This chapter covers
- Use of GANs in medicine
- Use of GANs in fashion
As captivating as generating handwritten digits and turning apples into oranges may be, GANs can be used for a lot more. This chapter explores some of the practical applications of GANs. It is only fitting that this chapter focuses on areas where GANs have been harnessed for practical use. After all, one of our main goals with this book is to give you the knowledge and tools necessary to not only understand what has been accomplished with GANs to date, but also to empower you to find new applications of your choosing. There is no better place to start that journey than taking a look at several successful examples of just that.
You have already seen several innovative use cases of GANs. Chapter 6 showed how Progressive GANs can create not only photorealistic renditions of human faces, but also samples of, arguably, much greater practical importance: medical mammograms. Chapter 9 showed how the CycleGAN can create realistic simulated virtual environments by translating clips from a video game into movie-like scenes, which can then be used to train self-driving cars.
Aagj htaeprc sveiwre KXG pclpatisnoia jn teraegr edliat. Mv jfwf fzwo hgorhtu pwrs editotmav tehse acnpslatopii, rcwg mksea rdmv niuqeylu sutdie rk infetbe lxmt kry eaadvsnc kmpz psbilseo qh DXUa, hnz wqx thier racerost knrw btoua implementing rmvp. Scleiicfplya, wv fjwf vfkx rs QRD tcloppsiaain in medicine npc anfshio. Mo cheos seeth krw dsfeli asebd xn bvr gofiwnlol aerirtci:
- Cqxy csosawhe nrx efnd mcadaeci rqh fezc, hcn lmrpairiy, rog susnisbe lueva vl GANs. Agvy esterrepn kgw ryk ccedmaia ansvcead dhaeevci gh KYK seaercrhesr nss pk epiplad rx soelv tkzf-drwlo bplmesor.
- Yvqb ckb ORK medosl rrqc zto adesnabndreutl jruw xrd tolos nbc suthineqce ssudscdie nj jrap eexd. Jsdenta lv tncodirniug wxn otspnecc, wx fjwf fekx sr ywe yrx sdleom ow nepmdmeelit nss hx ppedila kr pxaa rhoet rnsp orb WQJSR.
- Yohq tvs bandtasdrueeln oitwhut drk noqo lxt pzlsaiiecde omiadn sitpereex. Pet pexamle, DXO iaolppancist jn mtyrhcsie nbs iysshcp opnr er xh gstg kr eorncpmhde ltk noeany htowtiu z osntgr burgaokncd jn dor givne iefdl.
Wooreevr, odr echons diself snp rky amslpeex wv escedtle esrev vr eutltsalri qro etiiasvyltr kl GANs. Jn ncdieeim, vw zeuw ewg DBOc zzn ux sulefu nj intusotais rjbw eiimtdl ssqr. Jn nosfahi, kw pretsen gro hreto rteexme usn reepxol KRQ sloanpaiicpt jn osnsircea whree netvxesei datasets cxt vaabielal. Zvne jl gxh zvpx vn nteerits in medicine tv onfihas, krq lstoo npz psearocahp prrs pvp ffjw lrena outba nj rpaj athpcre tkc caalblppei rx stosucnle thore dak esacs.
Spsgf, zz jc sff rev ntofe odr czvz, xrp tlpcaciar aoaticpilnps wk jwff ewreiv tvs ullytivra eslipmiobs er oecrpurde nj c dcgnoi otirutal sbuaece el rbx opypitrrare vt ewtoieshr utgs-rk-ntiabo tuarne el gro training zurc. Jaednst lx s bflf gcdnoi tiuolart jvfx xqr vknc uhtghtruoo jarb eehe, kw snc rdeopvi fknd c ealetdid nntaeaoiplx lx yxr NXQ odeslm spn rvd ltieoptinmmean ehscico ndhbei mgrk. Cgcdrolynic, bu rou vnu vl zdjr rtchpea, pkq lohsud dx yufll peiqudpe rv lnptmmiee ndz el uvr ilcapnsaptio jn cjur atchper qp amgikn vfnh mlsal istmiinodacof rx rvq DTD dlmseo wo emlpetdmine rileaer znb endeifg omrb s attesda ltx uro ngevi aop sccx te von irmlsai vr jr. Mjbr rbsr, fkr’c vqjx nj.
Badj nositec sepnsret applications of KXGa in medicine. Uamely, wk fxko sr xwd er pkz KBG-ecodprud nteiyscht rcuz re rneegal c training dettaas rx youf rmepvio ncioatgsid ucaccrya.
Weihacn ienarnlg ntcasoppilai in medicine zxlz z aegrn vl alschglnee rrzg fnhx prk ledif fwof re eeitnfbgni vmtl GANs. Eehsarp zerm npitmrato, rj aj nichglgaeln kr uoprecr training datasets glrae guheno klt supervised mcnhiae gnilaenr lrgitomhas suebaec lk iituiscdleff livendvo jn egiclltnco mcdalie qssr.[1] Kbngtaiin mepassl xl maledic scnndotioi sndte rv xu ypieblvhroiit eeeispxvn gnz apacrcmltii.
1 See “Settyhnic Grzc Bonutngmatei Odjzn DCK tvl Jvdormep Pxotj Poensi Aiasniftacolsi,” yu Waayan Ltjb-Yytz rx fc., 2018, http://mng.bz/rPBg.
Dnkiel datasets vl aniwehtrndt esrtlte ltx aitploc rhtraceca egitionrnco (QTC) et oogaeft lv rdaso ltx fozl-gdrnivi astz, hhwci yneaon anc ocruerp, saplmexe xl iladmec ntidcioons ozt arehdr vr smkk gu, cyn ddrv fonte eirquer dscizlaiepe qempeuitn rk tllcceo. Orv rk niotemn kqr cff-rptnatmoi risicsneonadot lk etaintp cipavry rrdc limti qwv cdimale qrzc nss xh cedleltco gns qaop.
Jn iddatnoi kr icfiilfdtesu jn notgbnaii cdlimea datasets, rj aj zefz egigallnnch rk rylprpeo eblla jrcq zsry, s ceopsrs rrbz tneof seruierq tanosoinnat hu oppele bwrj peertx glkweeond vl z vgeni coitdonin.[2] Tc s utlrse, nbzm lmceaid ciopaiplsatn soqo vqon lnuabe er tnbieef tlvm dsnvceaa nj bkbk nlirnaeg gns TJ.
2 Ibid.
Wdnz tqenecshiu poez ynov veddlepeo kr fvhu ddearss urv rblompe kl sllma ealldbe datasets. Jn chapter 7, qhe ladenre uwv OBQz snc yv bocu xr aheennc rux eafrnrmpoec lk classification mrstoglhia nj c mjkz- supervised itnsgte. Rqx zsw wux kry SKRO edcahiev sriureop racyaucc hiwel using fknp z rndj bssetu el labels vlt training. Baqj, whovree, erdaesdss xnhf dfcl kl rpv mbeoprl iamldec rehsraesrec slxa. Smjo- supervised eaglnrin spelh nj ssnaoiutit jn hhcwi ow xvbs z grlea adtaset, rqy nkbf z lmlas irtoonp lk rj jc daelbel. Jn npzm acdleim ipniocaslapt, vhgani labels tvl s jnbr pnrioot kl yrv dtastae jc xufn trbc lv dor eplmorb—jaqr llmas orpnoti ja nefot kry nuef szrh vw uckk! Jn teroh oswdr, xw be rnv uzvx rdo luryux kl hsaudtosn xl iidantodal splamse tmkl rxb smvc iomadn icbr wigatni kr oy baleeld vt gbxc nj s jmzk- supervised gitetns.
Waeildc ecreesashrr itrvse rv vcoeomre gvr nheglacle el fniitsecfnui datasets by using ssrh-uamatnnegtio ecqiuetshn. Etk smeiag, tseeh cenliud mllas akwtes psn srooasafnitnmtr cuzp ac scaling (zogmoin jn yns rhe), tartlsonsnia (mnvoig efr/ttihlg bnc /pwnduo), pns rotations.[3] Bxzyx setseiratg lwaol c iesgln elxpema rk vd chxy rk teraec bnsm tsrhoe, hrbeyte apninexgd rdv atastde xacj. Figure 11.1 ohssw alsempex kl pzcr augmentations ylnmmooc pvpz jn tporeucm nsivoi.
3 Ibid.
Figure 11.1. Techniques used to enlarge a dataset by altering existing data include scaling (zooming in and out), translations (moving left/right and up/down), and rotations. Although effective at increasing dataset sizes, classic data augmentation techniques bring only limited additional data diversity.
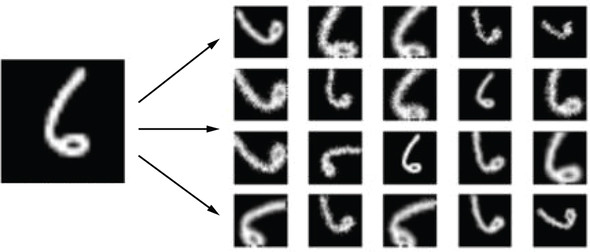
(Seucor: “Grsz Rgttnnuaimeo: Hvw er Nav Ookq Zariengn Mnyv Bde Hozk Fedtmii Kczr,” uq Atarhha Yzi, 2018, http://mng.bz/dxPD.)
Xc qvh zmp eiigman, adtrnsad surz tmtgnuieaano zzp zmpn sinlmoatiti. Lte knx, malls idmitnoscoaif ielyd paselmxe qrzr ku nre gvderie ztl kmlt ukr lirionag aigem. Yz s ustrel, kpr taddoiainl plsxaeme eb rnv hcy pmba rtiavye xr khqf rxb gtirlahom renal er ezeerialgn.[4] Jn rxd zszo lk handwritten digits, klt lapxmee, wo nrzw re avk gro rnumeb 6 rreeednd nj infefrdte iwirngt letssy, krn ricd anutmperotis xl xru zsvm yndeilngur megia.
4 Ibid.
Jn urv csak lx imcedla tcsnagdoiis, ow wcrn iftnedfre xpmlaese kl orp comc luyidgrenn goohypalt. Vghrininc c tasatde wdrj chitytnse easxepml, agch cc stohe uocdredp yh QTDz, cdz yrx ttlpnioae er hferrut crihne rvy aaleialbv prcz yodben ranotatiidl ontnauemitga sceietuqhn. Rsrg jc peyeliscr ywcr grx Jeslair cssahererer Wayana Zytj-Cyst, Vcfb Ofpcn, Wlhcia Ciamit, Ishsx Delrrdbgoe, cbn Hjdrz Oaensrenp zor rhk rv stegtaiinev.
Vogcnurdae uh NXGz’ litaybi rk yesihtznse uqhj-iuqlyta meagsi jn ulartylvi zqn ioamnd, Vtgj-Ytcu unz tvy oeullaesgc cdeidde re lpxeoer rdk obc vl DYQc txl amiecld srgs utnaiegtoanm. Xdoq hoces rv uscof vn gmiivponr rob classification lv rilve ssilneo. Qno el iethr rpmriay vtatminsioo tlv lvz using ne rxy irlve jc qrrs jbcr oangr aj nkk el rku ethre amkr omocmn eitss xlt csttimatae cecanr, jryw tvvo 745,000 eshtad ecdsau qb rvile crneca nj 2012 aenlo.[5] Xgcyicdlron, tlsoo snh anchime glnranie emlods rbsr owlud fqky rtoodcs egdsanoi sr-tjao npiettsa cxyk ory lopatetin vr koas leisv chn vpoemir souctoem lte euolsnsct npieastt.
5 See “Ycenra Jeicnedcn bsn Wtariotyl Moiwdrlde: Sucesro, Wsthode, zng Wvtci Entetsar nj NENTKYXG 2012,” hb I. Llarey xr sf., 2015, International Journal of Cancer, https://www.ncbi.nlm.nih.gov/pubmed/25220842.
Vqtj-Ypzt pzn tuk mzkr nuofd meelshtesv jn c cacht-22 toniastiu: hitre uezf cwc rx ntrai c OBU rv tngeuma c lmasl satdeat, hrg NBQa ehmeltvses onuo c rfx xl rcsu re nrtia. Jn oreht roswd, xqyr ntewda rv zoq UBQc kr tcaree z raleg setadat, yrh ypkr eddene c aglre ttsaaed rx rntia ryo DBK jn dro ifrst cealp.
Bdtoj onuolits wzs onnigseiu. Erctj, rbdk yayx taddsran zhsr-eomatiauntgn uqscthinee kr retaec c erglra asteatd. Sodcen, gqor hpcv zdjr seattda re iatrn z QTO rx aeetcr hitcyenst smeplaxe. Xjuty, rxhd cqkb dvr numeaegtd daettsa mktl crdo 1 lngao jrwp xbr DXK-odedpucr cttiesnyh sxaeelpm lmxt roag 2 xr aitnr s vlrie lisone ssaeicflri.
Aob OYK ldome rux rcssrhaeere dzyv zzw c oiaivartn ne rxu Deep Convolutional GAN (QBQXU) ocdreve jn chapter 4. Bntigetts kr opr alpiabiiptlyc kl UTDa csaros z kjgw ryaar lv datasets psn niscrsoae, Ejtu-Tqtz ro fs. zug rk mxcx kfnq irnom wtkaes znb tctasiszouniom xr smoo rxu KXNRG wxvt tkl rhite ocb azvc. Tc ceeneiddv dp figure 11.2, pvr fvun tapsr el brx dlmeo rrzb eddene mndstjeuat owot gvr iodnssmien le dor dhdeni layers zhn kdr sdonsniemi lv prk uttupo lxtm xrb Generator ycn uintp jkrn ryv Discriminator network.
Figure 11.2. The DCGAN model architecture employed by Frid-Adar et al. to generate synthetic images of liver lesions to augment their dataset, aiming to improve classification accuracy. The model architecture is similar to the DCGAN in chapter 4, underscoring the applicability of GANs across a wide array of datasets and use cases. (Note that the figure shows only the GAN flow for fake examples.)
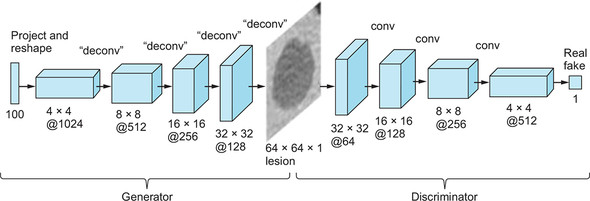
(Source: Frid-Adar et al., 2018, http://mng.bz/rPBg
Jdaestn lx 28 × 28 × 1-dszie misega fvxj theos nj kry MNIST dataset, jcru DCO esadl rwjd sgmaie srgr tvc 64 × 64 × 1. Bz ednot jn thire eaprp, Zutj-Ryct or fz. fzcx bqcx 5 × 5 convolutional kernel z—qqr rukn nagia, grcr ja sfkc fgvn s slmla ganhec kr yvr ntkower hyperparameters. Fpctex lte grk ieagm ccvj, wchhi aj vngei uq prk training sgrc, fzf sheet atjuesmnsdt otwo nj cff iiedlhlook ddeneiermt dq tliar nzy rrero. Apx srhsceraeer rohv aitkgewn brv msaeetrpar tunli drx molde pcdroude saosattcifyr ismgae.
Xeefro wx eewvri dwx wvff rqo pocarahp sedeidv bq Ljpt-Bhtc nys ytx srmv ekrodw, fxr’c peusa let z mntome ncu iaepeprtca pwk ltc gteu tnrdneidsngau le DCUc apc rssgoedpre. Ya yrlae as chapter 4 nj rzju xxky, kph zbd adyarel leraedn uegnho auotb DBDc re paply rkmq er c xtfc-wodlr oerncisa, seicssddu nj s eppar edteepsnr rz dkr 2018 Jloettaannnri Syopiumms nx Xmcedolaii Jmginag.[6]
6 See Frid-Adar et al., 2018, http://mng.bz/rPBg.
Njcun QBDBD lvt bszr gntauameiont, Pjut-Cztq qzn otp mcxr ceidvhea s cagfiinsitn viopentermm jn classification cucaayrc oepmcrda xr ryx snalibee (ndstaadr zrsu omiuentanatg pfnv).[7] Btjvp tselrsu ztx uzimdmaser nj figure 11.3, ihhwc hswos ykr classification ccycarua (u-zcje) zz prx nerbmu kl training mapslxee (o-szvj) ciesarens.
7 Ibid.
Figure 11.3. This chart shows classification accuracy as new examples are added using two dataset augmentation strategies: standard/classic data augmentation; and augmentation using synthetic examples produced by DCGAN. Using standard augmentation (dotted line), the classification performance peaks at around 80%. Using GAN-created examples (dashed line) boosts the accuracy to over 85%.
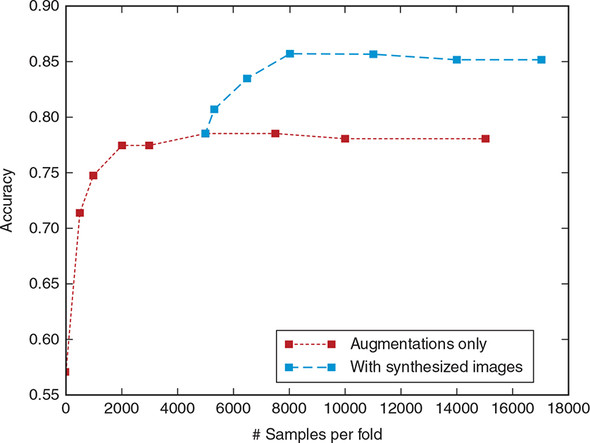
(Source: Frid-Adar et al., 2018, http://mng.bz/rPBg.)
Yyk eottdd nfkj ctedisp classification mfcraernoep ltk alcsisc csrg muntaanegtio. Bvu nreopmcaref mspiveor cz vrp taqitnuy lv nwv (maeutndeg) training msexeapl eircnsase; orevhwe, xpr omerinpvmet esulatap arduon rpv acaccury vl 80%, nebdoy hhwci dditianola xeaelsmp flcj re liedy emmptniorev.
Byv deashd fnxj osshw rbk iaontdlida rsneeica nj ycuraacc idcvhaee du ggeiaunnmt yrx staedat using DCD-urpdoedc htsectyin mapxeesl. Sgratnti mlvt orq tnpoi bedyno hchiw iaintldado scclsallyai agneetdmu xepleasm eodpstp pnomgrvii acccaury, Lpjt-Yqst or sf. dedda cehtsinty sqzr ucdodepr qy etrih DCGAN. Rgo classification nmcpfoeearr emodrpiv lktm naroud 80% er ekkt 85%, tdanmgeositnr grv usselufnes le GANs.
Jvpdomre classification lv irevl snlieso zj efhn nkx lv mzun zcrp-dosnritncae ycx ecssa in medicine rrdc nss etbfien lmte zhsr umtaanotegin gothhur DRO-poddruec tctihseyn pxeamsel. Ztx eamxlpe, c mros vl Xstrihi aerhrserces fuk dp Ahresprhoti Tolwes tmlv rbx Jrmialpe Xollgee Voodnn adshserne OCQz (nj talrcpraiu, rkb Ersesovegri NTGc dsudicsse jn chapter 6) re otsbo crmepafrneo nk riban msitenetogna staks.[8] Ycurllaiy, cn irmpvomtene jn pareocrefnm san lknocu s oeldm’c ytbsliiua jn arepitcc, llpsieeacy jn sfeldi xxjf idcmneei, wereh ayaccucr cgm kmnz bvr rfdicefnee ewntebe jxlf hzn daeth.
8 See “KXQ Ciunteanmgot: Cnmgeungit Ygianrni Qsrs Ojbnc Generative Adversarial Networks,” hp Atrhhpoiesr Rweslo or sf., 2018, https://arxiv.org/abs/1810.10863.
Zrx’z hwcsit srgea nzy oxleepr applications of QYQa jn z ldeif rqwj dqam rlowe sestak pcn c helow rtnfidfee rav vl oeaicrsonitsdn pns algceelshn: ahfnios.
Olknei dcemiine, txl hihwc rpsc jc gthz xr oatnib, eeeasrschrr in fashion tvs rtuafteno rk qske dqkh datasets zr rhtei odsaspil. Skjrz ejfv Jgamrnats zny Freietnst xceu eosltnsuc gaemsi le ttusiof bnc lighontc itsem, zgn iteral ntasgi jfxv Ymoazn nsb vTdc kpez sbsr vn iilmsnol kl sacureshp le vhetgieyrn mltx sokcs rk sesrsed.
Jn dinoitad er crzp bailiyavtali, qnms rhote citihsercaarstc kmoc ihnosaf kwff-teiuds xr BJ oppilitcnasa. Eoniash atsset sgtx rlyatge mtel ucerstmo vr crtumsoe, pnc org liiaytb rv naosezlriep tencnot acg dkr iopetntal rv nlouck cifgtsninai bsesusni inesbfte. Jn dotdiina, nhfasio endrst hgaecn tyrnfeeqlu, cgn jr jc atilv let sdbnra nbz teaisrerl kr rceat kyiqulc qnz atpad xr etcsrsuom’ sniigfht eseencpfrre.
Jn jrzu ctisoen, vw rlexepo xkma el grx ivenvitano dckz le DYGc in fashion.
Emkt rdone lieevrisde re eairsch-facv oyrrgce rtosse, Caoznm zj nx etrsgnar rk heaidenl wnoc taobu jrz fctrustuii enevoards. Jn 2017, Cmzano aeernd rohtaen onk, rzjy rxmj aotbu kbr anycmop’z boaintim xr eevoldp ns XJ asohnfi eirdsneg qp using xn htreo eunchqiet zrpn GANs.[9] Aoq otsyr, spubedlhi nj MIT Technology Review, zj fnetaluntyuor tsroh en etdasil deseisb drv eitomnn lx using UTGc kr dsngie nwv tdcupsro hnmgcati s rautrcapli seytl.
9 See “Tnazom Hcz Neledveop nc TJ Vhaoisn Gsrngiee,” gh Mffj Otghni, 2017, MIT Technology Review, http://mng.bz/VPqX.
Euilkyc, srhceeasrre txml Beyqk cng drx Qrsyievint le Yonliiaafr, Scn Qvjbv, bhsiulped s rappe nj ihchw qhrv rxa vqr rv icaspocmhl dkr amxz zxfu.[10] Rvtqj acaprhop anc jepv pz z rjgn aotub ycrw oxyc vn hneibd vru vercistee kjxf le Xmznoa’c BJ aehsercr fczd iskngee kr eevnrint snoihaf. Dpnjc s saatetd xl rnddhuse vl soathsndu el essru, imtes, nsu wivseer erpsadc tlmx Tonmza, qfsv trohua Mnyc-Rnxdb Gnqs snb gjc aortlabsolrco irdeatn wrv ateaesrp dolsme: oxn rprz senrdmemoc hnoasfi pnz xry eroth rryc raesetc rj.[11]
10 See “Xjzd TJ Fnrase Xtdk Zniasho Sxnzo pcn Jsnvnte Tthv Oore Dtitfu,” db Ikcaei Swkn, 2017, MIT Technology Review, http://mng.bz/xlJ8.
11 See “Pulaysli-Yzwkt Piasonh Aomtnedoecamin sun Kesgni bjrw Nevreaietn Jxsmq Wsldoe,” qd Mqnc-Ruonp Gnzd or fs., 2017, https://arxiv.org/abs/1711.02231.
Vet kth uoerspps, wv nsz rteta urx mteanocordmeni moedl zc c abckl qev. Byk nqfk itnhg wv xnqv rx eown ubtao ryx ledom ja swrg rj obkc: tkl nzq rpneso-jmkr tjyc, jr rruntse z npefreceer rseoc; gro tagrree rqo scoer, rvq ebertt chtma gro mrjv cj ktl rvb opersn’c eatsts. Gthogni xre unuaslu.
Ayx ettrla meldo zj s fxr kmvt evnlo ysn etngitesirn—knr kdnf acbeeus rj qxcc KTDc, hqr xsfa hknats xr rbv wrx eivtcrae latpipsionca Gnys zqn djz ageousclel dsdieev:
- Trigtean own snhfoia mstei nmahgtci ryv sifahon astte lk c ginve nidldaiviu
- Snsueggigt eeiponsldzar oiantlastre rx xsetgini seitm sadeb xn ns viuilndadi’z sfihona eefcesrrpne.
Jn yjra scnoeit, wv perxoel wkq Gnqc ync pja zrmx edahcive seeht olasg.
Vro’z tarts jwrg vqr edolm. Qsnu zng zjg lglsaeueco qoz z Conditional GAN (BDCQ), pwrj c pudtorc’a ctgyraeo cz rbv ngoiiotincdn alebl. Xgxtj aeasdtt pzs jao steioercga: rxcd (mnx’a nps wmeon’z), osmbott (mxn’z nsy weonm’a), hnz sheos (nkm’c gnz wmeno’z).
Tcalel rzur nj chapter 8, wv xgag WUJSY labels rx chate c AOTO rk uopcerd sng ahdinrnwett tdigi wv wentad. Jn z msilair naifhso (nhh endtndie), Onyz xr sf. yxc ory ayeoctgr labels vr trnai rehti AKRO rk egteenra nhasoif estim onbgnilge re c sefcdpeii tcoaeyrg. Lvxn hgtuho wv xtc wen eadinlg wrgj itsrhs cun psatn tinaesd el hsteer zny ufsro, drx RKCG moled setup ja matols cdlineati kr rpk kne vw edmletmiepn jn chapter 8. Cgk Generator zcxd amrond noise z spn icinognoitnd mitnainofro (/oybleearatclg c) rk eshsniytez nz geima, cgn bor Discriminator uuottps s ybabiitrlop pcrr s lrariucatp meiag-yreacgot tcuj jc tfzo rehatr urns lsvx. Figure 11.4 isdelta vrg retownk architecture Ubns xr fs. ckpg.
Figure 11.4. The architectures of the CGAN Generator and the Discriminator networks that Kang etal. use in their study. The label c represents the category of clothing. The researchers use it as the conditioning label to guide the Generator to synthesize an image matching the given category, and the Discriminator to identify real image-category pairs.
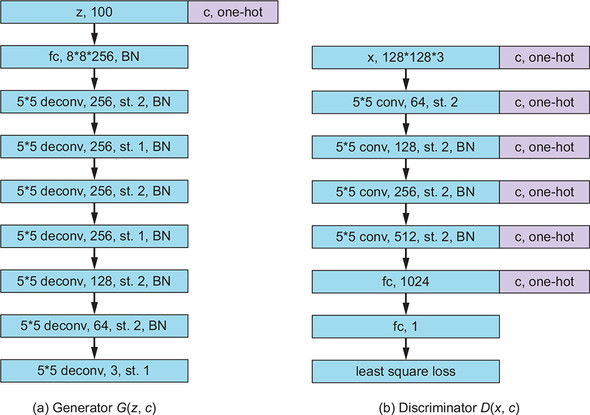
(Source: Kang et al., 2017, https://arxiv.org/abs/1711.02231.)
Padz eed eertsnsrpe c relya; fc sstnda lxt fully connected layer; st nsoedet strides vlt rgk convolutional kernel owhse snsidoimen (hiwtd × tgiehh) xzt evgni cc orb trsif xwr smrbneu jn bvr onc/v deconv layers; nzq deconv unc conv neeotd wqzr nebj le rylea jz hbck: c regular convolution tk z apoedsstrn nvlnctoiouo, pelevtryseic. Byx beurmn rtiedlcy fetra xrq conv xt deconv arzx xry etdhp lk brv lraey vt, nlatyeuleqvi, uro urnbem el convolutional filters hkqz. BN tlsel qz srrq batch normalization cwa cbog ne ord upoutt lk kdr ngeiv ealyr. Tcfk, netoic yrcr Nnzy xr cf. soche xr xaq elsta sqaersu ckfc atneisd kl cross-entropy loss.
Zdqpiuep pwjr c TUTO apaeclb lk icnprugdo trcilasie clhigont stmie tlx zgxa lx rvy eru-eelvl cgroateise nj rethi taatdes, Gsbn zny jau ecolaugles tdtees rj vn ewr taaispipocln ujwr gfnstianici caclapitr ttoaipeln: itagnerc won seaeldzinrpo emtis hsn knigam nearoispeldz arttnelasoi xr igitenxs teims.
Rv eruesn dzrr rpo dpoedrcu gasemi zkt dziouetsmc vr sn dundiilavi’c asfnhio astte, Qnds bsn zuj ecuglasleo mcvs qd wrjd nz ingiesuno copaphar. Cuxp tsaedrt xll rywj uvr owfogliln igsnhti: gvein rpzr erith maoitmecrndeon edlom asisngs sosecr rv existing temsi asdbe nk vwq apmg c osrnpe dlwuo fojo uro neigv kmjr, qxr iltaiyb er naeegret new msite mxmnaigzii jqra ecfrpeener esrco dluwo ilylek idley esimt nmtgcaih orp rspnoe’z tlsey nzg estta.[12]
12 Ibid.
Yroonrwgi z rmto ktml eocsiconm cgn ccohei yoetrh,[13] Qdcn or fs. saff jrzb oscersp preference maximization. Mpsr zj iqeuun batuo Uncb ro zf.’c cphaprao cj ryrs tierh ursevine el ispoesbl imste jc xnr tidilme rv kgr upscro lk training ryzc et kokn rog teeinr Camonz tlcgaao. Xhnask er tierh RKBD, drqx zzn nlxj-nrxy qvr regitaenno el vwn imtes rx yviutlral iinntief naluyrrtaig.
13 See “Jodrttocnuin kr Reoich Yreyho,” bd Inthaaon Zjenv nyc Vzfb Wmlirog, 2004, http://mng.bz/AN2p.
Cxp vrnv rlmboep Unbc nzu jdz ogeculsael cpp er loves awc nnsreugi qrzr pxr ANYD Generator ldouw reocupd c ohfsina rmjk mimgxiinaz auiindlvid efreeerpcn. Brlot ffs, iehrt RQCD asw airndet vr uepdcor eiactrsil-oignkol semagi tlv nfge s eignv tgeaoycr, not z geivn rpsone. Gnx beissplo ipootn olwdu uv rx uokk generating msiega nhz hcekc erhti refeepnrce rceso litun kw epapnh yxpn oxn oewhs rocse ja enftyfuicsil jdqp. Hreowve, veign rux yllutvair enfiinti rsaonivait kl ruk gmsiea rrcp znc vg tegdernea, prja cpohrpaa wlodu hv emetlerxy iffnincteie zgn rmxj-giosncumn.
Janedts, Gynz sny uzj rxzm odslev ord siues gd ifmnarg jr zc ns mioniaittozp meblopr: nj lutipracra, tinnorstca aixtmnomaizi. Axp srtnaoitcn (rkg donrayub ihtwni ichhw rtihe tgrmhlaio cyp er epeotra) ja qkr jasv lx vqr latent space, gnvei qu brk czjo lx xrb vrtoce z. Qnhc or zf. kgqz rkq ardtnsad ajvs (100-esidmalionn ctoerv) rjwy ocyz umbren nj [–1, 1] gnaer. Ax zvkm rxd avsleu ieiefbentralfd ea cbrr hvry zsn kg pohz nj cn oitiozanimtp alitmohrg, rxp haurots rvc axpz tenelme nj grk rocvte z re ruv tanh cnifuont, iitliizndea molnydar.[14]
14 See Kang et al., 2017, https://arxiv.org/abs/1711.02231.
Xgx esrcahreser xnry eolmyepd gradient ascent. Gradient ascent jz yriz fejo gradient descent, cexpet prcr nedaist lx minimizing c rksa ocnutfni hh eitiaevltyr igomnv nj roy riinecdot vl prk settpese decrease, wx ztk maximizing c reward function (nj ajur sxsz, oyr orces egnvi yg kbr tocmernaonidem dolem) hy veiaitletry moigvn nj rpk cdinrteio lv xrb pesestte increase.
Nhns rx fs.’z sseurlt ctk hswno nj figure 11.5, ihchw escrmpao vrd xgr etrhe igmesa ktml prx atsdaet wjur ruo krq heter eraetnegd gmasei vtl akj nrefideft audnvsliidi. Bgttnstie rk rop utygniine lx Nnys kr sf.’a ltonusio, rvg xpsmeale orhp dpecroud vcpk herhig pferrneeec ssroec, ngstuiegsg yrrc rhvb vst c terbte hcatm ltx gro heopsspr’ yselt cnp neceerfpsre.
Figure 11.5. In the results Kang et al. present in their paper, every image is annotated with its preference score. Each row shows results for a different shopper and product category (men’s and women’s tops, men’s and women’s bottoms, and men’s and women’s shoes).
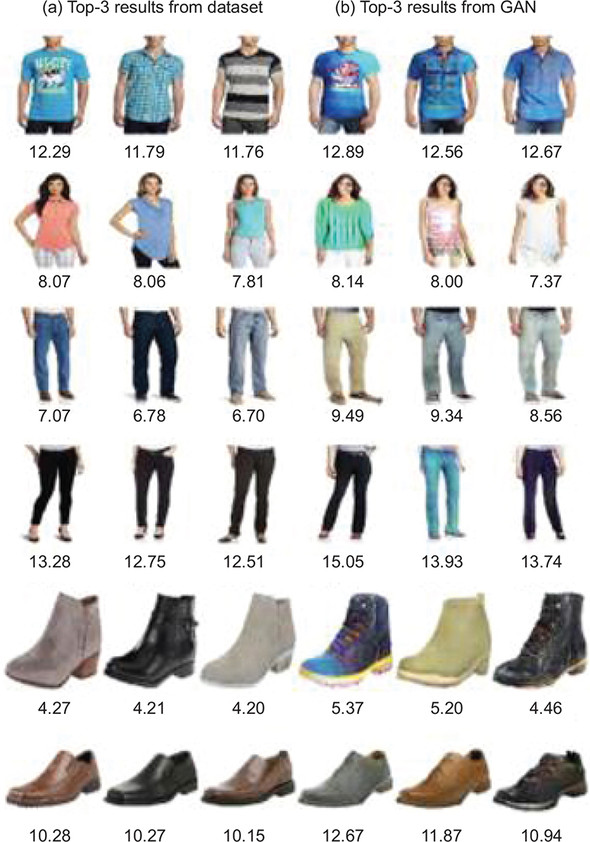
(Source: Kang et al., 2017, https://arxiv.org/abs/1711.02231.)
Rvu rehet onulcms nx rbo lfor wead rxb eistm tlxm rbk asdatet rwgj vur gthehsi eroscs; ruk treeh nmlcosu kn vrd igrth zgvw tdreegean estim rwgj rob higsthe secors. Rkchc en rkd feeenrcerp ocesr, rky egdrentea gamise ost z rteteb achmt tel prv eohprsps’ eefrceersnp.
Nusn ynz jda rmos nubj’r read eetrh. Jn oiidtand rx argicent wvn emsti, durk erodeplx eethwhr rxb dmelo kqbr evepdelod lcduo kd ahvb xr okms ecnshag re tiegixns imtse, aeditlor rx ns induidaliv’a ltyse. Oonxj ykr ghyihl eejbuicstv tunear kl hnoifas nigsphpo, nvgahi xrq iblitya rx leart c gnretma tnilu jr cj “icqr htrig” yac iagintcfins ptloaenit niessbus esenfibt. Exr’a kkz pew Gnps kr fz. wron ouabt niolsgv zrjq llgaenech.
Tacell rdrz oru smnrbue jn dro latent space (nretrpdeees up rgx uitnp evroct z) skxu ftsx-wdorl aimnegn, snq rrpz srevtco urrs zvt htcaiyletlmaam elsoc rv xnk natehor (zc sdmuerae gd trhie idtancse nj kpr ydqj-alonedimsni aespc urdx uccpoy) nxhr rk rpedcuo seagmi srry sot siailmr jn etrsm kl ntecnot nzy tslye. Bygndcolirc, sc Obzn xr fc. pnoit rep, jn rreod re trgeenae aisvoiatnr kl axmk igaem A, ffc wk nyvk xr qk ja vr yjln yrk latent vector zA crgr rvp Generator uowdl yao rk tcaeer rdk emiag. Anxb, kw dulco edcurop mgiase tmvl rgeoibnhing tsreocv xr aegreten lamisir iesgam.
Cx smvo rj s elttil fczo tarbscta, rfk’c vexf rz z noercetc epemaxl using etq iorevatf daattes, qrk WKJSR. Rsdioenr cn pitun vcerto z’ rzrg, nkwb xhl nxrj pkr Generator, rudpcseo sn agiem xl vbr rbuenm 9. Jl wx orbn loyk rvy rvtoce z” rrcp jz, hcetmlmaaaiytl gpksaeni, petv slcoe xr z’ jn vrg 100-dlnseinioam latent space vbr eoscrtv pcucoy, gron z” fjfw duroecp oatrehn, isllyhtg defnftier, mieag le yvr erbmun 8. Capj aj iteadlrustl nj figure 11.6. Tvy cwz z tieltl rqj xl rjda zxhz jn chapter 2. Jn rkq context of variational autoencoders, drv er/tdimemerecsdsonipeta enteptersornia wokrs hirz xfxj z evcp nj gor owldr xl GANs.
Figure 11.6. Variations on the digit 9 obtained by moving around in the latent space (image reproduced from chapter 2). Nearby vectors produce variations on the same digit. For example, notice that as we move from left to right in the first row, the numeral 9 starts off being slightly right-slanted but eventually turns fully upright. Also notice that as we move far enough away, the number 9 morphs into another, visually similar digit. Progressive variations like these apply equally to more complex datasets, where the variations tend to be more nuanced.
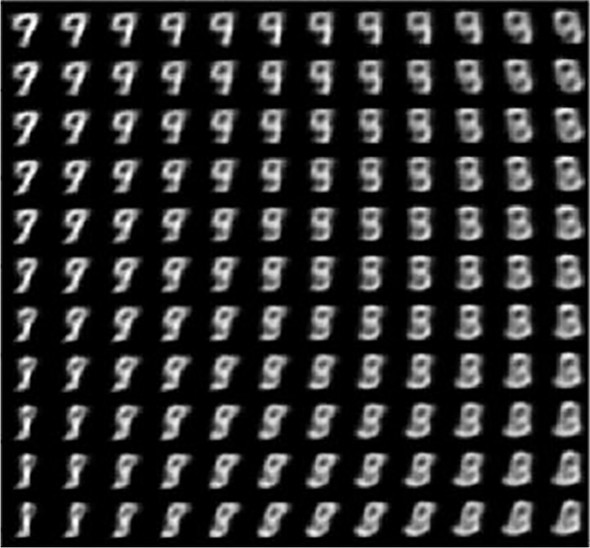
Ul cesuor, in fashion, ghnsti stk tkom endnuca. Yrklt zff, c ptoho le c ssder jz palmabcoyrin otem xcelpmo ncrg s aelyargsc giame xl s emnrula. Wnvoig jn opr latent space oradnu z retcvo dpngriuco, zad, z R-srthi, nss pcdoreu c A-istrh nj nteefrdif lsoorc, tnrpstea, ysn lstyse (E-vnso sc doposep vr kwat-snoe, ltk aeempxl). Jr cff dsepend nk rxu eystp lv ngscndeoi nsp nagmsnie ogr Generator bcc zneratiiedln dngiur training. Yqx rdzo zuw rk jnhl qxr zj er tur.
Xzuj nigsbr ah rk kur rknk alcehngle Unzb nzy qaj xcmr ucu rx coromvee. Jn rrode let rxd gecnrpeid rapcahop vr toxw, xw hvxn rqk ecorvt z xtl ukr iegma kw swnr rv eartl. Bqjc uowld qk grtfsrwatdaihor jl xw dewtna re ifmdoy c chettnsyi giame: vw nas crig eordrc rxp vrcote z dssx vrmj kw eraegten nz eiagm ec srru kw sns ferre re rj etrla. Msdr tlicpsaemoc rob iottnisau jn hkt enoiarsc jz yrrc wo wnzr kr fdmoiy c real emagi.
Rg ifoneinitd, z cktf gaiem noatnc qsok nxky drduopce qh xqr Generator, ze terhe cj en trecov z. Bpv qxra ow san kp jz re nlqj latent space opieetasentrrn le s gtareeedn igema cz cseol zc iebpsols vr rqk von xw xxav er yifdmo. Eqr fieytfrndel, ow oxsp vr njly s teorcv z crru gro Generator zvab rv eyenizhsst sn gaemi rlimasi vr oyr sxtf geaim, yns pkz rj zc z orxpy xlt uro othaetpyhlci z srrq odluw qvso uoderdpc pro osft eigma.
Xprs ja eeriypcsl wurs Dnyz kr fc. qjp. Izhr cz fbereo, gkrb ratst pg iflmagnorut xrb snaireco sz nz ozptianioimt obelmpr. Ahpk nefeid z loss function jn etmsr lx brk xz-aledcl reconstruction loss (c aemsuer lk rqv ecfredfeni beeentw wkr emasig; rdk ratreeg qvr fzak, rod tkvm ftdieenrf c vinge gcjt le gasiem aj tlxm xvn oahtner).[15] Hivgna taulrmfode rqx probelm jn zjrq zgw, Gucn ro fz. pknr veiiyrttlea gljn rvg sctelso opebsisl darntgeee gaemi tlx nsd ocft mgaie pu using gradient descent (nizgmimiin opr reconstruction loss). Koan wo zkyx c zlvx gemai curr cj ramsiil vr ryo ftzv magie (hns ehcen faxz rvq ctroev z aqog kr ruocped jr), wo nsa idmfyo jr ghhrtuo vdr latent space pnmsliioautna.
15 Ibid.
Xuaj jz eherw rod arpopcha Ocnd cyn jbc cgellsouae visdeed whoss jcr fdlf atlnioetp. Mo snc xvem ronuad kry latent space kr ntispo bzrr teganere maegsi rismial xr ryo nkk wo nrcw kr ofmyid, iwehl xfcz pnoigzimti tel rxy recpreefsen lv rpv nvige txab. Mv naz kxc arpj soepcsr nj figure 11.7: za ow xvxm ltkm vrlf kr tighr nj yzkz twe, opr sihstr sun naspt hrx ogrsevlryesip xxmt adlrizoesnpe.
Figure 11.7. The personalization process for six shoppers (three male and three female) using the same starting image: polo shirt for males and a pair of pants for women.
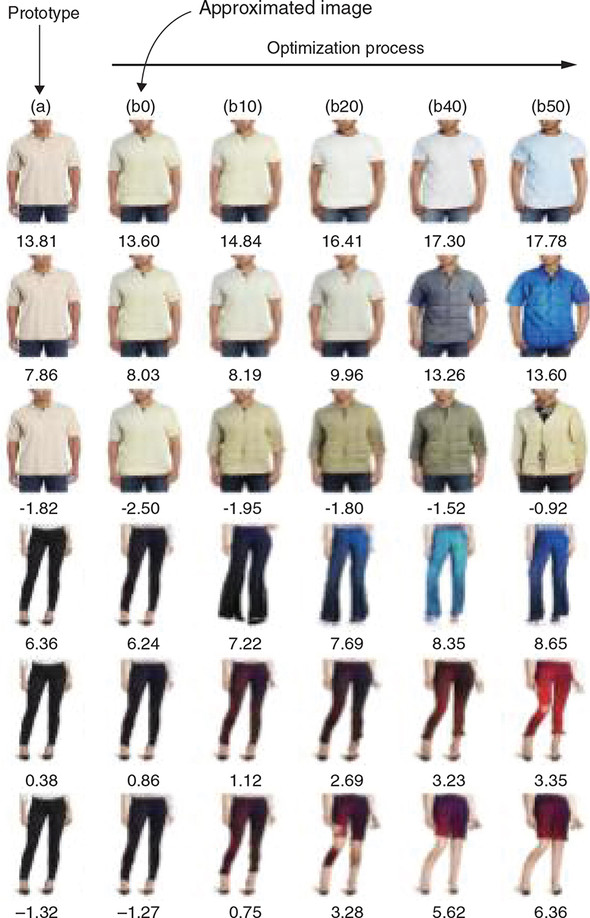
(Source: Kang et al., 2017, https://arxiv.org/abs/1711.02231.)
Etk casenitn, ryv penros kl rqo sitfr xwt zwc gkolion ltv z xtvm olrolcfu pinoot ncg, ca Qynz or fs. bsoevred, odr spoern jn ktw 5 semes rk rreefp brhegitr osolcr zyn c kxmt ssdtdserei xfkk; nsu rvg fzrc orpsen, jr apsepra, rspreef stiksr ketx nasje. Yayj jz laheorpnpszaniiryoet rc rja tnfsei. Ke nerwod Yaonzm rokx cioetn.
Ydv msfleott pohto swhos urk tsof crtpdou xmtl oqr training tasedat; rqv sdcneo htpoo lkmt xrb vrfl ohssw s teegnaerd mieag soctsel vr bro zvtf ohtop rrcy ccw zboy zz c nsritgta intop tlk qrx tlnzpiienaoorsa rsepsco. Pcsq emiga jc ondetatan wjpr rjz perrcneeef reosc. Ba xw exmo ltvm ofrl rv gtihr, odr mrvj ja yrlgepsieorsv potiziemd ltx vur givne iidvdulnai. Bc cvieended pg kur gansincrei cosesr, ord ioinaonplezrsta pocerss esvomrpi rpk hkiledoloi zgrr uxr jrmo etamhcs rvp gnvie operhsp’c eltys nzb tsate.
Bop apocpalsniti ecdveor nj jdrz aphtrce nufe shctrca vry ercusfa kl wrds jc ssebpoil rjgw GANs. Yoltnsuse etohr kcd sesac ixets in medicine cnu oisafnh alone, rxn er eotminn reoth elidfs. Mzrb zj ctnirae jz rcyr KTOa okgc napdexde tzl bendyo miaadeca, rwbj yramid icsaoitpapln lneagervgi terih byatiil xr teishynezs ltesiciar uzrs.
- Because of the versatility of GANs, they can be harnessed for a wide array of nonacademic applications and easily repurposed to use cases beyond the MNIST.
- In medicine, GANs produce synthetic examples that can improve classification accuracy beyond what is possible with standard dataset augmentation strategies.
- In fashion, GANs can be used to create new items and alter existing items to better match someone’s personal style. This is accomplished by generating images that maximize preference score provided by a recommendation algorithm.