7 Fine-tuning to follow instructions
This chapter covers
- The instruction fine-tuning process of LLMs
- Preparing a dataset for supervised instruction fine-tuning
- Organizing instruction data in training batches
- Loading a pretrained LLM and fine-tuning it to follow human instructions
- Extracting LLM-generated instruction responses for evaluation
- Evaluating an instruction-fine-tuned LLM
Previously, we implemented the LLM architecture, carried out pretraining, and imported pretrained weights from external sources into our model. Then, we focused on fine-tuning our LLM for a specific classification task: distinguishing between spam and non-spam text messages. Now we’ll implement the process for fine-tuning an LLM to follow human instructions, as illustrated in figure 7.1. Instruction fine-tuning is one of the main techniques behind developing LLMs for chatbot applications, personal assistants, and other conversational tasks.
Figure 7.1 The three main stages of coding an LLM. This chapter focuses on step 9 of stage 3: fine-tuning a pretrained LLM to follow human instructions.
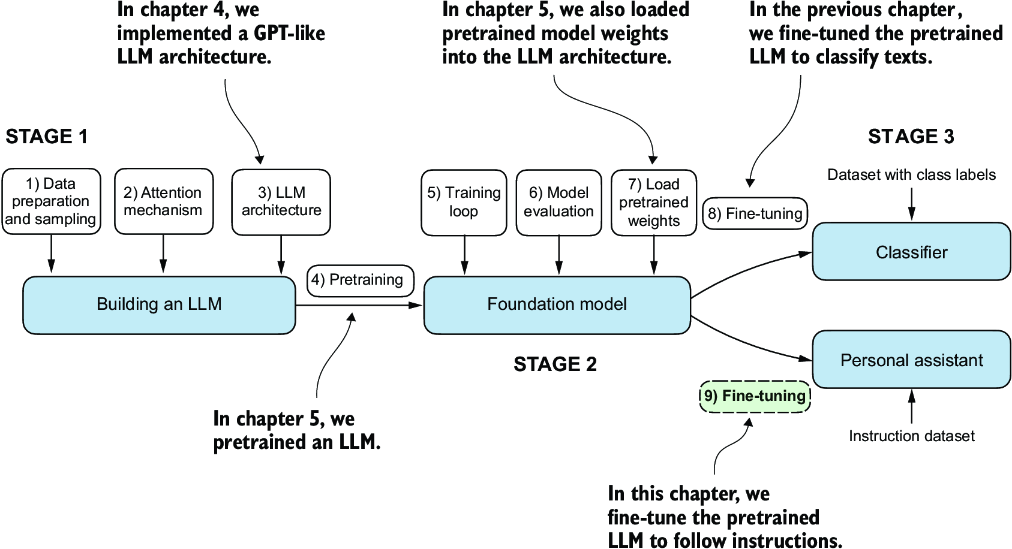
Figure 7.1 shows two main ways of fine-tuning an LLM: fine-tuning for classification (step 8) and fine-tuning an LLM to follow instructions (step 9). We implemented step 8 in chapter 6. Now we will fine-tune an LLM using an instruction dataset.