5 Probabilistic deep learning models with TensorFlow Probability
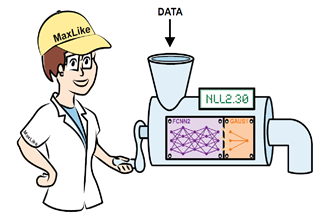
This chapter covers
- Introduction to probabilistic deep learning models
- The negative log-likelihood on new data as a proper performance measure
- Fitting probabilistic deep learning models for continuous and count data
- Creating custom probability distributions
In chapters 3 and 4 you encountered a kind of uncertainty that’s inherent to the data. For example, in chapter 3 you saw in the blood pressure example that two women with the same age can have quite different blood pressures. Even the blood pressure of the same woman can be different when measured at two different times within the same week. To capture this data-inherent variability, we used a conditional probability distribution (CPD): . With this distribution, you captured the outcome variability of y by a model. To refer to this inherent variability in the deep learning (DL) community, the term aleatoric uncertainty is used. The term aleatoric stems from the Latin word alea, which means dice, as in Alea iacta est ("the die is cast").