. Have you played any video games lately? I bet there was some kind of viz to show your character’s remaining health, your progress toward a goal, or a map to show you the lay of the land and maybe all the places you’d been.
Not only is it fun, but visualization can be incredibly important for the success of an individual, an organization, or even a society as a whole. In 19th-century England, Florence Nightingale turned the medical world upside down with visualizations of data she’d collected over decades of working in military hospitals. When she would present ideas to the “Powers That Be” and they would answer with, “That’s just not how we do it,” she managed to change minds only when she showed a visualization of her data.
Well-designed visualizations save lives and can turn entire industries around, but at the same time, they can also tell you how many lives you have left with your little red plumber guy before you have to restart the game all over again. ;)
In this first chapter, you’ll get a brief introduction to data visualization as a whole, followed by a brief introduction to this book, which will include the first of many reminders that knowing your audience is the most important thing to remember as you design and build any viz. From there, we’ll look at a handful of data visualization examples throughout time, and then, we’ll talk about some of the most popular tools used to create data visualization today.
If you picked up this book voluntarily, chances are pretty good that you already have an idea of what data visualization is. Or perhaps you’re stuck in a doctor’s office somewhere, and this is the only thing available to you in the waiting room (I’d love to meet that doctor!), so you’ve picked up the book as a matter of self-preservation to save yourself from boredom. Either way, I’m still going to tell you: data visualization, or data viz as most of us in the biz usually call it, is how we communicate numerical or quantitative information in a visual manner. When I’m telling people what I do, and they get that quizzical look on their faces at the mention of data visualization (it is rather a mouthful, after all), I normally tell them that I make charts and graphs for a living, and then the penny drops. In data viz, we use things such as charts, graphs, maps, and sometimes even pictures and iconography to translate numbers into visual information—they do say a picture is worth a thousand words, right? The medium can be anything you want: print, an interactive web page, a static infographic, crayons on paper, or even three-dimensional objects such as modeling clay, among many, many other things. I’m sure you could even do an interpretive dance if you felt so moved. There are various principles about what makes one viz more effective than another, but in the end, they’re still both visualizations. There are no absolute stipulations on how you do it, so the sky is the limit, and if you always keep your audience at the forefront of your mind and the center of your development process, you’ll be set up for success.
It is my greatest hope that you will find this book to be a practical, approachable, and fun guide to making beautiful and useful data visualizations that power and inform our everyday lives. However, before I tell you more about what this book is, I would like to say that this book is not a technical manual about implementing a tool or learning a coding language. We will not be walking through any coded examples, and there won’t be exercises at the end of each chapter or section: step-by-step tutorials are not the main attraction here. This book is meant to be a tool-agnostic guide about the principles of good design as they apply to visualizing data. You will be able to apply the concepts to any stage of any data visualization project on which you work, regardless of tool, size, or medium.
All that being said, you will find this book most useful if you are already working on a viz project, be it a personal gig that’s just for fun (the data viz community is teeming with like-minded nerds who gleefully spend their free time making charts and graphs) or something you’re making for work, which really can be just as fun. The first part of the book (chapters 1-3) serves as a foundation on which the other two parts will build, so I recommend you read that first (it is, after all, at the beginning). In the second part, chapters 4-7 each delve deeply into some aspect of viz, so we can understand the context of how it works and why it’s important, and then each wraps up on the more practical side, applying those design principles to our actual work of visualization. In the last part, chapters 8 and 9 detail the entire process of building a data visualization from start to finish and then present some tips for troubleshooting and how to handle projects that go sideways. I’ve tried to write this book in a way that lets you get a lot out of it no matter where you are on your viz journey, to the point that you could skip around and not read it in order, but it would still behoove you to read chapters 1-3 first.
The single most important thing you need to keep in mind when making a visualization is to know your audience. It’s all about them, and don’t ever forget it! You could make the most beautiful visualization that makes use of all the latest tricks and the trendiest charts, obeying all the best practices, but if it doesn’t meet the needs of your audience, then you have completely wasted your time. Part of meeting those needs is that the audience truly understands the work, the message, and the story you’re trying to tell. I can’t stress it enough: no matter how much blood, sweat, and tears you pour into your work, none of it matters if your audience doesn’t understand. Save yourself a ton of grief and talk to them early and often to find out what they need. Check in with them throughout your development process to make sure you’re addressing their questions and that you’re not making features that are too complex for them to grasp quickly.
To put a finer point on it, there’s knowing who your audience is and knowing who your audience isn’t. Have you ever tried to go out to eat with your entire family? If so, you probably shudder to remember how difficult it is to decide which restaurant or bar to bless with your patronage. Mom wants Italian, Dad wants “good ol’ meat and potatoes,” your siblings want something interesting like Ethiopian or Thai, and you just want to eat, for crying out loud. Whatever you wind up choosing is not going to please everyone—even if you wind up finding a unicorn of a restaurant that serves every cuisine under the Sun, they probably don’t do any of those dishes extremely well, so everyone is still disappointed. If you try to please everyone, you will wind up pleasing no one.
Even as I write this book, toiling away during my free time—honestly, much of which would otherwise have been spent bingeing TV shows on my favorite streaming services—the need to know one’s audience is still inescapable. My vision of who you are is that you’re an analyst or someone in an analytics-adjacent field who finds that they need to create visualizations but don’t have the design know-how to do so. No matter how hard or long I work, how eloquent my prose, how beautiful my examples, or how many people I pester to let me reproduce their gorgeous visualizations, none of it matters if that hypothetical analyst doesn’t learn anything. Always put the needs of the audience above your own.
Now, with that in the back of our minds, we’re going to take a quick trip through time to see examples of how data visualization evolved, and then we’ll dive into some of the most popular tools used today to create vizzes.
To spare you a full history lesson, we’re going to instead flip through a metaphorical photo album of some ground-breaking visualizations throughout history. Being familiar with the history of a discipline gives us context for the developments and advances we have or haven’t made by now, and many often find it inspiring in their current work.
Although it doesn’t quite predate human language the way art does, surprisingly, data visualization has about as lengthy a history as astronomy. In fact, the Lascaux Cave paintings in southern France are some very early cave drawings that date all the way back to the Paleolithic era. According to Martin Sweatman and Alistair Coombs in their 2018 article about ancient knowledge of the precession of the equinoxes in the Athens Journal of History, some of the paintings just might use constellations to encode the date of an “encounter with the Taurid meteor stream.” Figure 1.1 shows part of the Shaft Scene that depicts a rhinoceros on the left, followed by a man with a bird head. There is a bird above, which could be a duck or a goose. To the right, there is a bison or aurochs, and on an opposite wall (unpictured), there is a horse. Sweatman and Coombs contend that these are not just pictures of animals but are in fact constellations. The rhinoceros is thought to represent what is today known as the Taurus constellation, the bison/aurochs is thought to represent what is now Capricorn, the duck/goose is thought to represent Libra, and the horse is thought to represent what is now known as Leo.
Figure 1.1 The Shaft Scene from the cave walls at Lascaux depicting a rhinoceros on the left, a bird that could be a duck or a goose in the middle, a bison on the right, and a horse on another wall (unpictured). Coombs and Sweatman contend that these could represent constellations and are intended to reference certain dates. (Alistair Coombs. Used with permission)
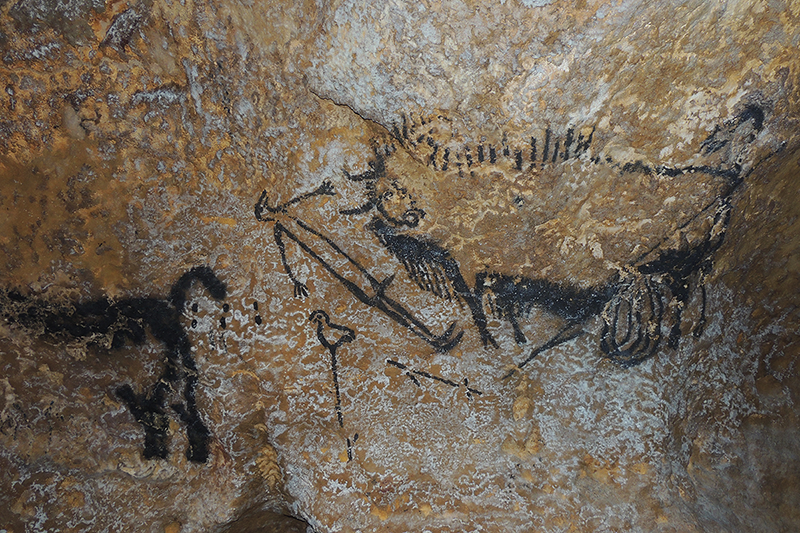
Fast-forwarding a bit, we can also see the visualization of quantitative information in the quipu, or talking knots, like those shown in figure 1.2 which were used by the Inca people in what is now Peru, starting around 2600 BCE. Talking knots were used to keep records about everything, from census data to taxes.
Figure 1.2 Quipu knots of the Inca people were used to record numerical information (everything from census data to taxes). (Pi3.124, CC BY-SA 4.0 https://creativecommons.org/licenses/by-sa/4.0, via Wikimedia Commons)
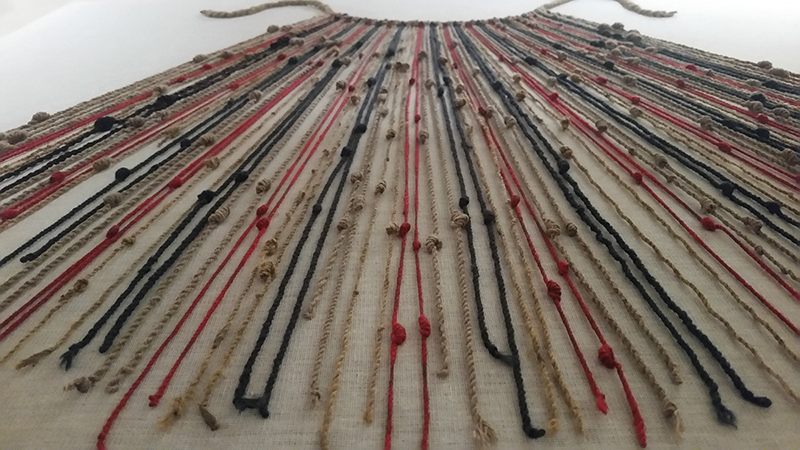
The earliest documented visualizations undoubtedly are maps, such as the Turin Papyrus Map dating back to the 1150s BCE in Egypt (see figure 1.3). The nearly 9-foot-long map, drawn by the artist Amennakhte, accurately depicts the location of gold and stone needed to build statues of King Ramesses the 4th.
Figure 1.3 The Turin Papyrus Map drawn by Amennakhte dates from somewhere between 1156 and 1150 BCE, and it depicts roads through the eastern part of the Egyptian desert, in Hammamat. (Public domain, via Wikimedia Commons)
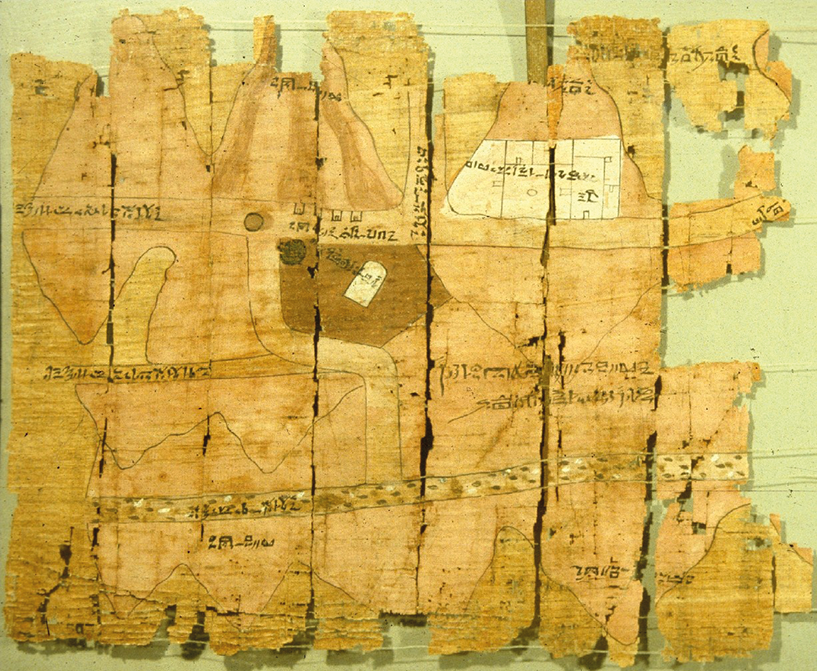
For the next couple thousand years, the most notable advances in data visualization were made in the world of cartography. However, around the 10th or 11th century, we start seeing evidence again of people recording astronomical information about planetary movement across the sky. By the 1500s, cartographers, navigators, and astronomers (among others) throughout the Eastern and Western worlds were regularly using instruments and tools to precisely measure geographical and astronomical locations, as well as other physical quantities.
By the 1600s, we start getting into the realm of “iconic infographics,” according to RJ Andrews’ “Interactive Timeline of the Most Iconic Infographics,” which can be found at http://history.infowetrust.com. It is a wonderfully delightful viz, and I highly encourage you to take a look on your own.
One of my personal favorites from RJ’s timeline is Napoleon’s march during his Russian campaign of 1812, shown in figure 1.4. This map expertly depicts six dimensions of data using only a single page: it shows the number of troops, distance they traveled, temperatures they endured, latitude and longitude of their travels, direction of their travel, and time. As you grow in your data viz skills, you will start to increasingly appreciate this impressive feat of visualization!
Figure 1.4 Charles Minard’s most famous work, a map of Napoleon’s Russian campaign of 1812, depicting six dimensions of data using only a single page (Martin Grandjean, CC BY-SA 3.0, via Wikimedia Commons)
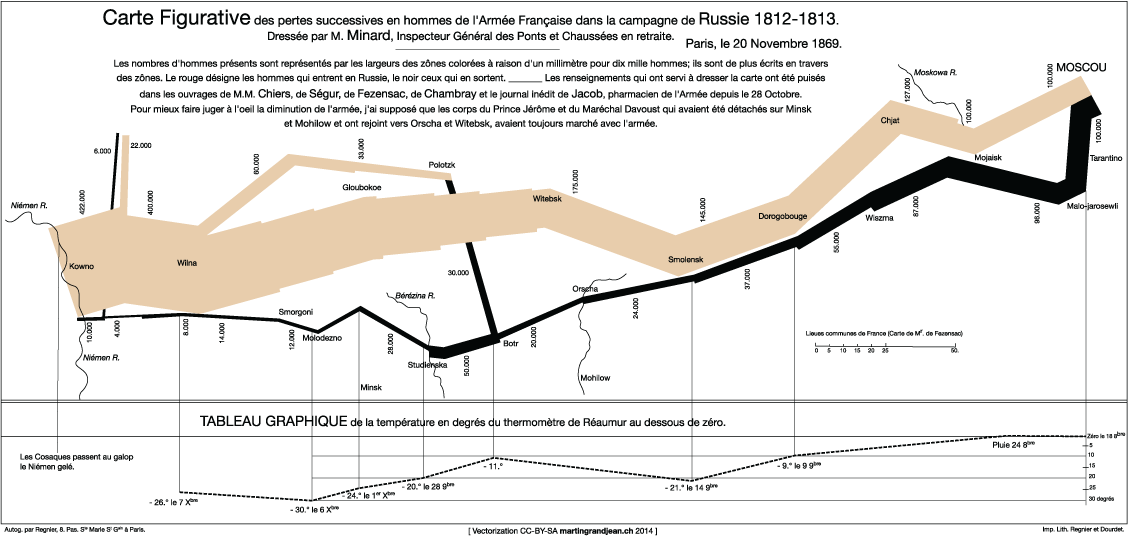
Perhaps you have heard of the woman, the legend that is Florence Nightingale? As the founding mother of modern nursing, this gentry-born Englishwoman collected and recorded data from her own experiences as a combat nurse during the Crimean War, from 1854 to 1856. Using this data about the conditions in the Barrack Hospital at Scutari, she created visualizations upon visualizations to lobby for better conditions in military hospitals. Pictured in figure 1.5 is one of her most famous pairs of comparative polar-area diagrams, a new chart type that she invented and is known today as a “Nightingale rose.” In these roses, she demonstrated that the leading cause of death in soldiers was not battle wounds (shown in red) but preventable diseases (shown in blue). Radial visualizations such as these can be a bit controversial these days because, as we’ll learn later, the human eye doesn’t interpret angles well. However, love them or leave them, these drove some big change.
Figure 1.5 Florence Nightingale’s most famous pair of roses, depicting the causes of death in soldiers during the Crimean War: preventable diseases in blue and battle wounds in red (Florence Nightingale, Public domain, via Wikimedia Commons)
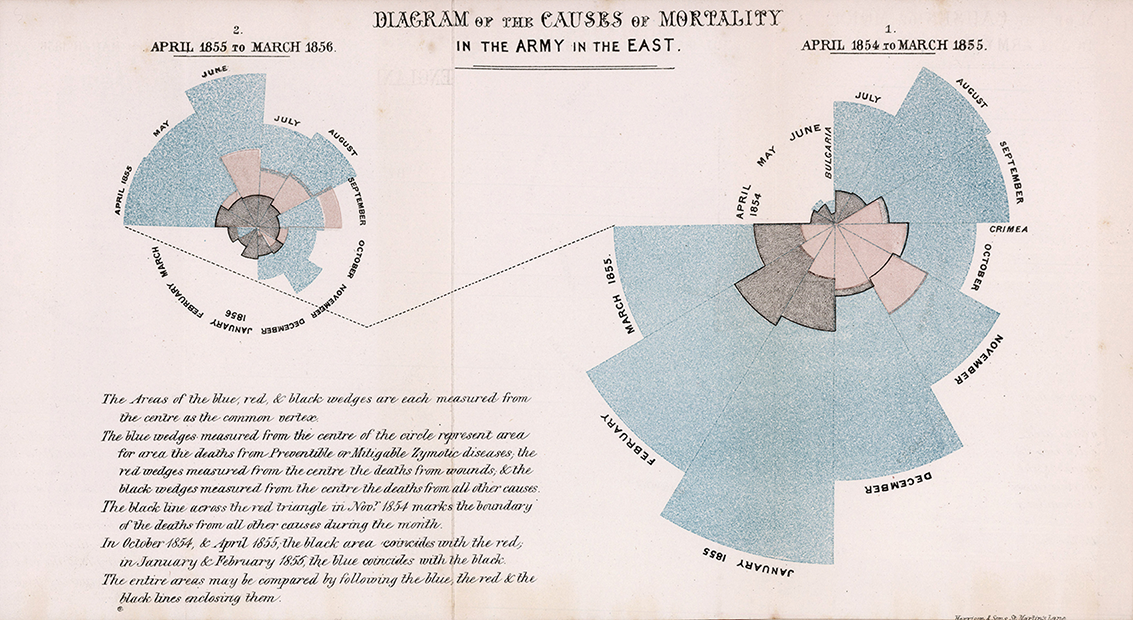
Armed with her data and visualizations, Nightingale was an early champion of handwashing and sanitation in hospitals, long before germ theory emerged. She lobbied all the powers that be of her time, sending self-published works to doctors, war offices, members of the House of Commons and the House of Lords, and even Queen Victoria herself. She drove massive change, the effects of which we still feel today.
Time marches onward, and so do we, into the 20th and 21st centuries. As one whose living is mainly made by the construction of interactive web-based data visualizations, it really blows my mind that up until the 1980s, you were going to be busting out (at best) a pen and paper if you wanted to make a data visualization. Spreadsheets didn’t come on the scene until VisiCalc was introduced to the public, first in 1979 on the Apple II, and then in 1981 on the IBM PC (yes, the great divide really goes back that far). Even today, this is the very favorite way for anyone to turn data into insights: you still can’t separate a finance person from a good old spreadsheet unless you pry it from their cold, dead fingers.
While we’re on the topic of spreadsheets, let’s talk a bit about tools for making data visualizations.
As we mentioned in section 1.1, the medium, or tools, used for a data visualization can be just about anything that results in something visual. While modeling clay and interpretive dance are totally valid media for data visualizations, in this book, we’re going to focus more on the two-dimensional and usually computational media.
Each year, the Data Visualization Society (DVS) conducts its State of the Industry Survey, and one of the questions they always ask is about the tools people use to create visualizations. Figure 1.6 shows the percentage of respondents each year stating they use a particular tool, where each tool is represented by a circle, and the percentage of respondents is represented by both the left-right position and the circle’s size. It’s important to note here that a tool’s change in rank from one year to the next may not only be due to its popularity rising or falling but also due to the types of respondents taking the survey that year or the tool’s presence in the multi-select list of options.
Figure 1.6 The most popular technologies by year (percentage of respondents) according to the annual State of the Industry Survey by the Data Visualization Society (DVS). Note that the emergence and increase in popularity of some tools may be due to the tool being added to the multi-select list of options for survey takers rather than a true increase in use. (Data Visualization Society. Used with permission)
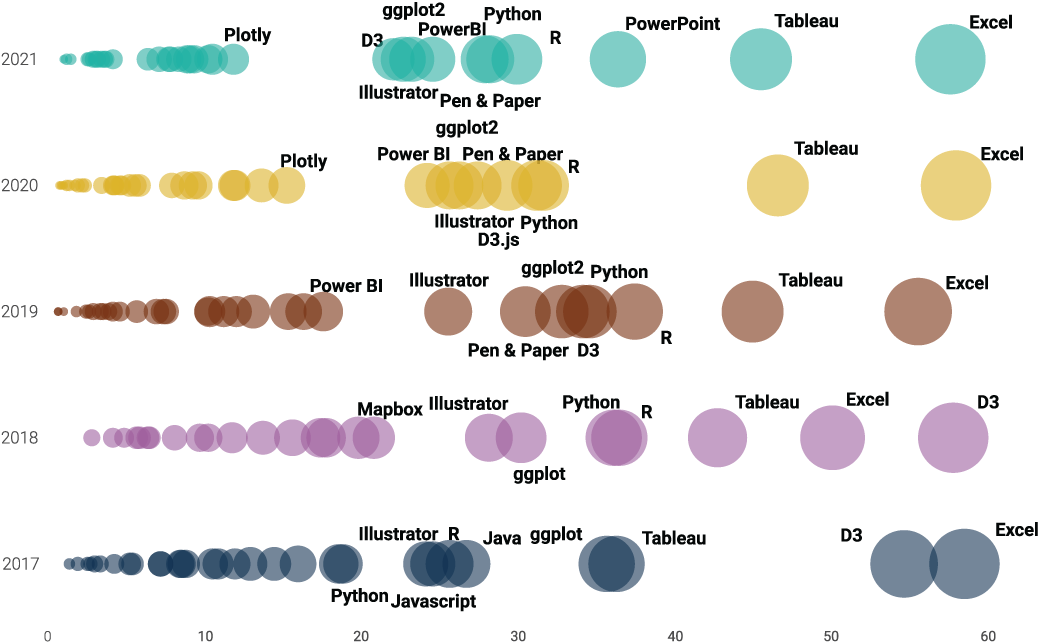
It’s okay if you are not familiar with the tools labeled in figure 1.6. We’ll be going over a handful of them in this section, but just so you can at least get a decent idea of what the list entails, I’ve broken down the labeled tools by type in table 1.1 for you.
Table 1.1 A breakdown of the types of tools labeled in figure 1.6 (view table figure)
D3 (aka D3.js or d3 or d3.js), Python, R, ggplot, ggplot2, Plotly, Java |
|
As shown in figure 1.6, Microsoft’s Excel is the most popular tool for visualizing data (except for 2018, but it only dropped one place to #2 that year). Excel’s popularity is completely understandable: as a program to which nearly all data viz practitioners have some level of access, its barrier for entry is remarkably low. It takes nearly nothing to paste or type in some data, and within two clicks, you can make a chart. The resulting chart, shown in figure 1.7, might not be beautiful, but it’s a chart, nonetheless.
Do you want to get more involved in your chart-making endeavors? Spreadsheet programs, even those not made by Microsoft, have you covered. You can create complex calculations to include in your chart, you can change titles and axes and formatting and legends, you can use tall data, you can use wide data, you can use big(-ish) data, and you can use small data. There’s a reason those finance people have such a tight grip: they’re MacGyver, and spreadsheets are the Swiss Army knife of data analysis.
I like to call business intelligence (BI) tools “spreadsheets on steroids” because, in many ways, they take what spreadsheets can do and greatly enhance it. As we saw in figure 1.6, Tableau was the most popular BI tool and in the top three tools overall for five years of the DVS survey. Power BI, which is Microsoft’s data viz tool and the biggest competitor for Tableau, is gaining in popularity due to its lower barrier for entry, oftentimes in the public sector. There are also competing products such as Looker (owned by Google), Domo, and Qlik, among others.
Figure 1.8 shows my sample fruit data in a chart I created in Tableau with a handful of clicks and a couple of drag-and-drops. Again, it won’t win me any awards, but it’s a bit nicer than the Excel one in figure 1.7, as I find the size of the bars relative to the white space between them to be less jarring to my eyes, and the gridlines are much lighter and thus pushed to the background.
BI tools might be your weapon of choice if you are making an internal-facing visualization for an organization. Many BI tools have an enterprise version that allows for some kind of publishing and sharing capabilities, as well as automatic updates of the data sources. This is great for regular reporting because it saves analysts and viz practitioners tons of time that they can use for better things than refreshing queries and emailing charts around. Oftentimes, there are options for stakeholders to subscribe to a report so that they automatically get updates and computer-generated screenshots sent to their inboxes or other communications platforms on a schedule. As a bonus, BI tools can often handle much more data than even the biggest spreadsheets.
Finally, depending on your technical prowess, many BI tools can handle various levels of coding, although you should keep in mind that the more you code, the more customized you can make your end product. The no-code GUIs usually don’t have a lot of flexibility in their formatting, while products that allow more customization will often require more coding and complex calculations.
If you’re already a software developer or engineer, spreadsheets and BI tools probably aren’t your cup of tea. In that case, you know well that writing code gives you the utmost control over exactly how your visualizations will look and behave. The good news is that even within the umbrella of coding, there are gradients of difficulty and control: you can use existing libraries tailored to the functionality you seek, or you can write your own functions, classes, and objects completely from scratch. When I’m coding, and I’m certainly not alone in this, I will often try to go the library route first—after all, why reinvent the wheel? Then, if I can’t find what I need, that’s when I’ll resort to writing something myself, and while my code sometimes turns out kind of like some of those “Pinterest fails” you’ve seen online, the resulting visualizations are exactly what I was trying to accomplish.
This section will go over two types of visualization development using code: front-end web libraries and statistical packages. I apologize to those with very little experience in the coding world because this section is about to get more technical than pretty much any of the rest of this book will. Teaching the basics of coding is well outside my purview, and you wouldn’t want to learn it from me anyway. Rest assured, we will not be doing any coding in this book, so if this is way outside your comfort zone, then you are welcome to skip to section 1.5.4.
Front-end web development is pretty much synonymous with JavaScript these days. If you are okay with not fully understanding when the different pieces of your code are going to run, then you should be quite successful with JavaScript’s asynchronous nature.
One of the most popular and powerful data visualization libraries for JavaScript is the free and open-source d3.js, also known as D3 or d3, which stands for “Data-Driven Documents.” Created by Michael Bostock, Vadim Ogievetsky, and Jeffrey Heer of Stanford University’s Stanford Visualization Group, it was released in 2011. As of this writing, Bostock is still by far the most active contributor to the d3 repository on GitHub, at https://github.com/d3/d3. It uses Scalable Vector Graphics (SVG), HTML5, and CSS standards and can be seen in action all over the web, such as in visualizations by The New York Times.
Figure 1.9 shows my fruit data again, but this time in as simple a bar chart as I could manage to make in d3. Creating the chart itself only took about 50 lines of code, which sounds like a lot, but d3 can be a bit wordy.
Earlier versions of d3 were rather monolithic in nature, where you had to import the entire library even if you only wanted to use a few pieces of it. When I first started using it, I would create entire web pages just using the d3 syntax (except for the barebones needed to create a blank HTML object). It was my gateway into JavaScript, and any front-end developer will tell you that this is an incredibly weird way to learn the language.
Nowadays, developers can use d3 in a piecemeal fashion, choosing only the components they need or want to use to create their visualizations. This makes it much easier to work within web frameworks such as React or Angular because, otherwise, there is a power struggle for control of the page once the code is running in a browser.
Personally, I love using d3, which is why I chose it to make many of the graphics for this book. It has a rather steep learning curve, but when you finally internalize its patterns and ways of doing things, as a developer, you feel like the entire world is at your fingertips, and there’s nothing you can’t do or can’t make.
If JavaScript and its asynchrony aren’t your jam, perhaps you’d prefer Python or R. While Python is an entire programming language, and R is a software environment specifically made for statistical analysis, both are free and open source, have a cult-like following among coders, and are great for data viz practitioners. I’m in Camp Python myself, as that’s what I started using in my undergraduate physics courses a hundred years ago, even though I’ve dabbled a little bit with R in the interim. Both R and Python have very friendly and approachable syntax, hence their considerable popularity. If you’re a statistician or data scientist, you probably already know R, and if you’re not, I’d wager you’d prefer Python. As for libraries to use in each, R users adore their ggplot/ggplot2 (which you may have noticed in figure 1.6 about data viz tools) and Shiny, while Python users have many other options, including Seaborn, Plotly, and Matplotlib.
Figure 1.10 shows my fruit data but this time brought to you by Python’s Matplotlib library and only about 18 lines of code, including 9 lines of my fruit data. I should note here, however, that these bars are not horizontal because the example I found for that was tremendously more complicated. Such complexity would have defeated the purpose of this exercise, so I opted against it.
One thing I really enjoy about Python is the massive homage to the British comedy troupe Monty Python. While I am generally more of a Mel Brooks fan, I do appreciate all the little Easter eggs found throughout the Python language and documentation. One such example is the built-in IDE (Integrated Development Environment) called IDLE, which they say stands for Integrated Development and Learning Environment, but coincidentally, Eric Idle was one of the troupe members. Wink, wink! I think I might be one of the only people left on Earth who still knows about IDLE due to the hugely popular Jupyter and IPython Notebooks.
If you made it to the end of this section, give yourself a nice pat on the back because you made it through what is easily the most technical part of this book. Well done, you! And just to reiterate, this book will not be forcing any coding on you, so if you want to use code to create visualizations, then more power to you. If you’d rather use something else, there are plenty of other options.
Moving on from coding, we come to the final and least computational way of creating visualizations on a computer: design software. Before we get into the details of which software does what, this is as good a place as any to introduce the idea of raster versus vector images.
Under the hood, raster images are just a bunch of colored pixels, tiny squares that contain only one color. When you zoom way out, you see them as a coherent picture, much like the pointillist painting in figure 1.11. If a raster image’s resolution is low, that means it contains few pixels in 1 square inch, while if the resolution is high, it contains many more pixels in 1 square inch. Some typical file formats for raster images include JPEG, PNG, GIF, and BMP.
Figure 1.11 A Sunday on La Grande Jatte, Georges Seurat, 1884. The inset shows how the individual points of paint are similar to the pixels of a raster image. (Georges Seurat, Public domain, via Wikimedia Commons)
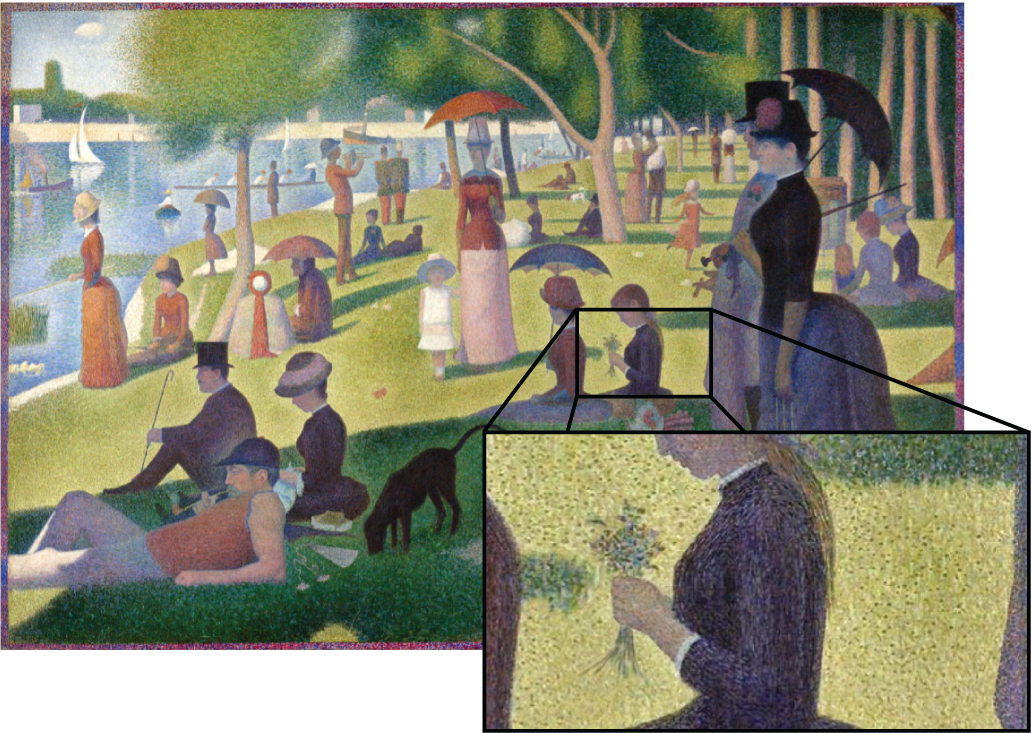
In contrast, vector images are basically a set of instructions for where an imaginary pen should touch the page and the locations to where it should move until the pen is instructed where it should lift off the page. It’s a set of paths that may or may not be filled with color, as well as instructions about the breadth and color of the stroke the pen makes, if any at all. Some typical file formats for vector images include SVG, EPS, and PDF.
The greatest thing about vector graphics is that resolution means very little to them—they can be scaled up or down ad nauseam and still maintain their fidelity because it’s all about the pen’s relative location on the page as it is drawing. As you might imagine, a filled-in shape with no stroke will scale differently than a pen stroke because the width of the pen stroke is still specified in a constant number of pixels, as demonstrated in figure 1.12.
The takeaway here is not that one image type is better than the other but simply that they are different. I said all that to point out that the different design software applications out there typically specialize in manipulating either raster or vector images, not both. Adobe Photoshop is the go-to solution for creating and editing raster images, while Adobe Illustrator is a classic solution for creating and editing vector images. At an enterprise level, while many design departments will have Adobe licenses, Adobe hasn’t done an amazing job at making it easy for designers to collaborate with each other or with stakeholders. Figma is thus my favorite solution for creating and editing vector images, including exporting them as raster images when necessary.
Figma can be used in a web browser on any device, or a desktop app is available to paid license holders. It has a freemium payment model, meaning that without paying, you can use nearly all the full features of the software in a browser, but you are limited in how many design files you can create and how you collaborate with others.
I adore using Figma for its intuitive interface and its cross-platform availability, but when I do collaborate on a file, that’s when it really shines. You and any number of users can be in the same file simultaneously, making edits and seeing each other’s changes (and even cursors) in real time! There is also a robust commenting feature where other collaborators can be tagged and notified, and then, they are taken directly to the place on the (infinite) canvas where the comment was left.
Not only does Figma excel at vector images and collaboration, but it’s also a great prototyping tool, so it’s perfect for the entire design process, from wireframing all the way through to high-fidelity, interactive prototypes. When you make something in Figma, you can click on any element and see the appropriate CSS (Cascading Style Sheets, which is the main way that the web is styled, including colors, animation, text alignment, and fonts, among many other things) tags to style final web elements exactly as shown in the mockup. It’s a dream for front-end web development.
Finally, many of the professional data viz practitioners, like those who make amazingly bespoke one-time visualizations for major news outlets, choose to create their visualizations in d3 for its handling of data. Then, they import the resulting SVG into Adobe Illustrator or Figma where they can do any fine-tuning that was too difficult or they chose not to do using code.
Figure 1.13 is the result of taking the d3 bar chart from figure 1.9 and doing some Very Bad Things to it in a matter of minutes in Figma:
- Made the fruit names bold and colored them purple
- Right-justified the fruit names
- Added drop shadow and rounded corners to the bars
- Filled the bars with a tri-color angular gradient (don’t try this at home, kids!)
Could I have accomplished all that craziness in d3? For sure, but I wanted to prove that you can do amazing things very quickly and easily in Figma.
- Data visualization, which is how we communicate information in a visual manner, is everywhere!
- Knowing your audience is the most important thing to remember throughout the entire process of making a visualization.
- Data viz has nearly always been around, from charting the locations of stars on cave walls and hand-drawing graphs that drive massive change to using computers to put the power to create and understand data visualization in the hands of everyone who wants it.
- When it comes to tools, there is generally a pretty direct tradeoff between ease of use and degree of control over the final product:
- A powerful and easy way to get even more control over a viz output in the form of a vector image, or SVG, is to import the SVG into a program such as Adobe Illustrator or Figma and make design changes there. However, this only works for one-time visualizations and not something recreated each time a web page is loaded.
- With so many tool options out there for digitally creating data visualizations, there should certainly be at least one that would suit you well. Remember, though, just because a tool is wildly popular doesn’t mean it’s the right one for the job or the right one for you.