In the previous chapter, we investigated various privacy-related threats and vulnerabilities in machine learning (ML) and concepts behind privacy-enhancing technologies. From now on, we will focus on the details of essential and popular privacy-enhancing technologies. The one we will discuss in this chapter and the next is differential privacy (DP).
Differential privacy is one of the most popular and influential privacy protection schemes used in applications today. It is based on the concept of making a dataset robust enough that any single substitution in the dataset will not reveal any private information. This is typically achieved by calculating the patterns of groups within the dataset, which we call complex statistics, while withholding information about individuals in the dataset.
For instance, we can consider an ML model to be complex statistics describing the distribution of its training data. Thus, differential privacy allows us to quantify the degree of privacy protection provided by an algorithm on the (private) dataset it operates on. In this chapter, we’ll look at what differential privacy is and how it has been widely adopted in practical applications. You’ll also learn about its various essential properties.
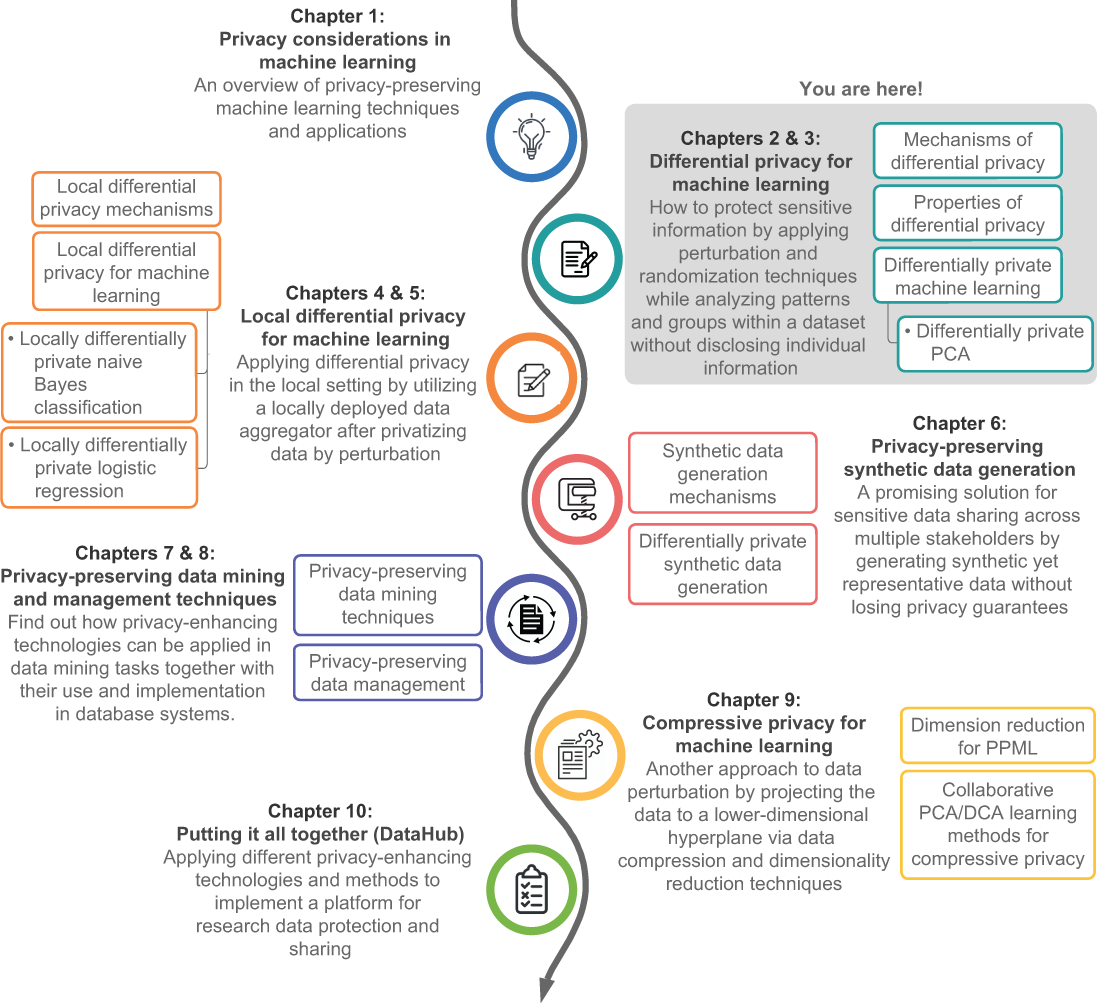