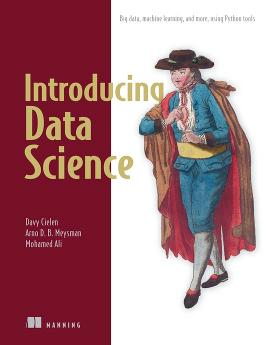
About this Book
I can only show you the door. You’re the one that has to walk through it.
Morpheus, The Matrix
Welcome to the book! When reading the table of contents, you probably noticed the diversity of the topics we’re about to cover. The goal of Introducing Data Science is to provide you with a little bit of everything—enough to get you started. Data science is a very wide field, so wide indeed that a book ten times the size of this one wouldn’t be able to cover it all. For each chapter, we picked a different aspect we find interesting. Some hard decisions had to be made to keep this book from collapsing your bookshelf!
We hope it serves as an entry point—your doorway into the exciting world of data science.
Chapters 1 and 2 offer the general theoretical background and framework necessary to understand the rest of this book:
- Chapter 1 is an introduction to data science and big data, ending with a practical example of Hadoop.
- Chapter 2 is all about the data science process, covering the steps present in almost every data science project.
In chapters 3 through 5, we apply machine learning on increasingly large data sets:
- Chapter 3 keeps it small. The data still fits easily into an average computer’s memory.
- Chapter 4 increases the challenge by looking at “large data.” This data fits on your machine, but fitting it into RAM is hard, making it a challenge to process without a computing cluster.
- Chapter 5 finally looks at big data. For this we can’t get around working with multiple computers.
Chapters 6 through 9 touch on several interesting subjects in data science in a more-or-less independent matter:
- Chapter 6 looks at NoSQL and how it differs from the relational databases.
- Chapter 7 applies data science to streaming data. Here the main problem is not size, but rather the speed at which data is generated and old data becomes obsolete.
- Chapter 8 is all about text mining. Not all data starts off as numbers. Text mining and text analytics become important when the data is in textual formats such as emails, blogs, websites, and so on.
- Chapter 9 focuses on the last part of the data science process—data visualization and prototype application building—by introducing a few useful HTML5 tools.
Appendixes A–D cover the installation and setup of the Elasticsearch, Neo4j, and MySQL databases described in the chapters and of Anaconda, a Python code package that’s especially useful for data science.
This book is an introduction to the field of data science. Seasoned data scientists will see that we only scratch the surface of some topics. For our other readers, there are some prerequisites for you to fully enjoy the book. A minimal understanding of SQL, Python, HTML5, and statistics or machine learning is recommended before you dive into the practical examples.
We opted to use the Python script for the practical examples in this book. Over the past decade, Python has developed into a much respected and widely used data science language.
The code itself is presented in a fixed-width font like this to separate it from ordinary text. Code annotations accompany many of the listings, highlighting important concepts.
The book contains many code examples, most of which are available in the online code base, which can be found at the book’s website, https://www.manning.com/books/introducing-data-science.