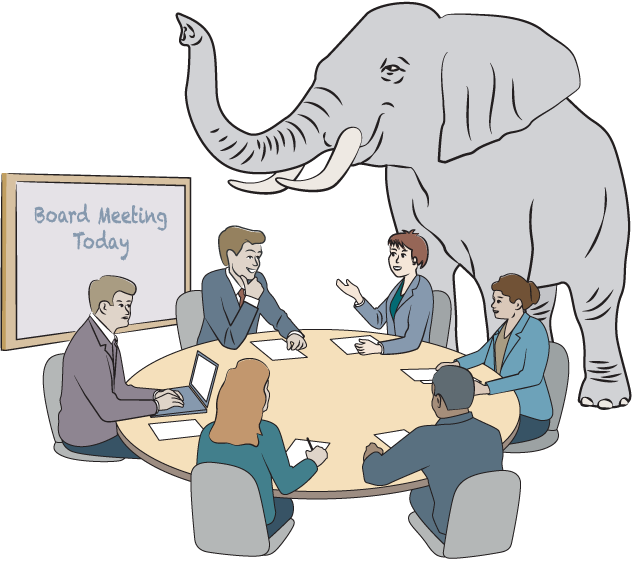
This chapter introduces Bayesian models. Besides the likelihood approach, the Bayesian approach is the most important method to fit the parameters of a probabilistic model and to estimate the associated parameter uncertainty. The Bayesian modeling approach incorporates an additional kind of uncertainty, called epistemic uncertainty. You will see that incorporating epistemic uncertainty results in better prediction performance and, more appropriately, quantification of the uncertainty of the predicted outcome distribution. The epistemic uncertainty becomes especially important when applying prediction models to situations not seen during the training. In regression, this is known as extrapolation.