concept token in category nlp
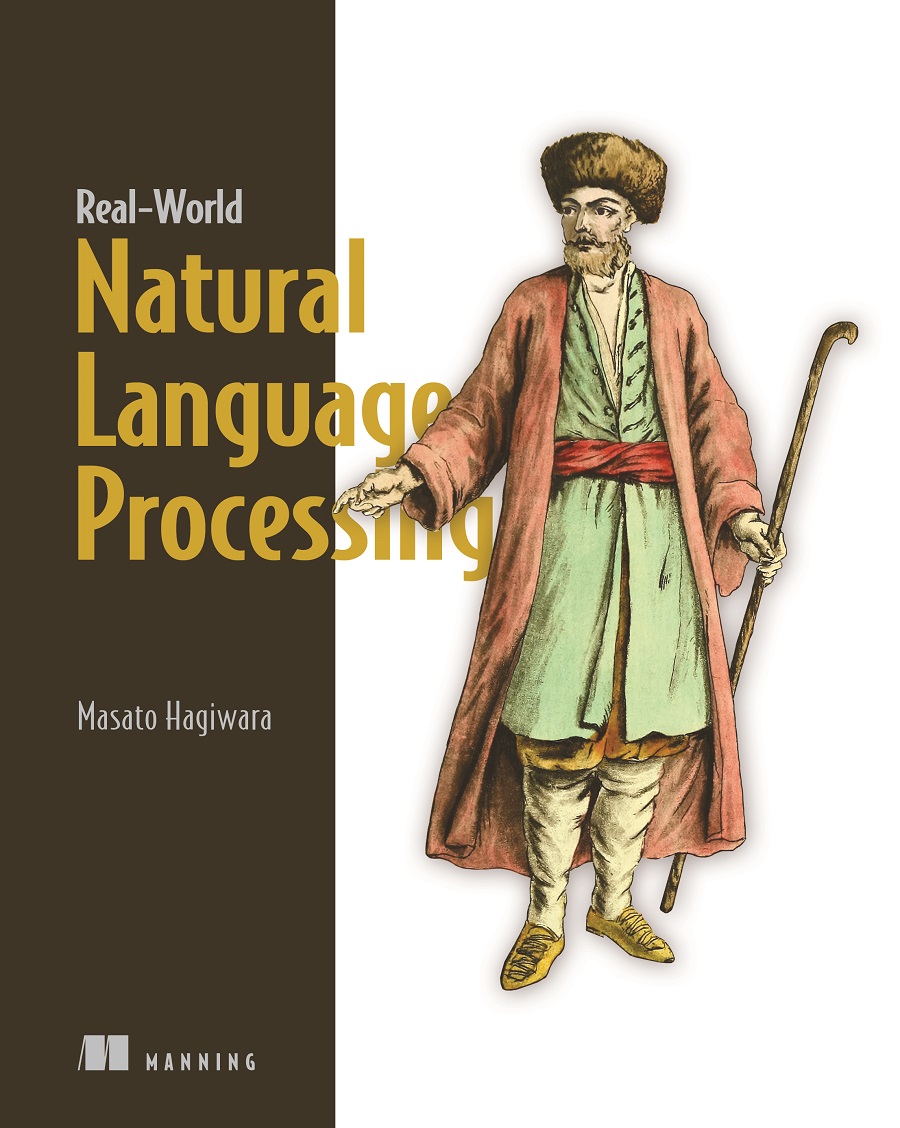
This is an excerpt from Manning's book Real-World Natural Language Processing MEAP V06.
Now you can use word_embeddings to convert words (or more precisely, tokens, which I’ll talk more about in Chapter 3) to their embeddings.
A closely related concept to word in NLP is token. A token is a string of contiguous characters that play a certain role in a written language. Most words (“apple”, “banana”, “zebra”) are also tokens when written. Punctuation marks such as the exclamation mark “!” are tokens but not words, because you can’t utter them in isolation. Word and token are often used interchangeably in NLP. In fact, when you see “word” in NLP text (including this book), it often means “token”, because most NLP tasks only deal with written text that is processed in an automatic way. Tokens are the output of a process called tokenization, which I’ll explain more below.
>>> import spacy >>> nlp = spacy.load('en_core_web_sm') >>> doc = nlp(s) >>> [token.text for token in doc] ['Good', 'muffins', 'cost', '$', '3.88', '\n', 'in', 'New', 'York', '.', ' ', 'Please', 'buy', 'me', 'two', 'of', 'them', '.', '\n\n', 'Thanks', '.'] >>> [sent.string.strip() for sent in doc.sents] ['Good muffins cost $3.88\nin New York.', 'Please buy me two of them.', 'Thanks.']
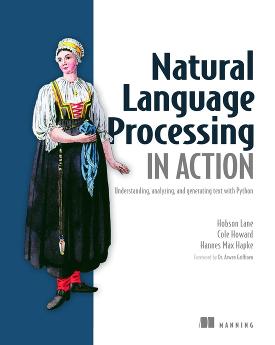
This is an excerpt from Manning's book Natural Language Processing in Action: Understanding, analyzing, and generating text with Python.
But let’s think for a moment about what information has been lost in our effort to count all the words in the messages we receive. We assign the words to bins and store them away as bit vectors like a coin or token sorter directing different kinds of tokens to one side or the other in a cascade of decisions that piles them in bins at the bottom. Our sorting machine must take into account hundreds of thousands if not millions of possible token “denominations,” one for each possible word that a speaker or author might use. Each phrase or sentence or document we feed into our token sorting machine will come out the bottom, where we have a “vector” with a count of the tokens in each slot. Most of our counts are zero, even for large documents with verbose vocabulary. But we haven’t lost any words yet. What have we lost? Could you, as a human, understand a document that we presented you in this way, as a count of each possible word in your language, without any sequence or order associated with those words? I doubt it. But if it was a short sentence or tweet, you’d probably be able to rearrange them into their intended order and meaning most of the time.
In this chapter and the next, we discuss most things in terms of time steps. This isn’t the same thing as individual data samples. We’re referring to a single data sample split into smaller chunks that represent changes over time. The single data sample will still be a piece of text, say a short movie review or a tweet. As before, you’ll tokenize the sentence. But rather than putting those tokens into the network all at once, you’ll pass them in one at a time. This is different than having multiple new document samples. The tokens are still part of one data sample with one associated label.
You can think of t as referring to the token sequence index. So t=0 is the first token in the document and t+1 is the next token in the document. The tokens, in the order they appear in the document, will be the inputs at each time step or token step. And the tokens don’t have to be words. Individual characters work well too. Inputing the tokens one at a time will be substeps of feeding the data sample into the network.
Listing 8.3. Data tokenizer + vectorizer
>>> def tokenize_and_vectorize(dataset): ... tokenizer = TreebankWordTokenizer() ... vectorized_data = [] ... for sample in dataset: ... tokens = tokenizer.tokenize(sample[1]) ... sample_vecs = [] ... for token in tokens: ... try: ... sample_vecs.append(word_vectors[token]) ... except KeyError: ... pass #1 ... vectorized_data.append(sample_vecs) ... return vectorized_data
Ahead of the Dense layer you have a vector that is of shape (number of neurons x 1) coming out of the last time step of the Recurrent layer for a given input sequence. This vector is the parallel to the thought vector you got out of the convolutional neural network in the previous chapter. It’s an encoding of the sequence of tokens. Granted it’s only going to be able to encode the thought of the sequences in relation to the labels the network is trained on. But in terms of NLP, it’s an amazing next step toward encoding higher order concepts into a vector computationally.