In this chapter
- types of errors a model can make: false positives and false negatives
- putting these errors in a table: the confusion matrix
- what are accuracy, recall, precision, F-score, sensitivity, and specificity, and how are they used to evaluate models
- what is the ROC curve, and how does it keep track of sensitivity and specificity at the same time
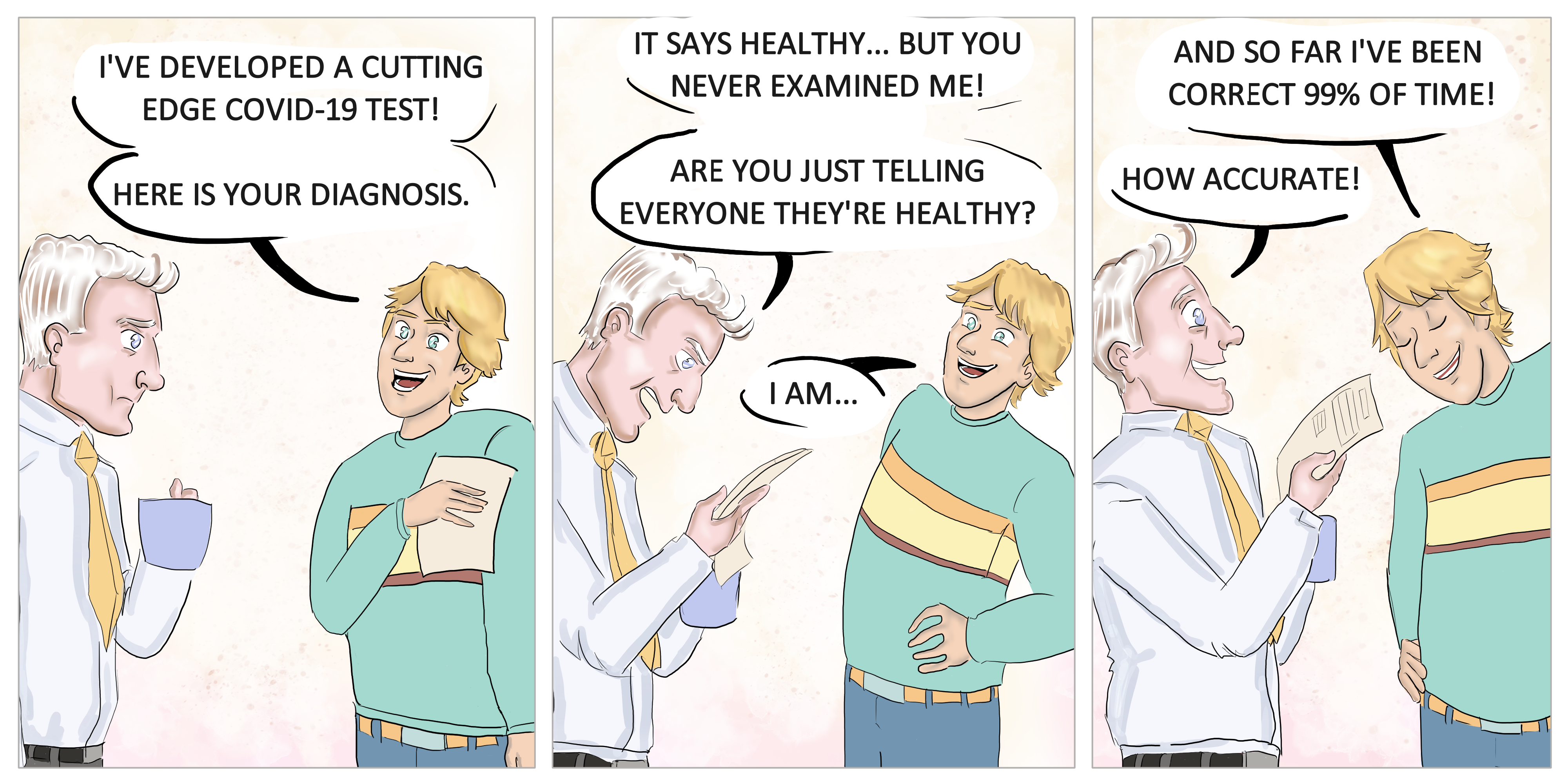
This chapter is slightly different from the previous two—it doesn’t focus on building classification models; instead, it focuses on evaluating them. For a machine learning professional, being able to evaluate the performance of different models is as important as being able to train them. We seldom train a single model on a dataset; we train several different models and select the one that performs best. We also need to make sure models are of good quality before putting them in production. The quality of a model is not always trivial to measure, and in this chapter, we learn several techniques to evaluate our classification models. In chapter 4, we learned how to evaluate regression models, so we can think of this chapter as its analog but with classification models.