concept combiner in category hadoop
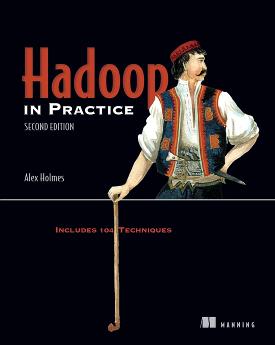
This is an excerpt from Manning's book Hadoop in Practice, Second Edition.
The combiner is a powerful mechanism that aggregates data in the map phase to cut down on data sent to the reducer. It’s a map-side optimization, where your code is invoked with a number of map output values for the same output key.
The combiner is invoked on the map side as part of writing map output data to disk in both the spill and merge phases, as shown in figure 8.5. To help with grouping values together to maximize the effectiveness of a combiner, use a sorting step in both phases prior to calling the combiner function.
Calling the setCombinerClass sets the combiner for a job, similar to how the map and reduce classes are set:
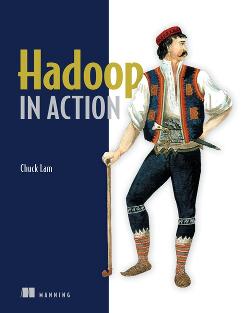
This is an excerpt from Manning's book Hadoop in Action.
In many situations with MapReduce applications, we may wish to perform a “local reduce” before we distribute the mapper results. Consider the WordCount example of chapter 1 once more. If the job processes a document containing the word “the” 574 times, it’s much more efficient to store and shuffle the pair (“the”, 574) once instead of the pair (“the”, 1) multiple times. This processing step is known as combining. We explain combiners in more depth in section 4.6.
Hadoop solves these bottlenecks by extending the MapReduce framework with a combiner step in between the mapper and reducer. You can think of the combiner as a helper for the reducer. It’s supposed to whittle down the output of the mapper to lessen the load on the network and on the reducer. If we specify a combiner, the MapReduce framework may apply it zero, one, or more times to the intermediate data. In order for a combiner to work, it must be an equivalent transformation of the data with respect to the reducer. If we take out the combiner, the reducer’s output will remain the same. Furthermore, the equivalent transformation property must hold when the combiner is applied to arbitrary subsets of the intermediate data.
If the reducer only performs a distributive function, such as maximum, minimum, and summation (counting), then we can use the reducer itself as the combiner. But many useful functions aren’t distributive. We can rewrite some of them, such as averaging to take advantage of a combiner.
The averaging approach taken by AverageByAttributeMapper.py is to output only each key/value pair. AverageByAttributeReducer.py will count the number of key/value pairs it receives and sum up their values, in order for a single final division to compute the average. The main obstacle to using a combiner is the counting operation, as the reducer assumes the number of key/value pairs it receives is the number of key/value pairs in the input data. We can refactor the MapReduce program to track the count explicitly. The combiner becomes a simple summation function with the distributive property.
Let’s first refactor the mapper and reducer before writing the combiner, as the operation of the MapReduce job must be correct even without a combiner. We write the new averaging program in Java as the combiner must be a Java class.
Programmatically, the combiner must implement the Reducer interface. The combiner’s reduce() method performs the combining operation. This may seem like a bad naming scheme, but recall that for the important class of distributive functions, the combiner and the reducer perform the same operations. Therefore, the combiner has adopted the reducer’s signature to simplify its reuse. You don’t have to rename your Reduce class to use it as a combiner class. In addition, because the combiner is performing an equivalent transformation, the type for the key/value pair in its output must match that of its input. In the end, we’ve created a Combine class that looks similar to the Reduce class, except it only outputs the (partial) sum and count at the end, whereas the reducer computes the final average.
To enable the combiner, the driver must specify the combiner’s class to the JobConf object. You can do this through the setCombinerClass() method. The driver sets the mapper, combiner, and the reducer:
A combiner doesn’t necessarily improve performance. You should monitor the job’s behavior to see if the number of records outputted by the combiner is meaningfully less than the number of records going in. The reduction must justify the extra execution time of running a combiner. You can easily check this through the JobTracker’s Web UI, which we’ll see in chapter 6.
Looking at figure 4.5, note that in the map phase, combine has 1,984,625 input records and only 1,063 output records. Clearly the combiner has reduced the amount of intermediate data. Note that the reduce side executes the combiner, though the benefit of this is negligible in this case.
Table 4.5. Monitoring the effectiveness of the combiner in the AveragingWithCombiner job
Counter
Map
Reduce
Total
Job Counters Data-local map tasks 0 0 4 Launched reduce tasks 0 0 2 Launched map tasks 0 0 4 Map-Reduce Framework Reduce input records 0 151 151 Map output records 1,984,055 0 1,984,055 Map output bytes 18,862,764 0 18,862,764 Combine output records 1,063 151 1,214 Map input records 2,923,923 0 2,923,923 Reduce input groups 0 151 151 Combine input records 1,984,625 493 1,985,118 Map input bytes 236,903,179 0 236,903,179 Reduce output records 0 151 151 File Systems HDFS bytes written 0 2.658 2,658 Local bytes written 20,554 2,510 23,064 HDFS bytes read 236,915,470 0 236,915,470 Local bytes read 21,112 2,510 23,622