This chapter covers
- An introduction to machine learning
- Training and applying decision tree classifiers in parallel with PySpark
- Matching problems and appropriate machine learning algorithms
- Training and applying random forest regressors with PySpark
Chapter 9 showed how we can write Python and take advantage of Spark, one of the most popular distributed computing frameworks. We saw some of Spark’s raw data transformation options, and we used Spark in the map and reduce style we’ve been exploring throughout the book. However, one of the reasons why Spark is so popular is its built-in machine learning capabilities.
Machine learning refers to the design, training, application, and study of judgmental algorithms that adjust themselves based on input data. A familiar example of machine learning is the spam filter. Spam filter designers feed spam into their spam filter algorithms, which either are or contain machine learning algorithms. Then the spam filter algorithm learns to make judgments about whether or not an email is spam (figure 10.1).
Figure 10.1. Spam filters are machine learning algorithms that learn how to judge emails as spam or not by looking at lots of spam emails and nonspam emails.
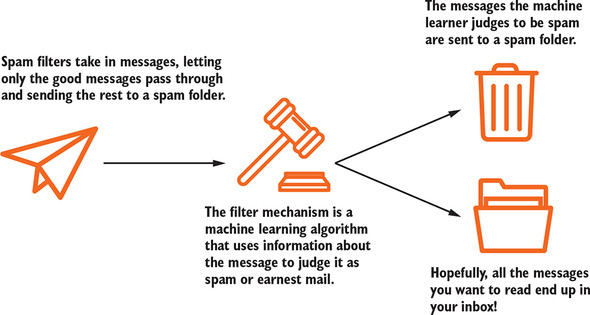
In this chapter, we’ll look at how to use PySpark for machine learning. First, we’ll explore what machine learning is in greater depth. Then we’ll build two machine learners in PySpark: