concept dictionary in category machine learning
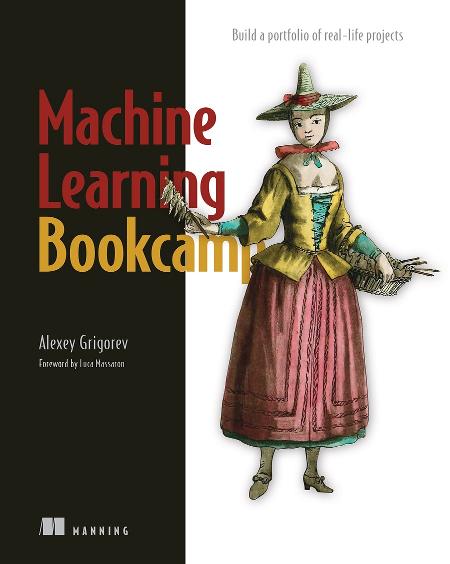
This is an excerpt from Manning's book Machine Learning Bookcamp MEAP V06.
As the name suggests, DictVectorizer takes in a dictionary and vectorizes it — that is, creates vectors from it. Then the vectors are put together as rows of one matrix. This matrix is used as input to a machine learning algorithm (figure 3.23).
Figure 3.23 The process of creating a model. First, we convert a dataframe to a list of dictionaries; then we vectorize the list to a matrix; and finally, we use the matrix to train a model.
![]()
To use it, we need to convert our dataframe to a list of dictionaries. It's very simple to do in Pandas. Use the to_dict method with the orient="rows" parameter:
Figure 3.23 The process of creating a model. First, we convert a dataframe to a list of dictionaries; then we vectorize the list to a matrix; and finally, we use the matrix to train a model.
![]()
Collections are special containers that allow keeping multiple elements in them. We will look at four types of collections: lists, tuples, sets, and dictionaries.
To avoid it, we can first check if the key is in the dictionary before attempting to get the value. We can use the “in” statement for checking it:
if 'five' in words_to_numbers: print(words_to_numbers['five']) else: print('not in the dictionary')When running this code, we'll see “not in the dictionary” in the output.
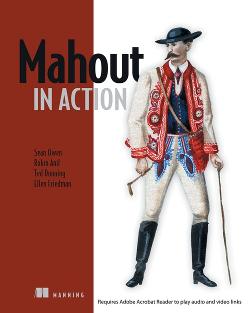
This is an excerpt from Manning's book Mahout in Action.
Table 8.2. Important flags for the Mahout dictionary-based vectorizer and their default values
Option
Flag
Description
Default value
Overwrite (bool) -ow If set, the output folder is overwritten. If not set, the output folder is created if the folder doesn’t exist. If the output folder does exist, the job fails and an error is thrown. Default is unset. N/A Lucene analyzer name (String) -a The class name of the analyzer to use. org.apache.lucene
.analysis.standard
.StandardAnalyzerChunk size (int) -chunk The chunk size in MB. For large document collections (sizes in GBs and TBs), you won’t be able to load the entire dictionary into memory during vectorization, so you can split the dictionary into chunks of the specified size and perform the vectorization in multiple stages. It’s recommended you keep this size to 80 percent of the Java heap size of the Hadoop child nodes to prevent the vectorizer from hitting the heap limit. 100 Weighting (String) -wt The weighting scheme to use: tf for term-frequency based weighting and tfidf for TFIDF based weighting. tfidf Minimum support (int) -s The minimum frequency of the term in the entire collection to be considered as a part of the dictionary file. Terms with lesser frequency are ignored. 2 Minimum document frequency (int) -md The minimum number of documents the term should occur in to be considered a part of the dictionary file. Any term with lesser frequency is ignored. 1 Max document frequency percentage (int) -x The maximum number of documents the term should occur in to be considered a part of the dictionary file. This is a mechanism to prune out high frequency terms (stop-words). Any word that occurs in more than the specified percentage of documents is ignored. 99 N-gram size (int) -ng The maximum size of n-grams to be selected from the collection of documents. 1 Minimum log-likelihood ratio (LLR) (float) -ml This flag works only when n-gram size is greater than 1. Very significant n-grams have large scores, such as 1000; less significant ones have lower scores. Although there’s no specific method for choosing this value, the rule of thumb is that n-grams with a LLR value less than 1.0 are irrelevant. 1.0 Normalization (float) -n The normalization value to use in the Lp space. A detailed explanation of normalization is given in section 8.4. The default scheme is to not normalize the weights. 0 Number of reducers (int) -nr The number of reducer tasks to execute in parallel. This flag is useful when running a dictionary vectorizer on a Hadoop cluster. Setting this to the maximum number of nodes in the cluster gives maximum performance. Setting this value higher than the number of cluster nodes leads to a slight decrease in performance. For more details, read the Hadoop documentation on setting the optimum number of reducers. 1 Create sequential access sparse vectors (bool) -seq If set, the output vectors are created as SequentialAccessSparseVectors. By default the dictionary vectorizer generates RandomAccessSparseVectors.
The former gives higher performance on certain algorithms like k-means and SVD due to the sequential nature of vector operations. By default the flag is unset.N/A
The Last.fm data set already looks like it can be directly converted to vectors. We create a simple vectorizer that first generates a dictionary of artists, and, using the dictionary, convert the artists into integer dimensions in a MapReduce fashion. We could have used the strings as IDs, but Mahout Vector format only takes integer dimensions. The reason for converting artists into integer dimensions will be clear once we arrive at the code that vectorizes Last.fm artists into vectors of their tags.
To generate these feature vectors for the Last.fm data set, we employ two MapReduce jobs. The first generates the unique artists in the form of a dictionary, and the second generates vectors from the data using the generated dictionary. The Mapper and Reducer classes of the dictionary generation code are shown in listings 12.4, 12.5 and 12.6.
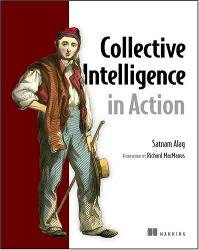
This is an excerpt from Manning's book Collective Intelligence in Action.
In the next release, they allowed the users to explicitly tag items by adding free text labels, along with saving or bookmarking items of interest. As users started tagging items, John and Jane found that there was a rich set of information that could be derived. First of all, users were providing new terms for the content that made sense to them—in essence they built folksonomies.[4] The tag cloud navigation now had both machine-generated and user-generated tags. The process of extracting tags using an automated algorithm could also be enhanced using the dictionary of tags built by the users. These user-added tags were also useful for finding keywords used by an ad-generation engine. They could also use the tags created by users to connect users with each other and with other items of interest. This is collective intelligence in action.
The tags associated with your application define the set of terms that can be used to describe the user and the items. This in essence is the vocabulary for your application. Folksonomies are built from user-generated tags. Automated algorithms have a difficult time creating multi-term tags. When a dictionary of tags is available for your application, automated algorithms can use this dictionary to extract multi-term tags. Well-developed ontologies, such as in the life sciences, along with folksonomies are two of the ways to generate a dictionary of tags in an application.