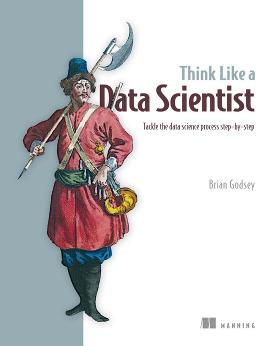
Data science still carries the aura of a new field. Most of its components—statistics, software development, evidence-based problem solving, and so on—descend directly from well-established, even old, fields, but data science seems to be a fresh assemblage of these pieces into something that is new, or at least feels new in the context of current public discourse.
Like many new fields, data science hasn’t quite found its footing. The lines between it and other related fields—as far as those lines matter—are still blurry. Data science may rely on, but is not equivalent to, database architecture and administration, big data engineering, machine learning, or high-performance computing, to name a few.
The core of data science doesn’t concern itself with specific database implementations or programming languages, even if these are indispensable to practitioners. The core is the interplay between data content, the goals of a given project, and the data-analytic methods used to achieve those goals. The data scientist, of course, must manage these using any software necessary, but which software and how to implement it are details that I like to imagine have been abstracted away, as if in some distant future reality.