concept hmm in category machine learning
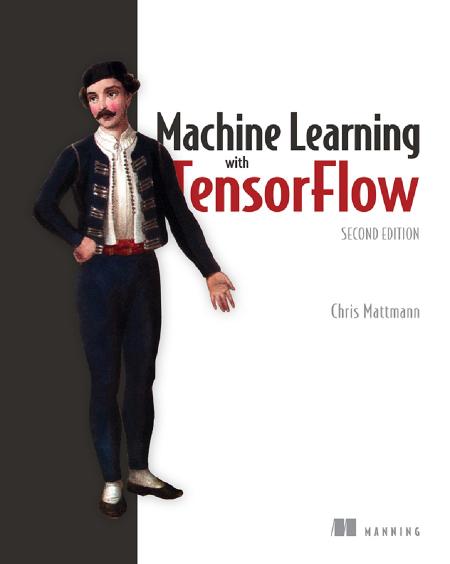
This is an excerpt from Manning's book Machine Learning with TensorFlow, Second Edition MEAP V08.
Before going into detail about Markov chains and HMMs, let’s consider alternative models. In the next section, you’ll see models that may not be interpretable.
Given this construction, you can build your initial, transition, and emission matrices, and run your HMM using the TensorFlow code in listing 10.1 and the Viterbi algorithm, which can tell you the actual hidden states given observed states. In this case, the hidden states are the true PoS tags, and the observed tags are the ambiguity-class PoS tags. Take a look at figure 10.6 for a summary of the discussion and for pinpointing how TensorFlow and HMMs can help you to disambiguate text.
Figure 10.6 The training and prediction steps for the HMM-based part of speech tagger. Both steps require input text (sentences), and the PoS tagger will annotate them ambiguously. In the training portion, humans provide unambiguous PoS for the input sentences to train the HMM on. In the prediction stage, the HMM predicts the unambiguous PoS. These form the three needed corpora to build an HMM.
![]()
HMMs are explainable models that accumulate probabilistic evidence and help guide decisions based on possible states that that evidence represents.