1 What is deep learning?
This chapter covers
- High-level definitions of fundamental concepts
- A soft introduction to the principles behind machine learning
- Deep learning’s rising popularity and future potential
Throughout the past decade, artificial intelligence (AI) has been a subject of intense media hype. Machine learning, deep learning, and AI come up in countless articles, often outside of technology-minded publications. We’re promised a future of intelligent chatbots, self-driving cars, and virtual assistants – a future sometimes painted in a grim light and other times as utopian, where human jobs will be scarce and most economic activity will be handled by robots or AI agents. For a future or current practitioner of machine learning, it’s important to be able to recognize the signal amid the noise, so that you can tell world-changing developments from overhyped press releases. Our future is at stake, and it’s a future in which you have an active role to play: after reading this book, you’ll be one of those who can develop those AI systems. So let’s tackle these questions: what has deep learning achieved so far? How significant is it? Where are we headed next? Should you believe the hype?
This chapter provides essential context around artificial intelligence, machine learning, and deep learning.
highlight, annotate, and bookmark
You can automatically highlight by performing the text selection while keeping the alt/ key pressed.
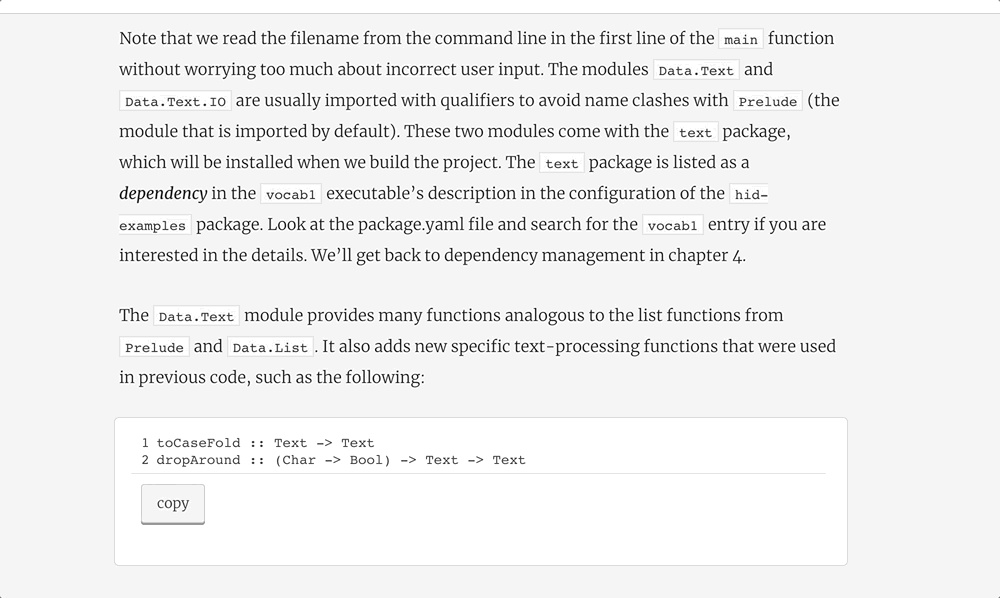
1.1 Artificial intelligence, machine learning, deep learning
First, we need to define clearly what we’re talking about when we mention AI. What are artificial intelligence, machine learning, and deep learning? How do they relate to each other?
Figure 1.1 Artificial intelligence, machine learning, and deep learning HTML: class=''small-image''
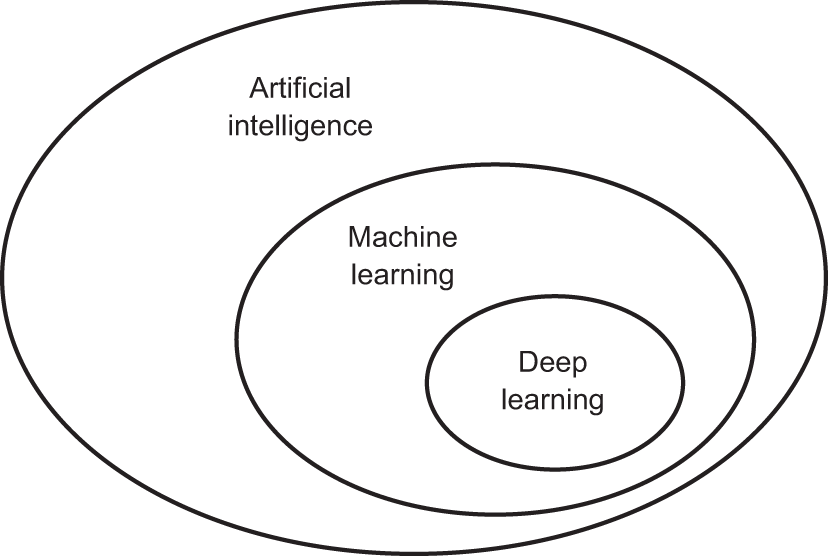
discuss
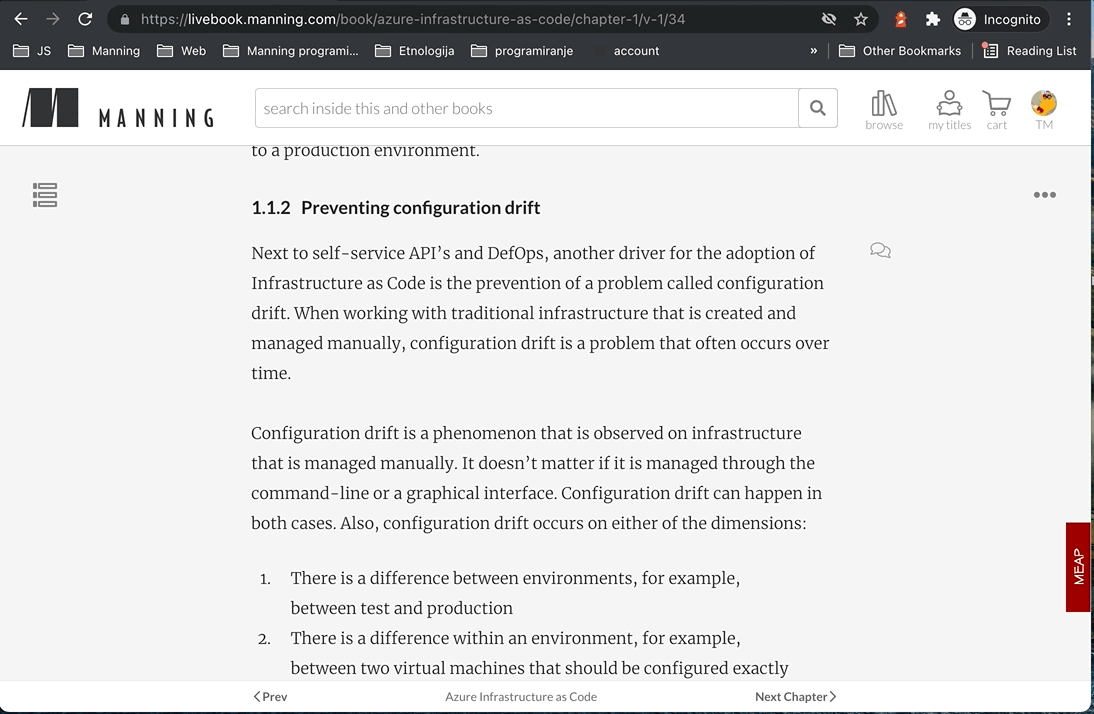
1.2 Artificial intelligence
Artificial intelligence was born in the 1950s, when a handful of pioneers from the nascent field of computer science started asking whether computers could be made to ''think'' – a question whose ramifications we’re still exploring today.
While many of the underlying ideas had been brewing in the years and even decades prior, ''artificial intelligence'' finally crystallized as a field of research in 1956, when John McCarthy, then a young Assistant Professor of Mathematics at Dartmouth College, organized a summer workshop under the following proposal:
The study is to proceed on the basis of the conjecture that every aspect of learning or any other feature of intelligence can in principle be so precisely described that a machine can be made to simulate it. An attempt will be made to find how to make machines use language, form abstractions and concepts, solve kinds of problems now reserved for humans, and improve themselves. We think that a significant advance can be made in one or more of these problems if a carefully selected group of scientists work on it together for a summer. —
At the end of the summer, the workshop concluded without having fully solved the riddle it set out to investigate. Nevertheless, it was attended by many people who would move on to become pioneers in the field, and it set in motion an intellectual revolution that is still ongoing to this day.
Concisely, AI can be described as the effort to automate intellectual tasks normally performed by humans. As such, AI is a general field that encompasses machine learning and deep learning, but that also includes many more approaches that may not involve any learning. Consider that until the 1980s, most AI textbooks didn’t mention ''learning'' at all! Early chess programs, for instance, only involved hardcoded rules crafted by programmers, and didn’t qualify as machine learning. In fact, for a fairly long time, most experts believed that human-level artificial intelligence could be achieved by having programmers handcraft a sufficiently large set of explicit rules for manipulating knowledge stored in explicit databases. This approach is known as symbolic AI. It was the dominant paradigm in AI from the 1950s to the late 1980s, and it reached its peak popularity during the expert systems boom of the 1980s.
Although symbolic AI proved suitable to solve well-defined, logical problems, such as playing chess, it turned out to be intractable to figure out explicit rules for solving more complex, fuzzy problems, such as image classification, speech recognition, or natural language translation. A new approach arose to take symbolic AI’s place: machine learning.
settings
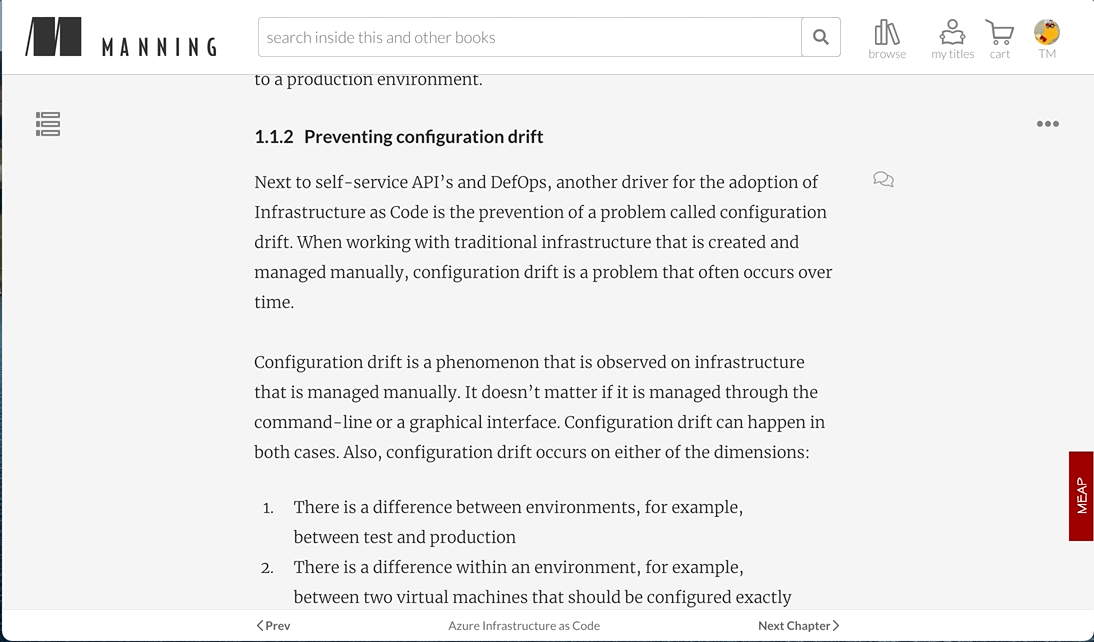
1.3 Machine learning
In Victorian England, Lady Ada Lovelace was a friend and collaborator of Charles Babbage, the inventor of the Analytical Engine: the first-known general-purpose mechanical computer. Although visionary and far ahead of its time, the Analytical Engine wasn’t meant as a general-purpose computer when it was designed in the 1830s and 1840s, because the concept of general-purpose computation was yet to be invented. It was merely meant as a way to use mechanical operations to automate certain computations from the field of mathematical analysis – hence, the name Analytical Engine. As such, it was the intellectual descendant of earlier attempts at encoding mathematical operations in gear form, such as the Pascaline, or Leibniz’s step reckoner, a refined version of the Pascaline. Designed by Blaise Pascal in 1642 (at age 19!), the Pascaline was the world’s first mechanical calculator – it could add, subtract, multiply, or even divide digits.
In 1843, Ada Lovelace remarked on the invention of the Analytical Engine:
The Analytical Engine has no pretensions whatever to originate anything. It can do whatever we know how to order it to perform.… Its province is to assist us in making available what we’re already acquainted with. —
Even with 177 years of historical perspective, Lady Lovelace’s observation remains arresting. Could a general-purpose computer ''originate'' anything, or would it always be bound to dully execute processes we humans fully understand? Could it ever be capable of any original thought? Could it learn from experience? Could it show creativity?
Her remark was later quoted by AI pioneer Alan Turing as ''Lady Lovelace’s objection'' in his landmark 1950 paper ''Computing Machinery and Intelligence,'' [1] which introduced the Turing test [2] as well as key concepts that would come to shape AI. Turing was of the opinion – highly provocative at the time – that computers could, in principle, be made to emulate all aspects of human intelligence.
Figure 1.2 Machine learning: a new programming paradigm HTML: class=''small-image''
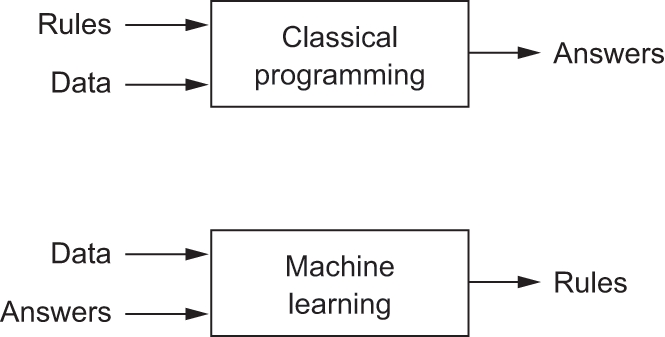
A machine-learning system is trained rather than explicitly programmed. It’s presented with many examples relevant to a task, and it finds statistical structure in these examples that eventually allows the system to come up with rules for automating the task. For instance, if you wished to automate the task of tagging your vacation pictures, you could present a machine-learning system with many examples of pictures already tagged by humans, and the system would learn statistical rules for associating specific pictures to specific tags.
Although machine learning only started to flourish in the 1990s, it has quickly become the most popular and most successful subfield of AI, a trend driven by the availability of faster hardware and larger datasets. Machine learning is related to mathematical statistics, but it differs from statistics in several important ways – in the same sense that medicine is related to chemistry, but cannot be reduced to chemistry, as medicine deals with its own distinct systems with their own distinct properties. Unlike statistics, machine learning tends to deal with large, complex datasets (such as a dataset of millions of images, each consisting of tens of thousands of pixels) for which classical statistical analysis such as Bayesian analysis would be impractical. As a result, machine learning, and especially deep learning, exhibits comparatively little mathematical theory – maybe too little – and is fundamentally an engineering discipline. Unlike theoretical physics or mathematics, machine learning is a very hands-on field driven by empirical findings and deeply reliant on advances in software and hardware.
highlight, annotate, and bookmark
You can automatically highlight by performing the text selection while keeping the alt/ key pressed.
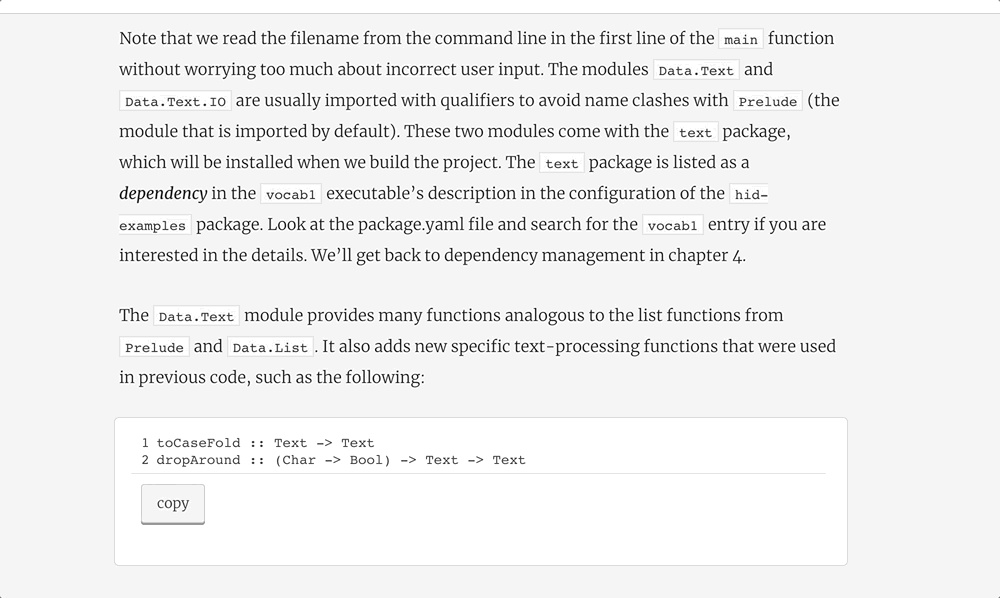
1.4 Learning rules and representations from data
To define deep learning and understand the difference between deep learning and other machine-learning approaches, first we need some idea of what machine learning algorithms do. We just stated that machine learning discovers rules to execute a data-processing task, given examples of what’s expected. So, to do machine learning, we need three things:
- Input data points – For instance, if the task is speech recognition, these data points could be sound files of people speaking. If the task is image tagging, they could be pictures.
- Examples of the expected output – In a speech-recognition task, these could be human-generated transcripts of sound files. In an image task, expected outputs could be tags such as ''dog,'' ''cat,'' and so on.
- A way to measure whether the algorithm is doing a good job – This is necessary in order to determine the distance between the algorithm’s current output and its expected output. The measurement is used as a feedback signal to adjust the way the algorithm works. This adjustment step is what we call learning.
A machine-learning model transforms its input data into meaningful outputs, a process that is ''learned'' from exposure to known examples of inputs and outputs. Therefore, the central problem in machine learning and deep learning is to meaningfully transform data: in other words, to learn useful representations of the input data at hand – representations that get us closer to the expected output.
Before we go any further: what’s a representation? At its core, it’s a different way to look at data – to represent or encode data. For instance, a color image can be encoded in the RGB format (red-green-blue) or in the HSV format (hue-saturation-value): these are two different representations of the same data. Some tasks that may be difficult with one representation can become easy with another. For example, the task ''select all red pixels in the image'' is simpler in the RGB format, whereas ''make the image less saturated'' is simpler in the HSV format. Machine-learning models are all about finding appropriate representations for their input data – transformations of the data that make it more amenable to the task at hand.
Let’s make this concrete. Consider an x-axis, a y-axis, and some points represented by their coordinates in the (x, y) system, as shown in figure 1.3.
Figure 1.3 Some sample data HTML: class=''extra-small-image''
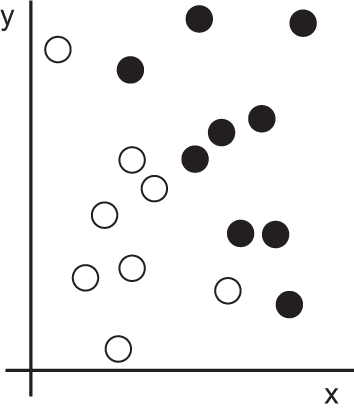
As you can see, we have a few white points and a few black points. Let’s say we want to develop an algorithm that can take a point’s (x, y) coordinates and output whether that point is likely black or white. In this case,
- The inputs are the coordinates of our points.
- The expected outputs are the colors of our points.
- A way to measure whether our algorithm is doing a good job could be, for instance, the percentage of points that are being correctly classified.
What we need here is a new representation of our data that cleanly separates the white points from the black points. One transformation we could use, among many other possibilities, would be a coordinate change, illustrated in figure 1.4.
Figure 1.4 Coordinate change HTML: class=''large-image''
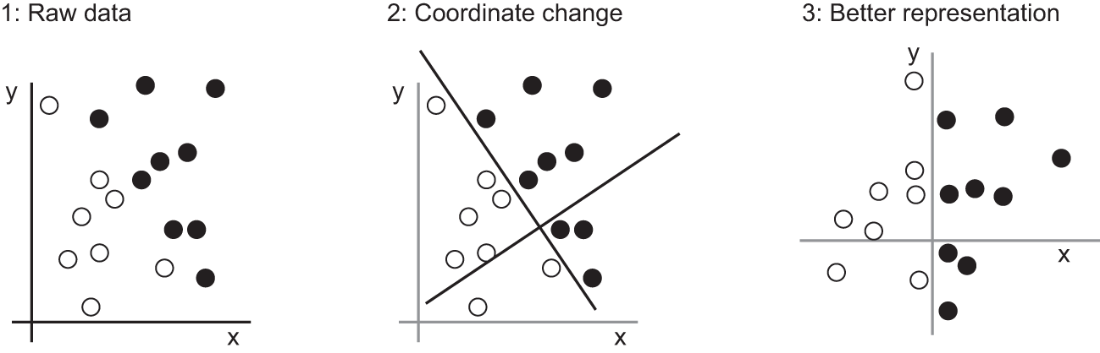
In this new coordinate system, the coordinates of our points can be said to be a new representation of our data. And it’s a good one! With this representation, the black/white classification problem can be expressed as a simple rule: ''Black points are such that x > 0,'' or ''White points are such that x < 0.'' This new representation, combined with this simple rule, neatly solves the classification problem.
In this case, we defined the coordinate change by hand: we used our human intelligence to come up with our own appropriate representation of the data. This is fine for such an extremely simple problem, but could you do the same if the task were to classify images of handwritten digits? Could you write down explicit, computer-executable image transformations that would illuminate the difference between a 6 and a 8, between a 1 and a 7, across all kinds of different handwritings?
This is possible to an extent. Rules based on representations of digits such as ''number of closed loops'', or vertical and horizontal pixel histograms can do a decent job at telling apart handwritten digits. But finding such useful representations by hand is hard work, and as you can imagine the resulting rule-based system would be brittle – a nightmare to maintain. Every time you would come across a new example of handwriting that would break your carefully thought-out rules, you would have to add new data transformations and new rules, while taking into account their interaction with every previous rule.
You’re probably thinking, if this process is so painful, could we automate it? What if we tried systematically searching for different sets of automatically-generated representations of the data and rules based on them, identifying good ones by using as feedback the percentage of digits being correctly classified in some development dataset? We would then be doing machine learning. Learning, in the context of machine learning, describes an automatic search process for data transformations that produce useful representations of some data, guided by some feedback signal – representations that are amenable to simpler rules solving the task at hand.
These transformations can be coordinate changes (like in our 2D coordinates classification example), or taking a histogram of pixels and counting loops (like in our digits classification example), but they could also be linear projections, translations, nonlinear operations (such as ''select all points such that x > 0''), and so on. Machine-learning algorithms aren’t usually creative in finding these transformations; they’re merely searching through a predefined set of operations, called a hypothesis space. For instance, the space of all possible coordinate changes would be our hypothesis space in the 2D coordinates classification example.
So that’s what machine learning is, concisely: searching for useful representations and rules over some input data, within a predefined space of possibilities, using guidance from a feedback signal. This simple idea allows for solving a remarkably broad range of intellectual tasks, from autonomous driving to natural-language question-answering.
Now that you understand what we mean by learning, let’s take a look at what makes deep learning special.
discuss
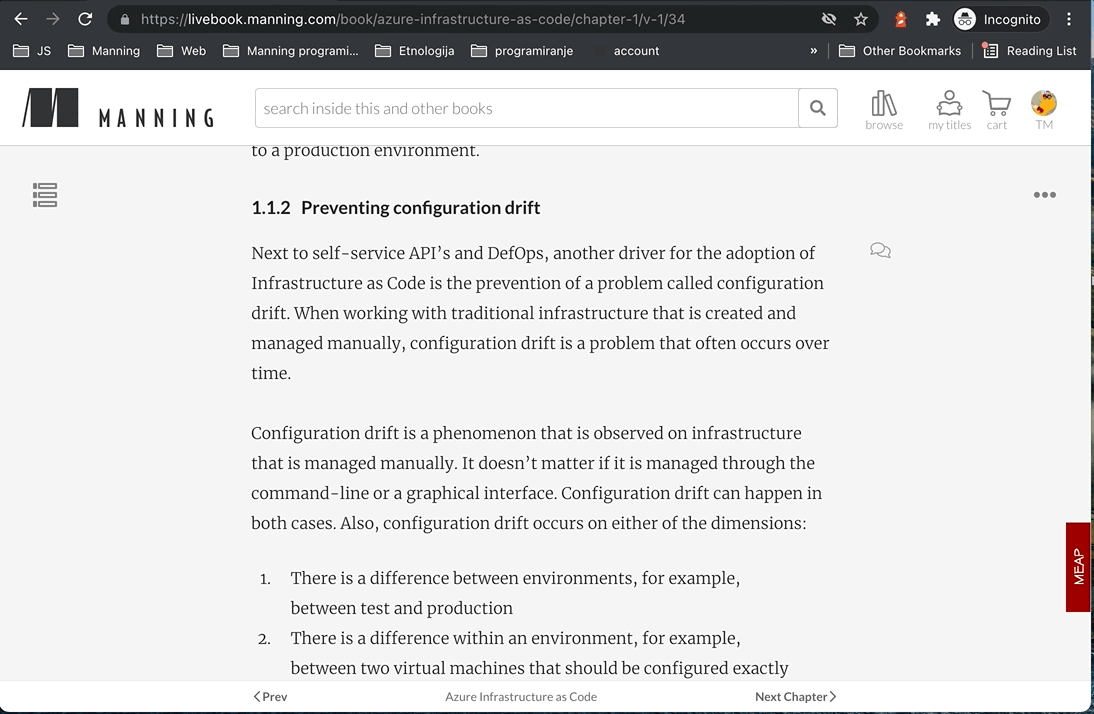
1.5 The ''deep'' in ''deep learning''
Deep learning is a specific subfield of machine learning: a new take on learning representations from data that emphasizes learning successive layers of increasingly meaningful representations. The ''deep'' in ''deep learning'' isn’t a reference to any kind of deeper understanding achieved by the approach; rather, it stands for this idea of successive layers of representations. How many layers contribute to a model of the data is called the depth of the model. Other appropriate names for the field could have been layered representations learning or hierarchical representations learning. Modern deep learning often involves tens or even hundreds of successive layers of representations – and they’re all learned automatically from exposure to training data. Meanwhile, other approaches to machine learning tend to focus on learning only one or two layers of representations of the data (say, taking a pixel histogram and then applying a classification rule); hence, they’re sometimes called shallow learning.
In deep learning, these layered representations are learned via models called neural networks, structured in literal layers stacked on top of each other. The term neural network is a reference to neurobiology, but although some of the central concepts in deep learning were developed in part by drawing inspiration from our understanding of the brain (in particular the visual cortex), deep-learning models are not models of the brain. There’s no evidence that the brain implements anything like the learning mechanisms used in modern deep-learning models. You may come across pop science articles proclaiming that deep learning works like the brain or is modeled after the brain, but that isn’t the case. It would be confusing and counterproductive for newcomers to the field to think of deep learning as being in any way related to neurobiology; you don’t need that shroud of ''just like our minds'' mystique and mystery, and you may as well forget anything you may have read about hypothetical links between deep learning and biology. For our purposes, deep learning is a mathematical framework for learning representations from data.
What do the representations learned by a deep-learning algorithm look like? Let’s examine how a network several layers deep (see figure 1.5) transforms an image of a digit in order to recognize what digit it is.
Figure 1.5 A deep neural network for digit classification
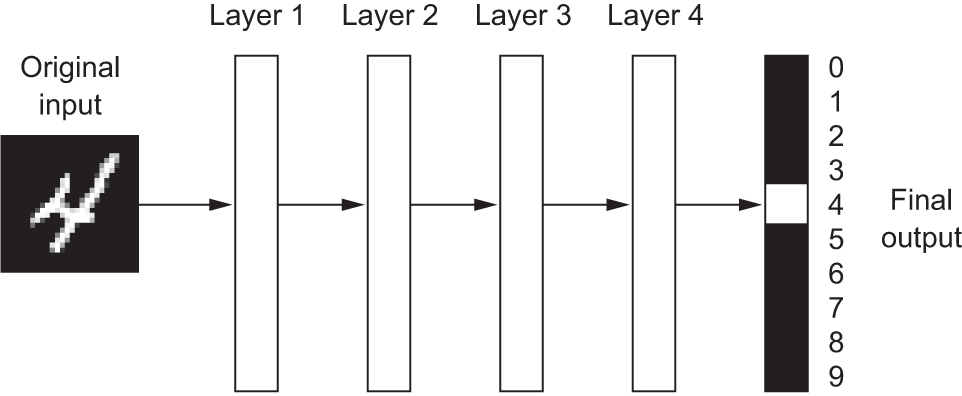
As you can see in figure 1.6, the network transforms the digit image into representations that are increasingly different from the original image and increasingly informative about the final result. You can think of a deep network as a multistage information-distillation process, where information goes through successive filters and comes out increasingly purified (that is, useful with regard to some task).
Figure 1.6 Deep representations learned by a digit-classification model HTML: class=''large-image''
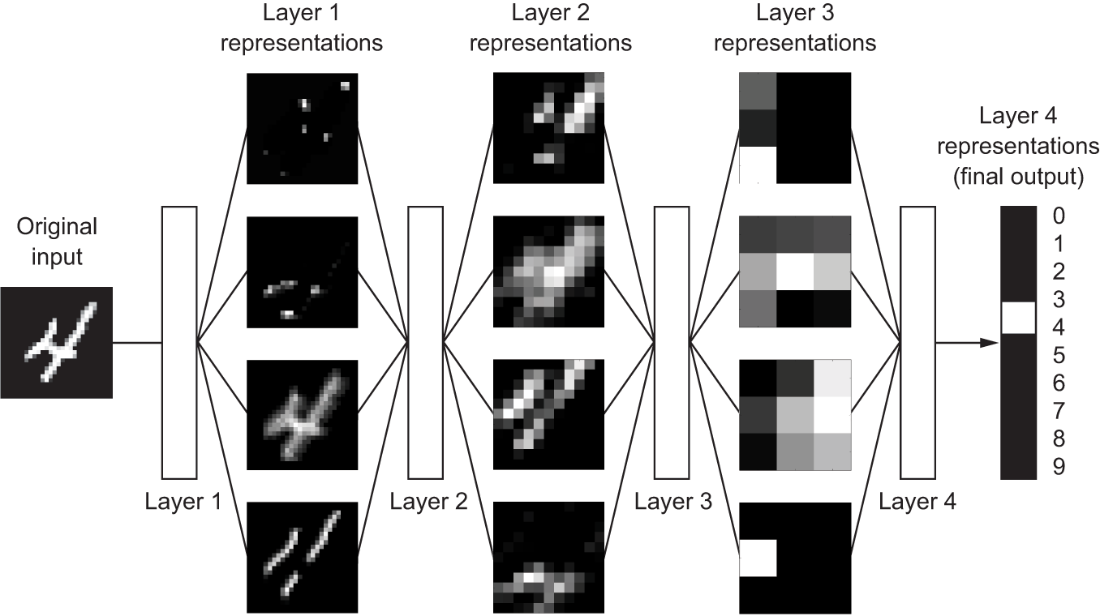
So that’s what deep learning is, technically: a multistage way to learn data representations. It’s a simple idea – but, as it turns out, very simple mechanisms, sufficiently scaled, can end up looking like magic.
settings
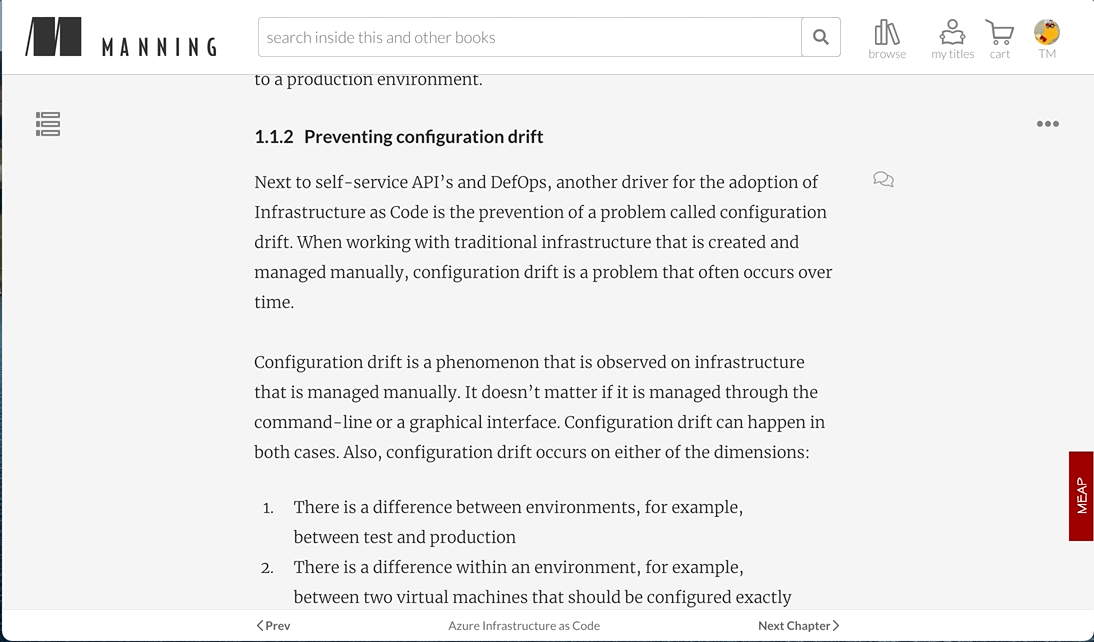
Take our tour and find out more about liveBook's features:
- Search - full text search of all our books
- Discussions - ask questions and interact with other readers in the discussion forum.
- Highlight, annotate, or bookmark.
1.6 Understanding how deep learning works, in three figures
At this point, you know that machine learning is about mapping inputs (such as images) to targets (such as the label ''cat''), which is done by observing many examples of input and targets. You also know that deep neural networks do this input-to-target mapping via a deep sequence of simple data transformations (layers) and that these data transformations are learned by exposure to examples. Now let’s look at how this learning happens, concretely.
The specification of what a layer does to its input data is stored in the layer’s weights, which in essence are a bunch of numbers. In technical terms, we’d say that the transformation implemented by a layer is parameterized by its weights (see figure 1.7). (Weights are also sometimes called the parameters of a layer.) In this context, learning means finding a set of values for the weights of all layers in a network, such that the network will correctly map example inputs to their associated targets. But here’s the thing: a deep neural network can contain tens of millions of parameters. Finding the correct value for all of them may seem like a daunting task, especially given that modifying the value of one parameter will affect the behavior of all the others!
Figure 1.7 A neural network is parameterized by its weights.
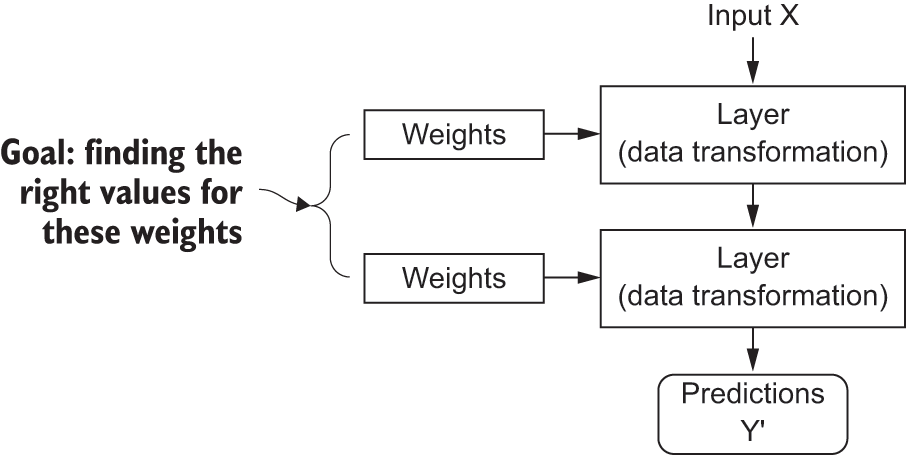
To control something, first you need to be able to observe it. To control the output of a neural network, you need to be able to measure how far this output is from what you expected. This is the job of the loss function of the network, also sometimes called the objective function or cost function. The loss function takes the predictions of the network and the true target (what you wanted the network to output) and computes a distance score, capturing how well the network has done on this specific example (see figure 1.8).
Figure 1.8 A loss function measures the quality of the network’s output. HTML: class=''small-image''
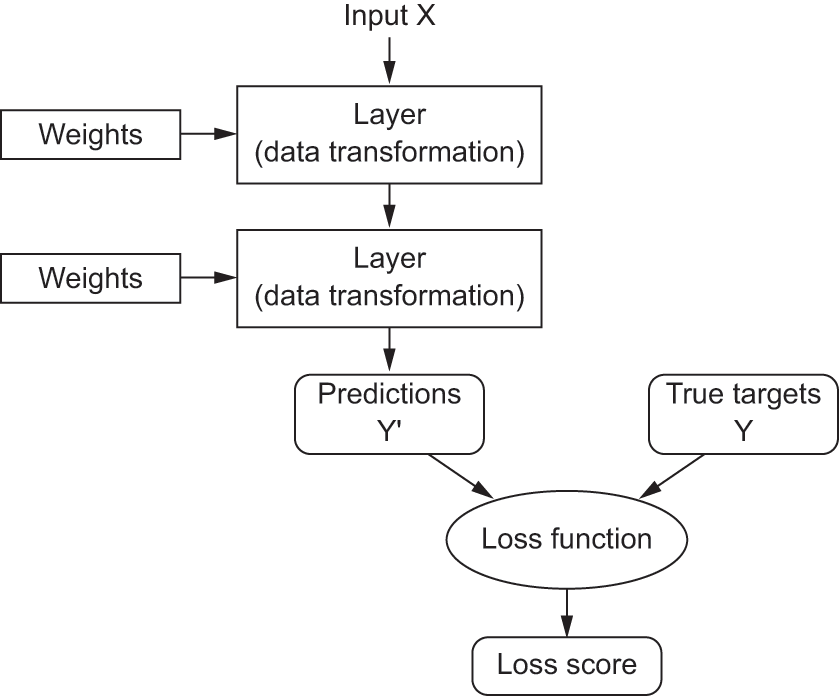
The fundamental trick in deep learning is to use this score as a feedback signal to adjust the value of the weights a little, in a direction that will lower the loss score for the current example (see figure 1.9). This adjustment is the job of the optimizer, which implements what’s called the Backpropagation algorithm: the central algorithm in deep learning. The next chapter explains in more detail how backpropagation works.
Figure 1.9 The loss score is used as a feedback signal to adjust the weights. HTML: class=''small-image''
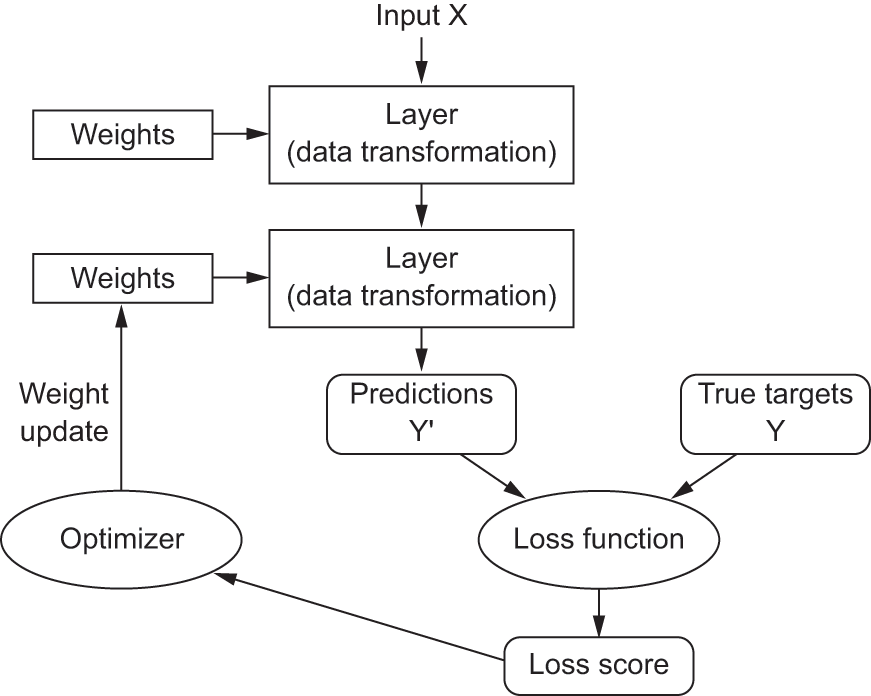
Initially, the weights of the network are assigned random values, so the network merely implements a series of random transformations. Naturally, its output is far from what it should ideally be, and the loss score is accordingly very high. But with every example the network processes, the weights are adjusted a little in the correct direction, and the loss score decreases. This is the training loop, which, repeated a sufficient number of times (typically tens of iterations over thousands of examples), yields weight values that minimize the loss function. A network with a minimal loss is one for which the outputs are as close as they can be to the targets: a trained network. Once again, it’s a simple mechanism that, once scaled, ends up looking like magic.
highlight, annotate, and bookmark
You can automatically highlight by performing the text selection while keeping the alt/ key pressed.
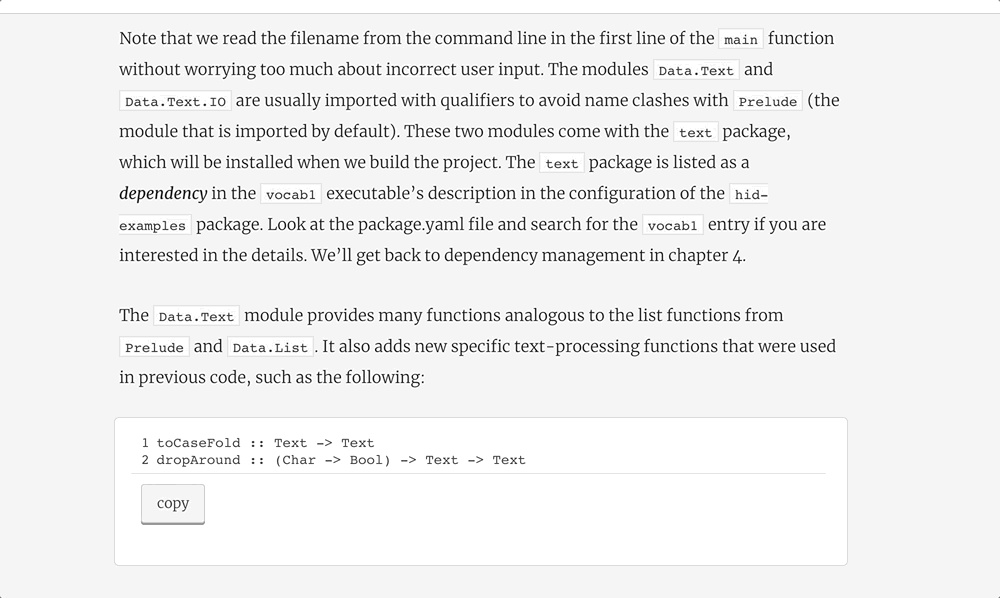
1.7 What makes deep learning different
Is there anything special about deep neural networks that makes them the ''right'' approach for companies be invest in and for researchers to flock to? Will we still be using deep neural networks in 20 years?
Deep learning has several properties that justify its status as an AI revolution, and it’s here to stay. We may not be using neural networks many decades from now, but whatever we use will directly inherit from modern deep learning and its core concepts. These important properties can be broadly sorted into three categories:
- Simplicity – Deep learning makes problem-solving much easier, because it automates what used to be the most crucial step in a machine-learning workflow: feature engineering. Previous machine-learning techniques – shallow learning – only involved transforming the input data into one or two successive representation spaces, which wasn’t expressive enough for most problems. As such, humans had to go to great lengths to make the initial input data more amenable to processing by these methods: they had to manually engineer good layers of representations for their data. This is called feature engineering. Deep learning, on the other hand, completely automates this step: with deep learning, you learn all features in one pass rather than having to engineer them yourself. This has greatly simplified machine-learning workflows, often replacing sophisticated multistage pipelines with a single, simple, end-to-end deep-learning model.
- Scalability – Deep learning is highly amenable to parallelization on GPUs or TPUs, so it can take full advantage of Moore’s law. In addition, deep-learning models are trained by iterating over small batches of data, allowing them to be trained on datasets of arbitrary size. (The only bottleneck is the amount of parallel computational power available, which, thanks to Moore’s law, is a fast-moving barrier.)
- Versatility and reusability – Unlike many prior machine-learning approaches, deep-learning models can be trained on additional data without restarting from scratch, making them viable for continuous online learning – an important property for very large production models. Furthermore, trained deep-learning models are repurposable and thus reusable: this is the big idea behind ''foundation models'' – large models trained on humongous amounts of data, that can be leveraged across many new tasks with little retraining – or even none at all.
discuss
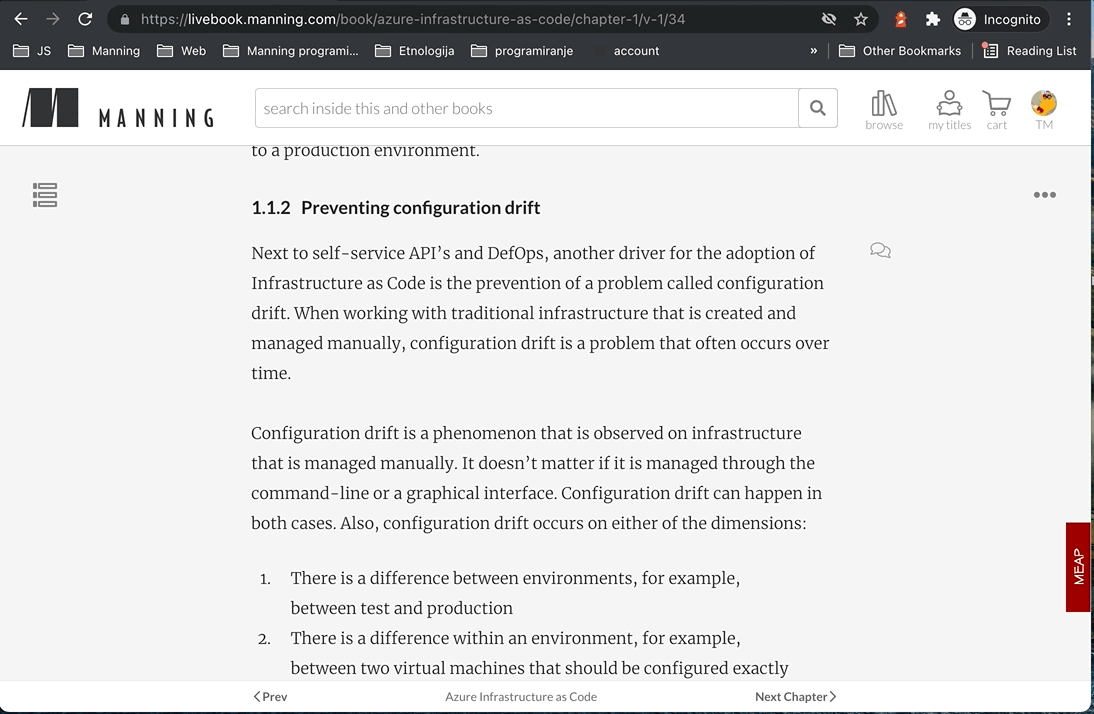
1.8 The age of generative AI
Perhaps the most prominent example of the power of foundation models is the recent wave of generative AI applications – chatbot assistants like ChatGPT, Gemini, and Claude, as well as image generation services like Midjourney. These applications have captured the public imagination with their ability to produce informative or even creative content in response to simple prompts, blurring the lines between human and machine creativity.
Generative AI is powered by very large ''foundation models'' that learn to reconstruct the text and image content fed into them – reconstruct a sharp image from a noisy version, predict the next word in a sentence, and so on. This means that the targets from figure 1.8 are taken from the input itself. This is referred to as self-supervised learning, and it enables those models to leverage vast amounts of unlabeled data. Doing away with the manual data annotations that bottlenecked previous brands of machine learning has unlocked a level of scale never seen before – some of these foundation models have hundreds of billions of parameters and are trained on over one petabyte of data, at the cost of tens of millions of dollars.
These foundation models operate as a kind of fuzzy database of human knowledge, making them amenable to a very wide range of applications without needed special-purpose programming or retraining. Because they’ve already memorized so much, they can solve new problems merely via prompting – querying the knowledge representations they’ve learned and returning the output most likely to be associated with your prompt.
Generative AI only rose to mainstream awareness in 2022, but it has a long history – the earliest experiments with text generation date back to the 1990s. The first edition of this book, released in 2017, already had a hefty chapter titled ''Generative AI'' that explored the text generation and image generation techniques of the time, while promising the then-outlandish notion that, ''soon'', much of the cultural content we consume would be created with the help of AI.
settings
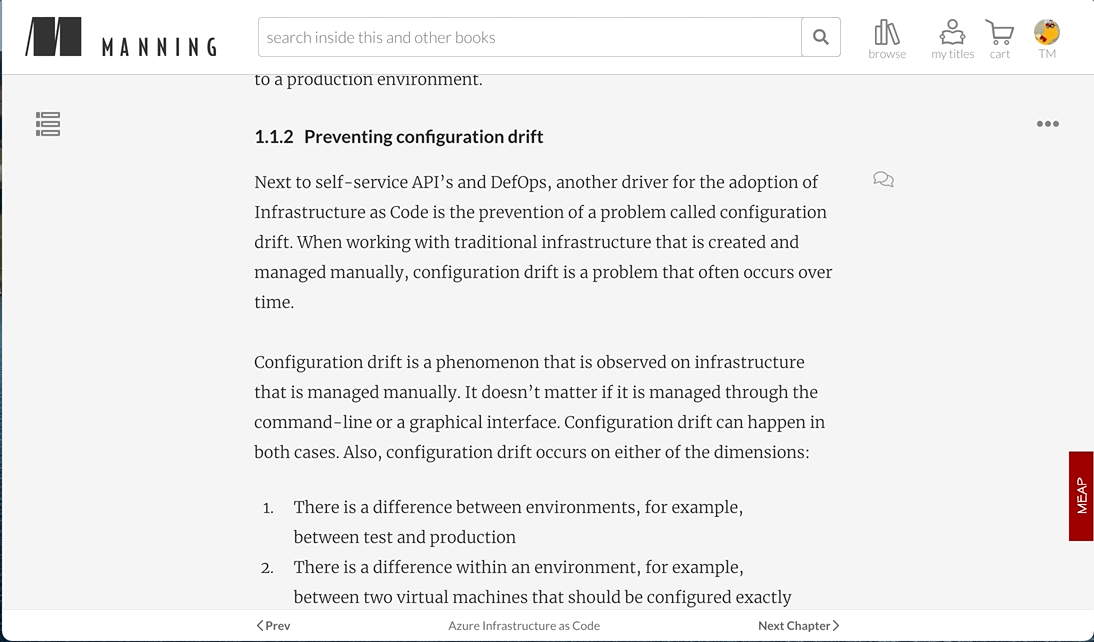
1.9 What deep learning has achieved so far
Over the past decade, deep learning has achieved nothing short of a technological revolution, starting with remarkable results on perceptual tasks from 2013 to 2017, then moving on fast progress on natural language processing tasks from 2017 to 2022, and culminating with a wave of transformative generative AI applications from 2022 to now.
Deep learning has enabled major breakthroughs, all in extremely challenging problems that had long eluded machines:
- Fluent and highly versatile chatbots such as ChatGPT and Gemini
- Programming assistants like GitHub Copilot
- Photorealistic image generation
- Human-level image classification
- Human-level speech transcription
- Human-level handwriting transcription & printed text transcription
- Dramatically improved machine translation
- Dramatically improved text-to-speech conversion
- Human-level autonomous driving, already deployed to the public in Phoenix and San Francisco as of 2024
- Improved recommender systems, as used by YouTube, Netflix, or Spotify
- Superhuman Go playing, Chess playing, Poker playing
We’re still exploring the full extent of what deep learning can do. We’ve started applying it with great success to a wide variety of problems that were thought to be impossible to solve just a few years ago – automatically transcribing the tens of thousands of ancient manuscripts held in the Vatican’s Secret Archive, detecting and classifying plant diseases in fields using a simple smartphone, assisting oncologists or radiologists with interpreting medical imaging data, predicting natural disasters such as floods, hurricanes or even earthquakes… With every milestone, we’re getting closer to an age where deep learning assists us in every activity and every field of human endeavor – science, medicine, manufacturing, energy, transportation, software development, agriculture, and even artistic creation.
highlight, annotate, and bookmark
You can automatically highlight by performing the text selection while keeping the alt/ key pressed.
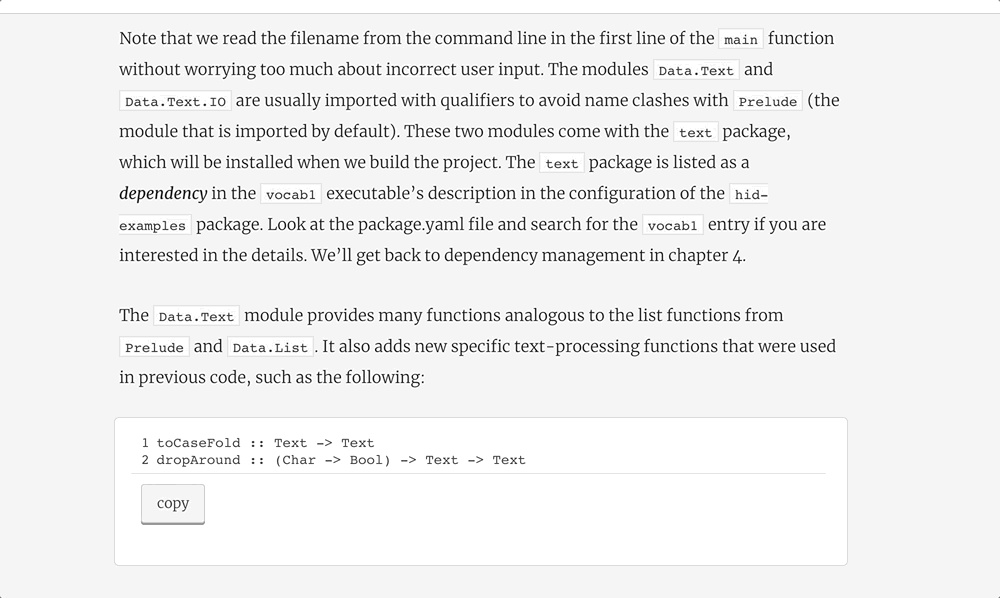
1.10 Beware of the short-term hype
This seemingly unstoppable string of successes has led to a wave of intense hype, some of which is somewhat grounded, but most of which is just fancy fairy tales.
In early 2023, soon after the release of GPT-4 by OpenAI, many pundits were claiming that ''no one needed to work anymore'' and that mass unemployment would be coming within a year, or that economic productivity would soon shoot up by 10x to 100x. Of course, two years later, none of this has come to pass – the unemployment rate in the US is lower now than it was then, while productivity metrics are flat. Don’t get me wrong: the impact of AI – in particular generative AI – is already considerable, and it is growing remarkably fast. Generative AI is generating a few billions of dollars in revenue per year – which is extremely impressive for an industry that did not exist two years ago! But it doesn’t yet make much of a dent in the overall economy, and pales in comparison to the absolutely unbridled promises we were inundated with at its onset.
While discussions about unemployment and 100x productivity gains triggered by AI are already stirring anxieties, there’s an even more sensational side to the AI hype. This side proclaims the imminent arrival of human-level general intelligence (AGI), or even ''superintelligence'' far surpassing human capabilities. These claims are fueling fears beyond economic disruption – the human species itself might be in danger of being replaced by our digital creations.
It might be tempting for those new to the field to assume that it is the practical successes of generative AI that caused the belief in near-term AGI, but that is actually backwards. The claims of near-term AGI came first, and they majorly contributed to the rise of generative AI. As early as 2013, there were fears among tech elites that AGI might be coming within a few years – back then, the idea was that DeepMind, a London AI research startup acquired by Google in 2013, was on track to achieve it. This belief was the impetus behind the founding of OpenAI in 2015, which initially aimed to be an open-source counter-weight to DeepMind. OpenAI played a critical role in kickstarting generative AI, so in a peculiar twist, it was the belief in near-term AGI that fueled the ascent of generative AI, not the other way around. In 2016, OpenAI’s recruiting pitch was that it would achieve AGI by 2020! Although, to be fair, only a minority of people in the tech industry believed in such an optimistic timeline back then. By early 2023, however, a significant fraction of engineers in the San Francisco Bay Area seemed convinced that AGI would be coming within the following couple of years.
It’s crucial to approach such claims with a healthy dose of skepticism. Despite its name, today’s ''artificial intelligence'' is more accurately described as ''cognitive automation'' – the encoding and operationalization of human skills and knowledge. AI excels at solving problems with narrowly defined requirements or those where ample precise examples are available. It’s about enhancing the capabilities of computers, not about replicating human minds.
To be clear, cognitive automation is incredibly useful. But intelligence – cognitive autonomy – is a different creature altogether. Think of it this way: AI is like a cartoon character, while intelligence is like a living being. A cartoon, no matter how realistic, can only act out the scenes it was drawn for. A living being, on the other hand, can adapt to the unexpected.
''If the cartoon is drawn with sufficient realism and covers sufficiently many scenes, what’s the difference?'', you may ask. If a large language model can output a sufficiently human-sounding answer when asked a question, does it matter if it possesses true cognitive autonomy? The key difference is adaptability. Intelligence is the ability to face the unknown, adapt to it, and learn from it. Automation, even at its best, can only handle situations it’s been trained on, or programmed for. That’s why creating robust automation is so challenging - it requires accounting for every possible scenario.
So, don’t worry about AI suddenly becoming self-aware and taking over humanity. Today’s technology simply isn’t headed in that direction. Even with significant advancements, AI will remain a sophisticated tool, not a sentient being. It’s like expecting a better clock to lead to time travel - they’re just different things altogether.
discuss
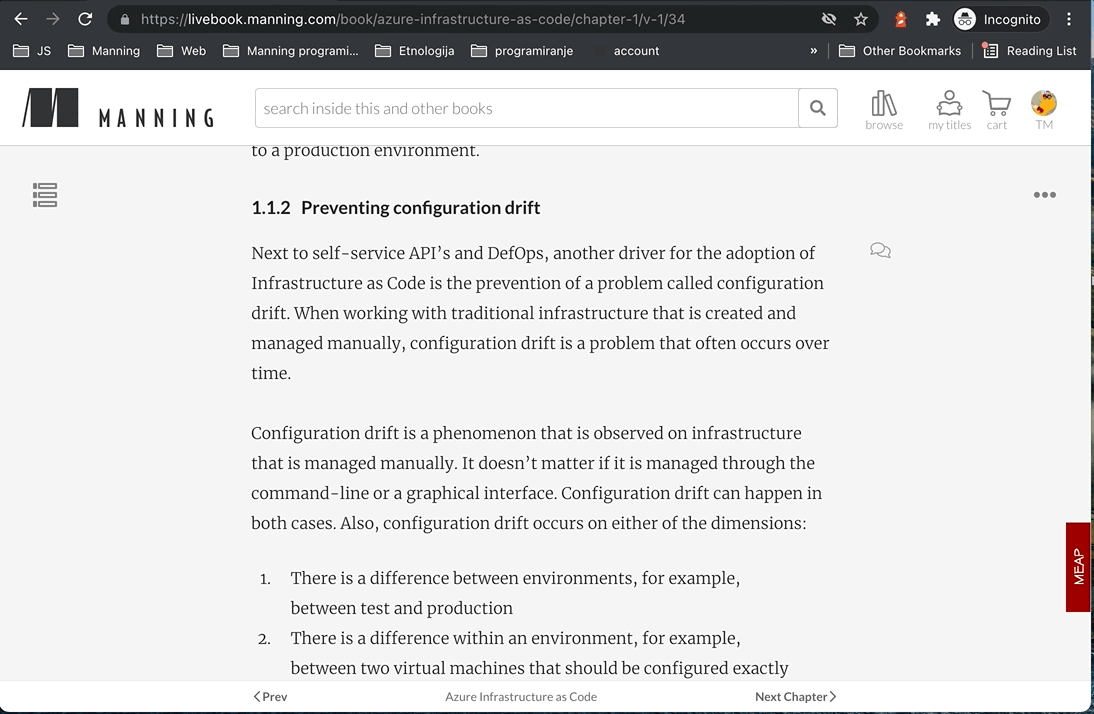
Take our tour and find out more about liveBook's features:
- Search - full text search of all our books
- Discussions - ask questions and interact with other readers in the discussion forum.
- Highlight, annotate, or bookmark.
1.11 Summer can turn to winter
The danger of inflated short-term expectations is that when technology inevitably falls short, research investment could dry up, slowing progress for a long time.
This has happened before. Twice in the past, AI went through a cycle of intense optimism followed by disappointment and skepticism, with a dearth of funding as a result. It started with symbolic AI in the 1960s. In those early days, projections about AI were flying high. One of the best-known pioneers and proponents of the symbolic AI approach was Marvin Minsky, who claimed in 1967, ''Within a generation … the problem of creating `artificial intelligence' will substantially be solved.'' Three years later, in 1970, he made a more precisely quantified prediction: ''In from three to eight years we will have a machine with the general intelligence of an average human being.'' In 2020, such an achievement still appears to be far in the future – so far that we have no way to predict how long it will take – but in the 1960s and early 1970s, several experts believed it to be right around the corner (as do many people today). A few years later, as these high expectations failed to materialize, researchers and government funds turned away from the field, marking the start of the first AI winter (a reference to a nuclear winter, because this was shortly after the height of the Cold War).
It wouldn’t be the last one. In the 1980s, a new take on symbolic AI, expert systems, started gathering steam among large companies. A few initial success stories triggered a wave of investment, with corporations around the world starting their own in-house AI departments to develop expert systems. Around 1985, companies were spending over $1 billion each year on the technology; but by the early 1990s, these systems had proven expensive to maintain, difficult to scale, and limited in scope, and interest died down. Thus began the second AI winter. We may be currently witnessing the third cycle of AI hype and disappointment – and we’re still in the phase of intense optimism.
My current view is that we’re unlikely to see a full-scale retreat away from AI research like we saw in the 1990s. If there is a winter, it should be mild. However, it seems inevitable that some air will need to be let out of the 2023-204 AI bubble. Currently, AI investment, primarily in data centers and GPUs, surpasses $100 billion annually, while revenue generation lags significantly, closer to $10 billion. AI is currently being judged by executives and investors not by what it has accomplished, but what we are told it might soon become able to do – much of which will durably stay out of reach of existing technologies. Something will have to give. But what will happen precisely as the AI bubble deflates is still up in the air.
settings
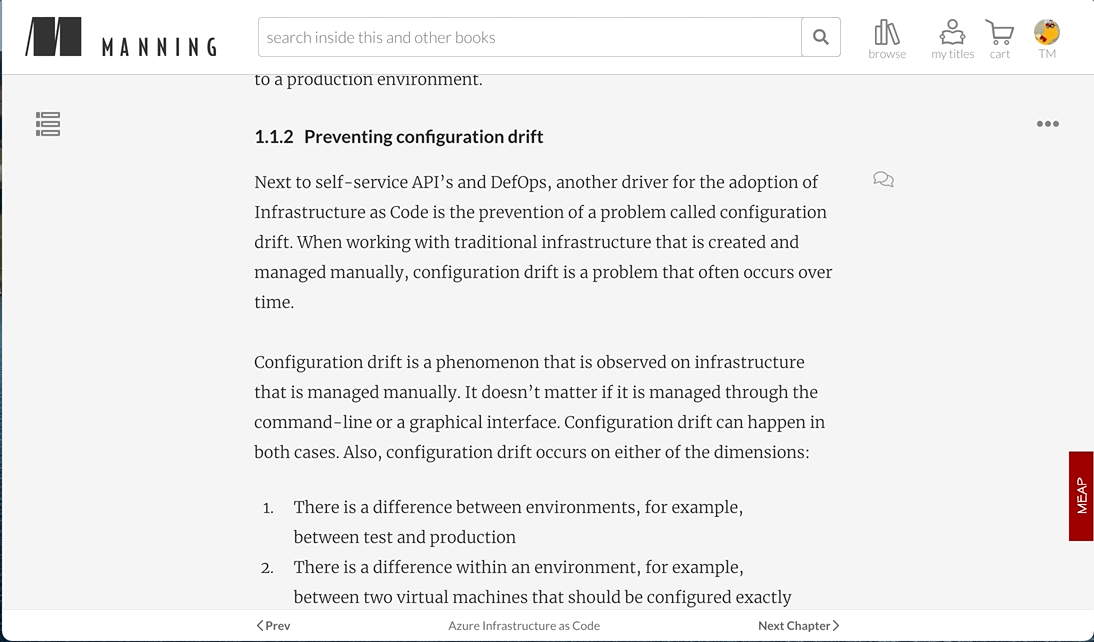
1.12 The promise of AI
Although we may have unrealistic short-term expectations for AI, the long-term picture is looking bright. We’re only getting started in applying deep learning to many important problems for which it could prove transformative, from medical diagnoses to digital assistants.
In 2017, in this very book, I wrote:
Right now, it may seem hard to believe that AI could have a large impact on our world, because it isn’t yet widely deployed – much as, back in 1995, it would have been difficult to believe in the future impact of the internet. Back then, most people didn’t see how the internet was relevant to them and how it was going to change their lives. The same is true for deep learning and AI today. But make no mistake: AI is coming. In a not-so-distant future, AI will be your assistant, even your friend; it will answer your questions, help educate your kids, and watch over your health. It will deliver your groceries to your door and drive you from point A to point B. It will be your interface to an increasingly complex and information-intensive world. And, even more important, AI will help humanity as a whole move forward, by assisting human scientists in new breakthrough discoveries across all scientific fields, from genomics to mathematics.
Fast-forward to 2024, most of these things have come either come true or are on the verge of coming true – and this is just the beginning.
- Tens of millions of people are using AI chatbots like ChapGPT, Gemini, or Claude as assistants on a daily basis. In fact, question-answering and ''educating your kids'' (homework assistance) have turned out to be the top applications of these chatbots! AI is already their go-to interface to the world’s information.
- Tens of thousands of people interact with AI ''friends'' in applications such as Character.ai
- Fully autonomous driving is already deployed at scale in two cities: San Francisco and Phoenix. And those autonomous Waymo cars are powered by Keras models!
- AI is making major strides towards helping accelerate science. The AlphaFold model from DeepMind is helping biologists predict protein structures with unprecedented accuracy. Renowned mathematician Terence Tao believes that by around 2026, AI could become a reliable co-author in mathematical research and other fields when used appropriately.
The AI revolution, once a distant vision, is now rapidly unfolding before our eyes. On the way, we may face a few setbacks and maybe even a new AI winter – in much the same way the internet industry was overhyped in 1998–1999 and suffered from a crash that dried up investment throughout the early 2000s. But we’ll get there eventually. AI will end up being applied to nearly every process that makes up our society and our daily lives, much like the internet is today.
Don’t believe the short-term hype, but do believe in the long-term vision. It may take a while for AI to be deployed to its true potential – a potential the full extent of which no one has yet dared to dream – but AI is coming, and it will transform our world in a fantastic way.
[1] A. M. Turing, ''Computing Machinery and Intelligence,'' Mind 59, no. 236 (1950): 433-460.
[2] Although the Turing test has sometimes been interpreted as a literal test – a goal the field of AI should set out to reach – Turing merely meant it as a conceptual device in a philosophical discussion about the nature of cognition.