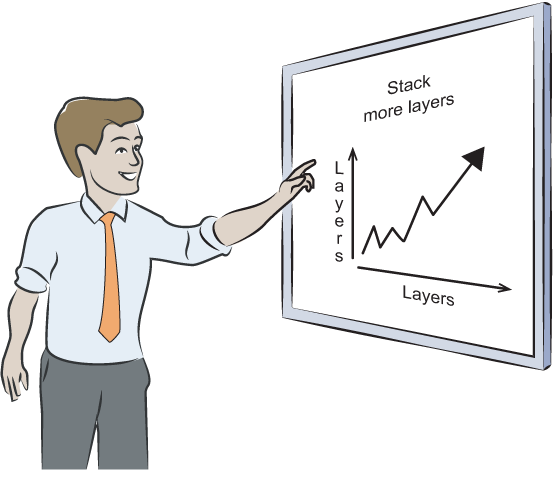
The vast majority of DL models are based on one or a combination of three types of layers: fully connected, convolutional, and recurrent. The success of a DL model depends in great part on choosing the right architecture for the problem at hand.
If you want to analyze data that has no structure, like tabular data in Excel sheets, then you should consider fully connected networks. If the data has a special local structure like images, then convolutional neural networks (NNs) are your friends. Finally, if the data is sequential like text, then the easiest option is to use 1D convolutional networks. This chapter gives you an overview of the different architectures used in DL and provides hints as to when to use which architectural type.