concept pipeline in category nlp
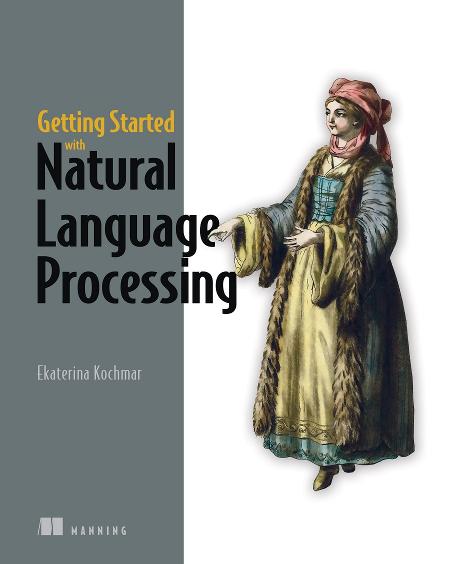
This is an excerpt from Manning's book Getting Started with Natural Language Processing MEAP V06.
Unlike NLTK that treats different components of language analysis as separate steps, spaCy builds an analysis pipeline from the very beginning and applies this pipeline to text. Under the hood, the pipeline already includes a number of useful NLP tools that are run on input text without you needing to call on them separately. These tools include, among others, a tokenizer and a POS tagger. You simply apply the whole lot of tools with a single line of code calling on the spaCy processing pipeline, and then your program stores the result in a convenient format until you need it. This also ensures that the information is passed between the tools without you taking care of the input-output formats. Figure 4.13 visualizes spaCy’s NLP pipeline, that we are going to discuss in more detail next:
Sklearn’s pipeline is highly customizable and it allows you to bolt together various tools and subsequently run them with a single line of code (i.e., invoking the pipeline when needed). It makes it easy to experiment with different settings of the tools and find out what works best. So, let’s find out how the pipeline works. Code Listing 8.10 shows how to define a pipeline:
Listing 8.10 Code to define Pipeline from sklearn.pipeline import Pipeline #A from sklearn.preprocessing import Binarizer #B text_clf = Pipeline([('vect', CountVectorizer(min_df=10, max_df=0.5)), ('binarizer', Binarizer()), ('clf', MultinomialNB()), ]) #C text_clf.fit(train_data, train_targets) print(text_clf) #D predicted = text_clf.predict(test_data) #ENote that instead of defining the tools one by one and passing the output of one tool as the input to the next tool, you simply pack them up under the Pipeline and after that you don’t need to worry anymore about the flow of the information between the bits of the pipeline. In other words, you can train the whole model applying fit method as before (which will use the whole set of tools this time) and then test it on the test set using predict method. Figure 8.12 is thus an update on Figure 8.11:
Figure 8.16 You can iterate on the final steps in the pipeline updating your algorithm with new features
![]()