concept Skip - gram model in category nlp
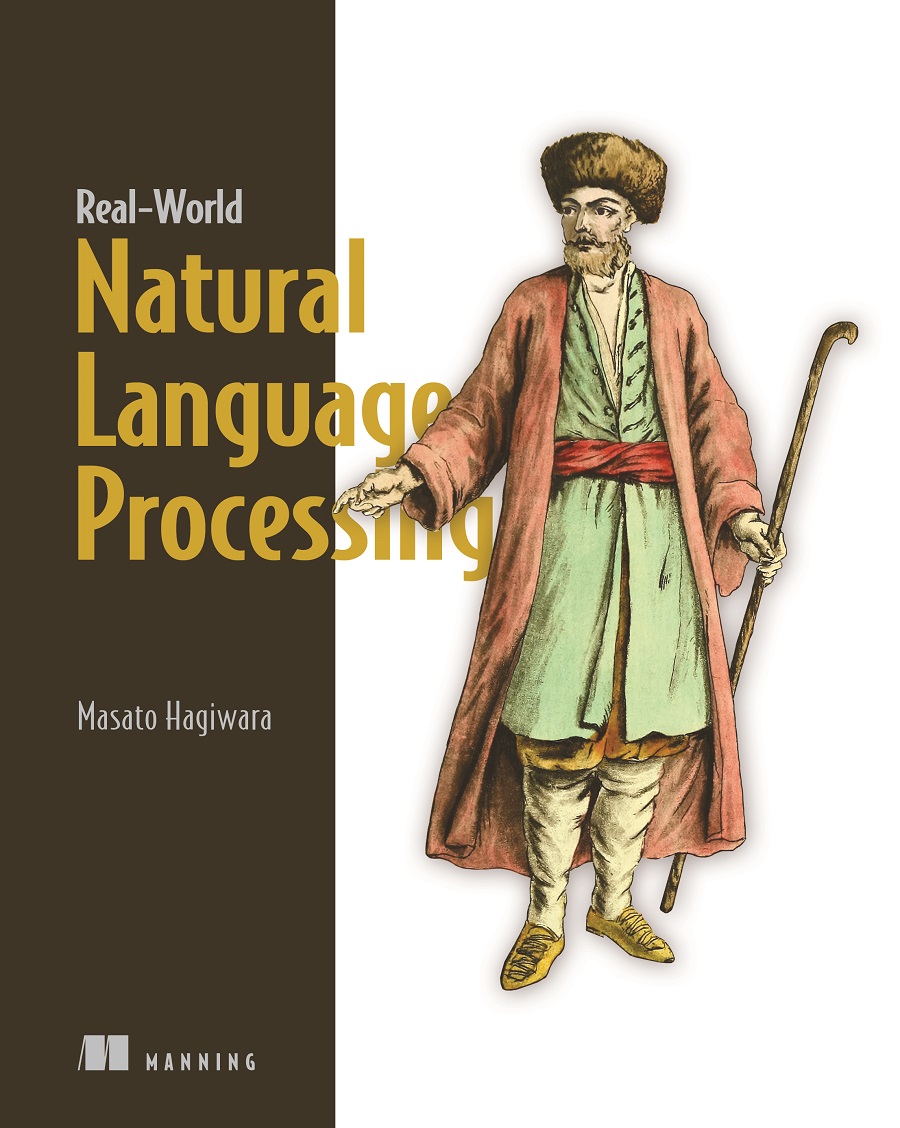
This is an excerpt from Manning's book Real-World Natural Language Processing MEAP V06.
Putting all together, the structure we want for the Skip-gram model is shown in Figure 3.5. This network is very simple. It takes a word embedding as an input and expands it via a linear layer to a set of scores, one for each context word. Hopefully this is not as intimidating as many people think!
Finally, you need to implement the body of the Skip-gram model as shown in listing 3.1.
Listing 3.1: Skip-gram model implemented in AllenNLP
class SkipGramModel(Model): #A def __init__(self, vocab, embedding_in): super().__init__(vocab) self.embedding_in = embedding_in #B self.linear = torch.nn.Linear( in_features=EMBEDDING_DIM, out_features=vocab.get_vocab_size('token_out'), bias=False) #C def forward(self, token_in, token_out): #D embedded_in = self.embedding_in(token_in) #E logits = self.linear(embedded_in) #F loss = functional.cross_entropy(logits, token_out) #G return {'loss': loss}
There is another word embedding model that is often mentioned along with the Skip-gram model called continuous bag-of-words, or CBOW, model. As a close sibling of the Skip-gram model proposed at the same time (http://realworldnlpbook.com/ch3.html#mikolov13), the architecture of the CBOW model looks similar to that of the Skip-gram model but flipped upside down. The “fake” task the model is trying to solve is to predict the target word from a set of its context words. This is also similar to fill-in-the-blank type of questions. For example, if you see a sentence “I heard a ___ barking in the distance,” most of you can probably guess the answer “dog” instantly. Figure 3.8 shows the structure of this model.
Figure 3.8: Continuous bag-of-words (CBOW) model
![]()
I’m not going to implement the CBOW model from scratch here for a couple of reasons: It should be straightforward to implement if you understand the Skip-gram model. Also, the accuracy of the CBOW model measured on word semantic tasks is usually slightly lower than that of Skip-gram, and CBOW is less often used in NLP than Skip-gram. Both models are implemented in the original word2vec (https://code.google.com/archive/p/word2vec/) toolkit if you want to try them yourself, although the vanilla Skip-gram and CBOW models are less and less often used nowadays because of the advent of more recent, powerful word embedding models (such as GloVe and FastText) that are covered in the rest of this chapter.